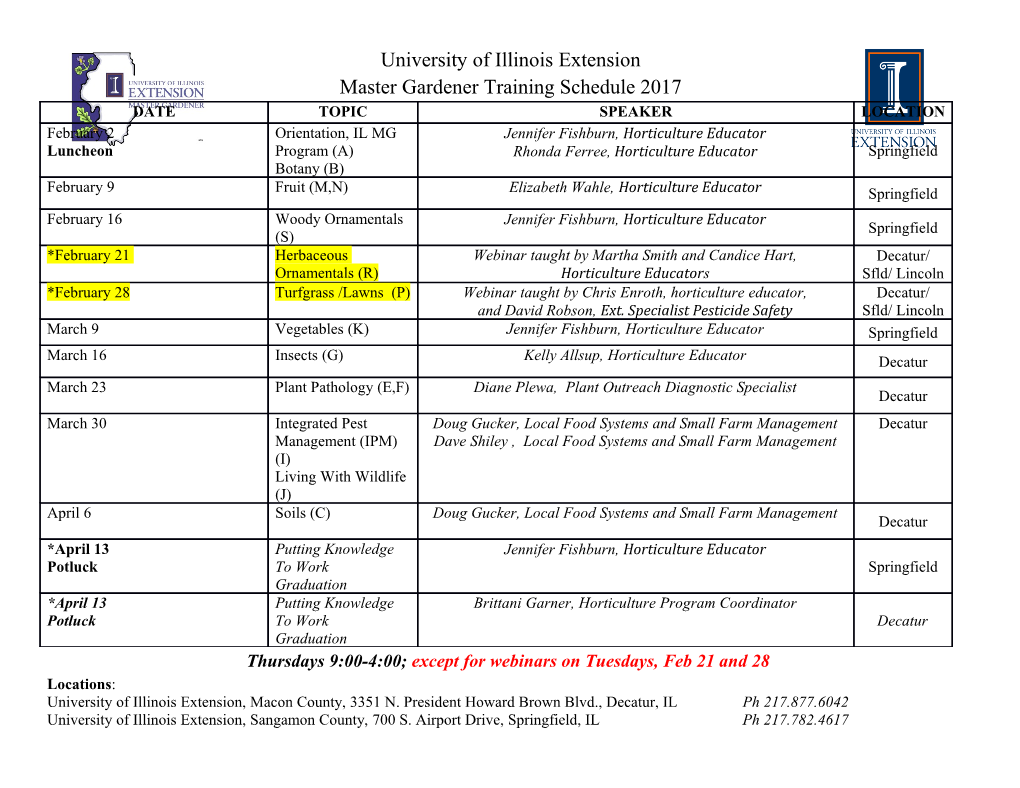
Robust Nonlinear Wavelet Transform based on Median-Interpolation David L. Donoho & Thomas P.Y. Yu Department of Statistics Stanford University Stanford, CA 945305, USA [email protected] [email protected] Abstract distribution; and so some form of homogeneous treatment (i.e. thresholding all coefficients in a standard way) is plau- It is well known that wavelet transforms can be de- sible. n (z ) rived from stationary linear refinement subdivision schemes Now consider (1) in cases when the white noise i i=1 [2, 3]. In this paper we discuss a special nonlinear re- is highly non-Gaussian. An example of such setting arises finement subdivision scheme – median-interpolation. It is a in target tracking in radar [9, 13], in which one encounters nonlinear cousin of Deslauriers-Dubuc interpolation and of data contaminated by impulsive glint noise. As a model, n (z ) average-interpolation. The refinement scheme is based on we consider i to be i.i.d. Cauchy distributed. The i=1 R ` ` = f (x)dx constructing polynomials which interpolate median func- Cauchy distribution has no moments x for ; 2;::: tionals of the underlying object. The refinement scheme can 1 , in particular neither mean nor variance. It therefore be deployed in multiresolution fashion to construct nonlin- offers an extreme example of non-Gaussian data. n z ) ear pyramid schemes and associated forward and inverse ( Under this model, typical noise realizations i con- i=1 transforms. In this paper we discuss the basic properties tain a few astonishingly large observations: the largest (n) of this transform and its possible use in wavelet de-noising observation is of size O . (In comparison, for Gaus- p ( log (n)) schemes for badly non-Gaussian data. Analytic and com- sian noise, the largest observation is of size O .) putational results are presented to show that the nonlinear Moreover, the wavelet transform of i.i.d. Cauchy noise does pyramid has very different performance compared to tradi- not result in independent, nor identically distributed wavelet tional wavelets when coping with non-Gaussian data. coefficients. In fact, coefficients at larger scales are more likely to be affected by the perturbing influence of the few large noise values, and so have a systematically larger dis- persion at large scales. Invariance of distribution under or- 1. Introduction p ( log(n)) thogonal transforms and O behavior of maxima A number of recent theoretical studies [6, 5] have found are fundamental to the results of [5, 6]. This extremely non- that the orthogonal wavelet transform offers a promising ap- Gaussian situation lacks key quantitative properties which proach to noise removal. They assume that one has noisy were used in de-noising in the Gaussian case. In this paper we study a wavelet-like approach to deal samples of an underlying function f with such extremely non-Gaussian data which is based = f (t )+z ;i=1;:::;n; y on abandoning linear orthogonal transforms and using in- i i i (1) stead a kind of nonlinear wavelet transform called Median- n (z ) where i is a Gaussian white noise. In this setting, Interpolating Pyramid Transform (MIPT.) The nonlinear i=1 they show that one removes a noise successfully by apply- transforms we propose combine ideas from the theory of ro- ing a wavelet transform, then applying thresholding in the bust estimation [10, 8] and ideas from wavelets and the the- wavelet domain, then inverting the transform. ory of interpolating subdivisions [1, 7]. The forward trans- A key principle underlying this approach is the fact that form (FMIPT) is based on deploying the median in a mul- an orthogonal transform takes Gaussian white noise into tiresolution pyramid; this gives an analysis method which is Gaussian white noise. It follows from this, and the Gaussian highly robust against extreme observations. The transform assumption on the noise z , that in the transform domain, all itself is based on computation of “block medians” over tri- wavelet coefficients suffer noise with the same probability adic cells, and the recording of block medians in a “r´esum´e- detail” array. The inverse transform (IMIPT) is based on Unlike Lagrange interpolation or average-interpolation, deploying median-interpolation in a multiresolution fash- median-interpolation is a nonlinear procedure and we are ion. At its center is the notion of “median-interpolating re- not aware of any algorithms available in the literature. In finement scheme”, a two-scale refinement scheme based on the following we briefly describe our attempt in solving this imputing behavior at finer scales from coarse-scale infor- problem. =0 mation. D . It is the simplest by far; in that case one is fitting (t) = C onst A = 0 Our method of building transforms from refinement a constant function j;k . Hence , and (t)=m : j;k schemes is similar to the way interpolating wavelet (2) becomes j;k The imputation step (3) then m = m l =0; 1; 2: +1;3k +l j;k transforms are built from Deslauriers-Dubuc interpolating yields j for Hence refinement schemes in [2] and in the way biorthogonal wavelet trans- proceeds by imputing a constant behavior at finer scales. D > 0 I = [i 1;i] x = i 1=2 i = i forms are built from average-interpolating refinement in [3]. Let i , , D +1 D +1 ;:::;(D +1) F : R ! R However, despite structural similarities there are important 1 . Define the map by (F (v )) = med( jI ) i differences: i where is the polynomial specified D +1 2 R by the vector v and the Lagrange interpolation both the forward and inverse transforms can be nonlin- ear; basis, D +1 D +1 X Y x x ) the transforms are based on a triadic pyramid and a 3- ( j (x)= v l (x) l (x)= : i i to-1 decimation scheme; i where (4) (x x ) i j i=1 j =1;j 6=i n the transforms are expansive (they map data into F =2n 3 coefficients). We have the following bisection algorithm to apply to a given vector v . It would be more accurate to call the approach a nonlinear = v tol Algorithm m BlockMedian( , ): pyramid transform. a+b + ) = max( jI ) m = min( jI ) m = ( 1. m , , , 2 v 2. Median-Interpolating Refinement where is formed from as in (4). (x) m In this section, we describe the mechanism of median- 2. Calculate all the roots of , collect the ones a; b) interpolating refinement, which is the major building block that are (i) real, (ii) inside ( and (iii) with odd fr ;:::;r g k of MIPT. multiplicities into the ordered array 1 (i.e. r <r r = a r = b i+1 0 k +1 i ). Let and . I med (f jI ) Given a function f on an interval , let de- P f I note a median of for the interval , for definiteness we + (a) m M = r r M = i1 3. If , i and i odd P P follow John Tukey’s suggestion and use the lowest median, M = r r r r i i1 i1 i ; else and i odd i even P med (f jI ) = inf f : m(t 2 I : f (t) ) defined by + M = r r i1 i . i even (t 2 I : f (t) )g: m Now suppose we are given a tri- j 3 1 + + jM M j < tol M >M + fm g adic array j;k of numbers representing the medi- 4. If , terminate, else if k =0 + + j j tol m = m; m =(m + m )=2 m = f I =[k 3 ; (k +1)3 ) ans of on the triadic intervals j;k : , , goto 2 ; else j m; m =(m + m )=2 m = med(f jI ) 0 k 3 : j;k j;k The goal of median- , goto 2. interpolating refinement is to use the data at scale j to infer To search for a degree D polynomial with prescribed +1 behavior at the finer scale j , obtaining imputed medi- fm g fI g i block median i at , we need to solve the nonlin- f I +1;k ans of on intervals j . Obviously we are missing the information to impute perfectly; nevertheless we can try to ear system do a reasonable job. (v )=m: F (5) We will employ polynomial-imputation. Starting from a fixed even integer D , it involves two steps. There are general nonlinear solvers that can be used to 5 I solve ( ). We recommend below a more tailored method [M1] (Interpolation) For each interval j;k , find a polyno- D = 2A which is based on fixed point iteration. mial j;k of degree satisfying the median- interpolation condition: The main empirical observation is that median- interpolation can be well approximated by Lagrange inter- med( jI )=m A l A: j;k +l j;k +l j;k for (2) j[a; b]) ((a + polation. We first observe that med( )=2) [M2] (Imputation) Obtain (pseudo-) medians at the finer b . The above approximation is exactly, for example, [a; b] [a; b] scale by setting when is monotonic on , which is the case when is away from the vicinity of the extremum points of . The m~ = med( jI ) l =0; 1; 2: +1;3k +l j;k j;3k +l j for (3) linear approximation can be combined with the idea of fixed =2 point iteration to give the following iterative algorithm for In case D , we have no closed-form expression for median-interpolation. the result. The operator R is nonlinear, and proving the ex- ~ f v = (m; tol ) h ! 1 +h Algorithm MedianInterp : istence of a limit j as requires more than a direct application of theory in linear refinement schemes.
Details
-
File Typepdf
-
Upload Time-
-
Content LanguagesEnglish
-
Upload UserAnonymous/Not logged-in
-
File Pages5 Page
-
File Size-