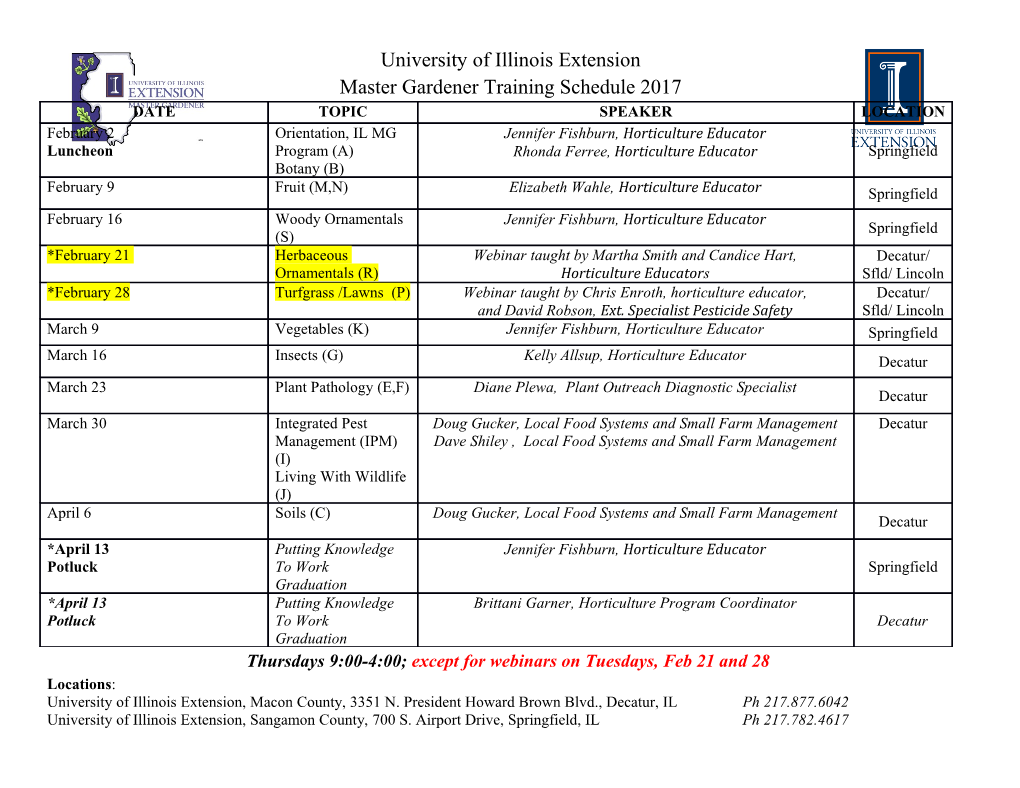
Online Learning for Big Data Analytics Irwin King, Michael R. Lyu and Haiqin Yang Department of Computer Science & Engineering The Chinese University of Hong Kong Tutorial presentation at IEEE Big Data, Santa Clara, CA, 2013 1 Outline • Introduction (60 min.) – Big data and big data analytics (30 min.) – Online learning and its applications (30 min.) • Online Learning Algorithms (60 min.) – Perceptron (10 min.) – Online non-sparse learning (10 min.) – Online sparse learning (20 min.) – Online unsupervised learning (20. min.) • Discussions + Q & A (5 min.) 2 Outline • Introduction (60 min.) – Big data and big data analytics (30 min.) – Online learning and its applications (30 min.) • Online Learning Algorithms (60 min.) – Perceptron (10 min.) – Online non-sparse learning (10 min.) – Online sparse learning (20 min.) – Online unsupervised learning (20. min.) • Discussions + Q & A (5 min.) 3 What is Big Data? • There is not a consensus as to how to define Big Data “A collection of data sets so large and complex that it becomes difficult to process using on-hand database management tools or traditional data processing applications.” - wikii “Big data exceeds the reach of commonly used hardware environments and software tools to capture, manage, and process it with in a tolerable elapsed time for its user population.” - Tera- data magazine article, 2011 “Big data refers to data sets whose size is beyond the ability of typical database software tools to capture, store, manage and analyze.” - The McKinsey Global Institute, 2011i 4 What is Big Data? Activity: Activity: IOPS File/Object Size, Content Volume Big Data refers to datasets grow so large and complex that it is difficult to capture, store, manage, share, analyze and visualize within current computational architecture. 5 Evolution of Big Data • Birth: 1880 US census • Adolescence: Big Science • Modern Era: Big Business 6 Birth: 1880 US census 7 The First Big Data Challenge • 1880 census • 50 million people • Age, gender (sex), occupation, education level, no. of insane people in household 8 The First Big Data Solution • Hollerith Tabulating System • Punched cards – 80 variables • Used for 1890 census • 6 weeks instead of 7+ years 9 Manhattan Project (1946 - 1949) • $2 billion (approx. 26 billion in 2013) • Catalyst for “Big Science” 10 Space Program (1960s) • Began in late 1950s • An active area of big data nowadays 11 Adolescence: Big Science 12 Big Science • The International Geophysical Year – An international scientific project – Last from Jul. 1, 1957 to Dec. 31, 1958 • A synoptic collection of observational data on a global scale • Implications – Big budgets, Big staffs, Big machines, Big laboratories 13 Summary of Big Science • Laid foundation for ambitious projects – International Biological Program – Long Term Ecological Research Network • Ended in 1974 • Many participants viewed it as a failure • Nevertheless, it was a success – Transform the way of processing data – Realize original incentives – Provide a renewed legitimacy for synoptic data collection 14 Lessons from Big Science • Spawn new big data projects – Weather prediction – Physics research (supercollider data analytics) – Astronomy images (planet detection) – Medical research (drug interaction) – … • Businesses latched onto its techniques, methodologies, and objectives 15 Modern Era: Big Business 16 Big Science vs. Big Business • Common – Need technologies to work with data – Use algorithms to mine data • Big Science – Source: experiments and research conducted in controlled environments – Goals: to answer questions, or prove theories • Big Business – Source: transactions in nature and little control – Goals: to discover new opportunities, measure efficiencies, uncover relationships 17 Big Data is Everywhere! • Lots of data is being collected and warehoused – Science experiments – Web data, e-commerce – Purchases at department/ grocery stores – Bank/Credit Card transactions – Social Networks 18 Big Data in Science • CERN - Large Hadron Collider – ~10 PB/year at start – ~1000 PB in ~10 years – 2500 physicists collaborating • Large Synoptic Survey Telescope (NSF, DOE, and private donors) – ~5-10 PB/year at start in 2012 – ~100 PB by 2025 • Pan-STARRS (Haleakala, Hawaii) US Air Force – now: 800 TB/year – soon: 4 PB/year 19 Big Data from Different Sources 4.6 12+ TBs 30 billion RFID tags today billion of tweet data camera (1.3B in 2005) every day phones world wide 100s of millions of of GPS enabled day devices ? TBs data every every data sold annually 25+ TBs of 2+ log data billion every day people on the 76 million smart Web by meters in 2009… end 2011 200M by 2014 20 Big Data in Business Sectors SOURCE: McKinsey Global Institute 21 Characteristics of Big Data • 4V: Volume, Velocity, Variety, Veracity Volume Velocity Variety Veracity Volume Volume VolumeText Images US predictsVolume powerful quake this week New sharing over 2.5 Videos Audios UAE body says it's a 8 billion TB in 2015, billion per day rumour; 40 ZB in 2020 new data over 500TB By Wam/Agencies 5.2TB per person per day Published Saturday, April 20, 2013 22 Big Data Analytics • Definition: a process of inspecting, cleaning, transforming, and modeling big data with the goal of discovering useful information, suggesting conclusions, and supporting decision making • Connection to data mining – Analytics include both data analysis (mining) and communication (guide decision making) – Analytics is not so much concerned with individual analyses or analysis steps, but with the entire methodology 23 Outline • Introduction (60 min.) – Big data and big data analytics (30 min.) – Online learning and its applications (30 min.) • Online Learning Algorithms (60 min.) – Perceptron (10 min.) – Online non-sparse learning (10 min.) – Online sparse learning (20 min.) – Online unsupervised learning (20. min.) • Discussions + Q & A (5 min.) 24 Challenges and Aims • Challenges: capturing, storing, searching, sharing, analyzing and visualizing • Big data is not just about size – Finds insights from complex, noisy, heterogeneous, longitudinal, and voluminous data – It aims to answer questions that were previously unanswered • This tutorial focuses on online learning techniques for Big Data 25 Learning Techniques Overview • Learning paradigms – Supervised learning – Semisupervised learning – Transductive learning – Unsupervised learning – Universum learning – Transfer learning 26 What is Online Learning? • Batch/Offline learning • Online learning – Observe a batch of training – Observe a sequence of data x , y N data i i i1 x1, y1 ,,xt , yt – Learn a model from them – Learn a model incrementally – Predict new samples as instances come accurately – Make the sequence of online predictions accurately Make prediction user True response Update a model 27 Online Prediction Algorithm • An initial prediction rule f0 () • For t=1, 2, … – We observe x t and make a prediction ft1(xt ) – We observe the true outcome yt and then compute a loss l( f (xt ), yt ) – The online algorithm updates the prediction rule using the new example and construct f t (x) ft1(xt ) xt user y x t f Update t 28 Online Prediction Algorithm • The total error of the method is T l( ft1(xt ), yt ) t1 • Goal: this error to be as small as possible • Predict unknown future one step a time: similar to generalization error ft1(xt ) x user t yt 29 Regret Analysis • f * ( ) : optimal prediction function from a class H, e.g., the class of linear classifiers T f* arg min l( f (xt ), yt ) f H t1 with minimum error after seeing all examples • Regret for the online learning algorithm 1 T regret l( f (x ), y ) l( f (x ), y ) T t1 t t * t t t1 We want regret as small as possible 30 Why Low Regret? • Regret for the online learning algorithm 1 T regret l( f (x ), y ) l( f (x ), y ) T t1 t t * t t t1 • Advantages – We do not lose much from not knowing future events – We can perform almost as well as someone who observes the entire sequence and picks the best prediction strategy in hindsight – We can also compete with changing environment 31 Advantages of Online Learning • Meet many applications for data arriving sequentially while predictions are required on-the-fly – Avoid re-training when adding new data • Applicable in adversarial and competitive environment • Strong adaptability to changing environment • High efficiency and excellent scalability • Simple to understand and easy to implement • Easy to be parallelized • Theoretical guarantees 32 Where to Apply Online Learning? Social Media Online Learning Internet Finance Security 33 Online Learning for Social Media • Recommendation, sentiment/emotion analysis 34 Where to Apply Online Learning? Social Media Online Learning Internet Finance Security 35 Online Learning for Internet Security • Electronic business sectors – Spam email filtering – Fraud credit card transaction detection – Network intrusion detection system, etc. 36 Where to Apply Online Learning? Social Media Online Learning Internet Finance Security 37 Online Learning for Financial Decision • Financial decision – Online portfolio selection – Sequential investment, etc. 38 Outline • Introduction (60 min.) – Big data and big data analytics (30 min.) – Online learning and its applications (30 min.) • Online Learning Algorithms (60 min.) – Perceptron (10 min.) – Online non-sparse learning (10 min.) – Online sparse learning (20 min.) – Online unsupervised learning (20. min.) • Discussions + Q & A (5 min.) 39 Outline • Introduction (60 min.) – Big data and big data analytics (30 min.) – Online learning and
Details
-
File Typepdf
-
Upload Time-
-
Content LanguagesEnglish
-
Upload UserAnonymous/Not logged-in
-
File Pages116 Page
-
File Size-