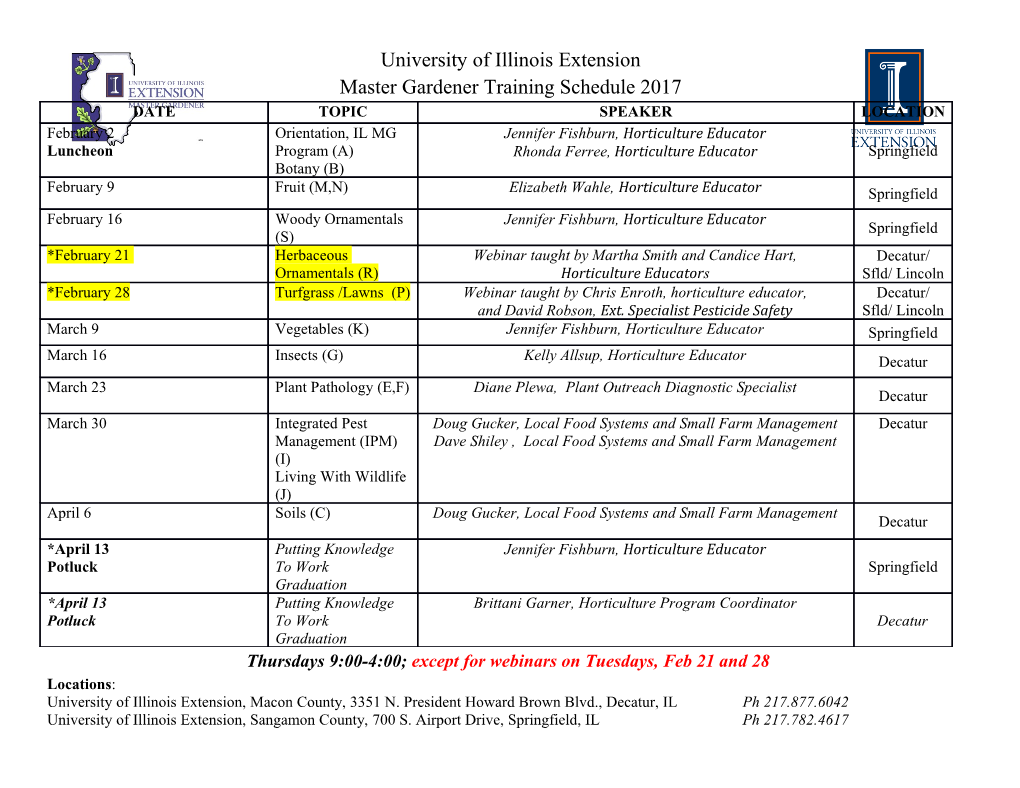
Leveraging Clustering and Natural Language Processing to Overcome Variety Issues in Log Management Tobias Eljasik-Swoboda1 a, and Wilhelm Demuth2 1ONTEC AG, Ernst-Melchior-Gasse 24/DG, 1100 Vienna, Austria 2SCHOELLER NETWORK CONTROL GmbH, Ernst-Melchior-Gasse 24/DG, 1100 Vienna, Austria [email protected], [email protected] Keywords: Industrial Applications of AI, Intelligence and Cybersecurity, Machine Learning, Natural Language Processing, Trainer/Athlete Pattern, Log Analysis, Log Management, Event Normalization, Security Information and Event Management, Big Data Abstract: When introducing log management or Security Information and Event Management (SIEM) practices, organizations are frequently challenged by Gartner’s 3 Vs of Big Data: There is a large volume of data which is generated at a rapid velocity. These first two Vs can be effectively handled by current scale-out architectures. The third V is that of variety which affects log management efforts by the lack of a common mandatory format for log files. Essentially every component can log its events differently. The way it is logged can change with every software update. This paper describes the Log Analysis Machine Learner (LAMaLearner) system. It uses a blend of different Artificial Intelligence techniques to overcome variety issues and identify relevant events within log files. LAMaLearner is able to cluster events and generate human readable representations for all events within a cluster. A human being can annotate these clusters with specific labels. After these labels exist, LAMaLearner leverages machine learning based natural language processing techniques to label events even in changing log formats. Additionally, LAMaLearner is capable of identifying previously known named entities occurring anywhere within the logged event as well identifying frequently co-occurring variables in otherwise fixed log events. In order to stay up-to-date LAMaLearner includes a continuous feedback interface that facilitates active learning. In experiments with multiple differently formatted log files, LAMaLearner was capable of reducing the labeling effort by up to three orders of magnitude. Models trained on this labeled data achieved > 93% F1 in detecting relevant event classes. This way, LAMaLearner helps log management and SIEM operations in three ways: Firstly, it creates a quick overview about the content of previously unknown log files. Secondly, it can be used to massively reduce the required manual effort in log management and SIEM operations. Thirdly, it identifies commonly co-occurring values within logs which can be used to identify otherwise unknown aspects of large log files. 1 INTRODUCTION organization. The process of generating, transmitting, storing, analyzing and disposing of log Computers and network components like switches, data is referred to as log management (Kent and routers or firewalls create log files that list events Souppaya, 2006). Centrally collecting log files from occuring on them. Originally, these log files were different components provides a number of tangible only used for troubleshooting problems after errors advantages. Firstly, log files can be accessed even if have occurred. Since then, technology has been the machine that originally generated them is no developed that can react immediately to the longer available. Secondly, central analysis and occurrence of specific events within logs. More correlation is only possible if the relevant log files interesting use cases arise when one combines log are centrally stored. The widespread deployment of files from multiple machines to get a bigger picture modern computing and networking machinery of events occuring within the IT environment of an generates challenges for organizations attempting to a https://orcid.org/0000-0003-2464-8461 leverage central log management. These challenges In this work, we introduce the Log Analysis are similar to those expressed by Gartner (2011) as Machine Learner (LAMaLearner). It uses a blend of the three Vs of Big Data: different artificial intelligence techniques in order to Firstly there is the problem of volume: The minimize the effort of implementing SIEM and log generated amount of log files can easily reach management practices within organizations. This is Terabytes. Secondly there is the problem of velocity especially relevant for Small or Medium Enterprises as depending on what is happening in the computing (SMEs) that oftentimes do not possess the necessary environment, log files can be generated at a rapid human resources to employ large teams focusing on pace. Scale-out architectures, that distribute the log management and SIEM. To do so, this paper workload across multiple machines are capable of outlines the relevant state of the art and technology handling these volume and velocity problems of this field in section 2. Section 3 describes our effectively (Singh & Reddy 2014). The third underlying model which is followed up by section 4 problem of big data is variety. In the case of log that provides some details about the implementation management, it stems from the lack of a mandatory of this technology. Section 5 evaluates the norm for log formats. Essentially, every log file is effectiveness of LAMaLearner for a number of different. Manually interpreting enormous amounts different log file formats and provides information of such log data can easily overwhelm a human about the time and effort that was saved by using being that attempts this task (Varanadi, 2003). LAMaLearner for Log Management projects. Last The process of transforming heterogenous log but not least, section 6 finishes with our conclusions formats to a common output that is centrally stored about the usage of AI to overcome variety is also referred to as event normalization (Teixeira, challenges in log management and SIEM. 2017). This is mainly achieved by using parsers that apply regular expressions to extract specific values from logs in previously known formats. These are 2 STATE OF THE ART subsequently stored in a normalized fashion within searchable databases. The data quality within these There are many tools for log management. Some databases is strongly dependant on the parsing frequently mentioned commercial options are quality. Even though parsing rules for common log Splunk, IBM QRadar, Loggly, Logentries, and sumo files are freely shared on the Internet, these usually logic (Splunk, 2019) (IBM, 2019) (Loggly, 2019) only match highly specific fields such as time (Logentries, 2019) (Sumo Logic, 2019). Most of the stamps and source ip adresses. The essential aforementioned systems are cloud based and require unstructured, natural language message gets a connection to the provider in order to perform the frequently stored as a string. necessary log management. A popular open source Security Information and Event Management solution is the Elastic Stack (formerly known as (SIEM) attempts to leverage centrally managed logs ELK Stack), which is maintained by the company to increase the IT security of an organization ElasticSearch which also offers support for the (Williams and Nicolett, 2005). In practice, specific solution (Elastic, 2019). This company’s full-text event types are oftentimes visualized by occurrence search engine goes by the same name and is an per time frame. For instance the amount of failed log integral part of the Elastic Stack. Another popular in attempts per hour. One can also define alert rules open source solution is Graylog which is maintained that trigger automated stepts. An example for such and supported by the company of the same name an alert rule are to inform a human being if there are (Graylog, 2019). To the best of our knowledge, all more than 10 firewall rejections per minute or that these log management solutions either require malware was detected on a computer within the manual pattern defintions to match relevant known network (Swift, 2010). Currently, the only way to events or provide taxonomies of relevant events for identify specific events is to know the log format in specific systems. None use artificial intelligence advance and having a pattern that can match to the technology for this purpose. In the context of log specific event during normalization. As pointed out, management, AI technologies are frequently used there is no norm for log events. Additionally, the for anomaly detection of aggregated event format of the log file can change with updates of the occurences per time frame instead of the utilized software. Therefore, the implementation and identification and representation of relevant events updating of Log Management and SIEM systems are (Splunk, 2019) (IBM, 2019) (Elastic, 2019). labor intensive. A detailed examination of these log management technologies as well as all contemporary AI techniques goes well beyond the scope of this paper. combines them with a SVM model that is evaluated Therefore the remainder of this section focuses on using n-fold cross-validation. TFIDF-SVM was relevant approaches useful for overcoming the evaluated in the challenging argument stance aforementioned log management variety problem. recognition task and achieved up to .96 F1 for Vaarandi (2003) proposes a data clustering previously unknown arguments about the same topic algorithm for mining patterns from event logs. it was trained on. Encouragingly, it was also able to Different from other text clustering approaches, this achieve up to .6 F1 when determining the stance of algorithm has the key insight,
Details
-
File Typepdf
-
Upload Time-
-
Content LanguagesEnglish
-
Upload UserAnonymous/Not logged-in
-
File Pages8 Page
-
File Size-