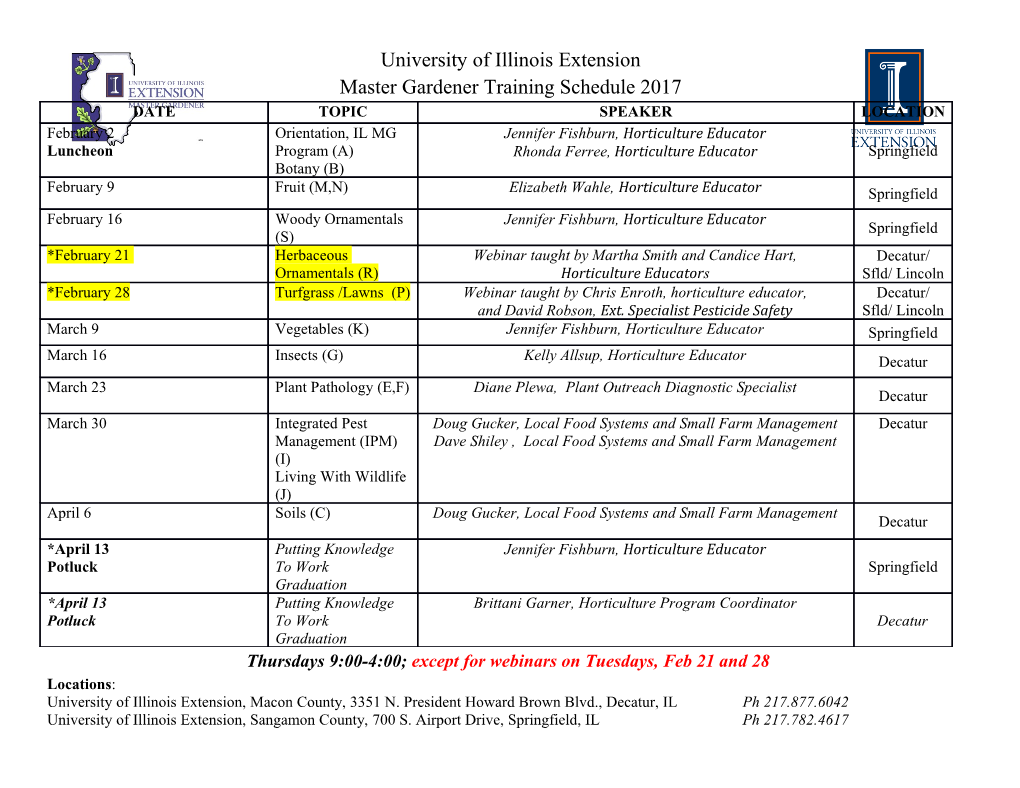
Measurement, 6: 25–53, 2008 Copyright © Taylor & Francis Group, LLC ISSN 1536-6367 print / 1536-6359 online DOI: 10.1080/15366360802035497 Latent Variable Theory Denny Borsboom University of Amsterdam This paper formulates a metatheoretical framework for latent variable modeling. It does so by spelling out the difference between observed and latent variables. This difference is argued to be purely epistemic in nature: We treat a variable as observed when the inference from data structure to variable structure can be made with certainty and as latent when this inference is prone to error. This difference in epistemic accessibility is argued to be directly related to the data- generating process, i.e., the process that produces the concrete data patterns on which statistical analyses are executed. For a variable to count as observed through a set of data patterns, the relation between variable structure and data structure should be (a) deterministic, (b) causally isolated, and (c) of equivalent cardinality. When any of these requirements is violated, (part of) the variable structure should be considered latent. It is argued that, on these criteria, observed variables are rare to nonexistent in psychology; hence, psychological variables should be considered latent until proven observed. Key words: latent variables, measurement theory, philosophy of science, psychometrics, test theory In the past century, a number of models have been proposed that formulate probabilistic relations between theoretical constructs and empirical data. These models posit a hypothetical structure and specify how the location of an object in this structure relates to the object’s location on a set of indicator variables. It is common to refer to the hypothetical structure in question as a latent structure and to the indicator variables as observed variables. In general, models that follow the idea set forth above are called latent variable models. There are several kinds of latent variable models, which are often categorized in terms of the types of observed and latent variables to which they apply. If the observed and latent variables are both continuous, then the resulting model is called a factor model (Jöreskog, 1971; Lawley & Maxwell, 1963; Correspondence should be addressed to Denny Borsboom, Department of Psychology, University of Amsterdam, Roetersstraat 15, 1018 WB Amsterdam. E-mail: [email protected] 26 BORSBOOM Bollen, 1989); if the observed variable is categorical and the latent variable is continuous, then we have an Item Response Theory (IRT) model (Rasch, 1960; Birnbaum, 1968; Hambleton & Swaminathan, 1985; Embretson & Reise, 2000; Sijtsma & Molenaar, 2002); if the observed and latent variables are both categorical, the resulting model is known as a latent class model (Lazarsfeld & Henry, 1968; Goodman, 1974); and if the observed variable is continuous while the latent variable is categorical, then we get a mixture model (McLachlan & Peel, 2000), which upon appropriate distributional assumptions becomes a latent profile model (Lazarsfeld & Henry, 1968; Bartholomew, 1987). However, various mixed forms of these models are possible. For instance, at the latent level, one may have several distinct systems of continuous latent variables that themselves define latent classes (Lubke & Muthén, 2005; Rost, 1990), and at the observed front these models may also relate to a mixture of categorical and continuous observed variables (e.g., Moustaki, 1996; Moustaki & Knott, 2000). In fact, any model that relates some kind of latent structure to an observed structure could be called a latent variable model; and the possibilities regarding the dimensionality and form of these structures are endless, as is the number of functions that can be used to relate one to the other. Like most statistical techniques, latent variable modeling is not an isolated statistical number crunching endeavor but part of a research procedure embedded in a set of more or less closely associated ideas, norms, and practices regarding the proper treatment of data in scientific research. The present paper represents an attempt to articulate these ideas, by articulating a fitting metatheoretical framework for latent variable modeling. To distinguish this framework from latent variable models themselves, we may indicate it with the term latent variable theory, which indicates that latent variable modeling is central to it and at the same time emphasizes that the theory is broader in scope than the purely statistical formulation of latent variable models. WHAT BINDS LATENT VARIABLE MODELS? Mathematically, latent variable models specify a generalized regression function that can be written as f(E(X))=g(), where f is a link function, E is the expec- tation operator, X denotes a matrix of observed variables, is a latent structure, and g is some function that relates the latent structure to the observed variables. If, upon a suitable choice of f, the function g is linear, then the resulting family of models is covered by Generalized Linear Item Response Theory (Mellenbergh, 1994). This is true for most of the models used in factor analysis and IRT. By expanding the matrices X and to apply to series of observations made at different time points, models for time series, like the hidden Markov model (Rabiner, 1989; Visser, Raijmakers, & Molenaar, 2002) or the dynamic factor LATENT VARIABLE THEORY 27 model (Molenaar, 1985), may be formulated; it is also possible to model inter- and intraindividual differences simultaneously (Hamaker, Molenaar, & Nessel- roade, 2007). Thus, a latent variable model is simply a model that relates the expectation of observables to a latent structure through some regression function. However, most people working in latent variable modeling have a strong intuition that the group of latent variable models comprises a homogeneous structure, in the sense that they have something in common that separates them from other commonly used statistical models (say, analysis of variance or principal compo- nents analysis). It is, however, useful to note that no such delineation follows from the mathematical structure of the model. Mathematically, all that is being said in this structure is that the expectation of some set of variables is a function of another set of variables, and it is difficult to say why this should specify a latent variable model. In fact, if we should take the mathematical structure itself to define latent variable models, then virtually all statistical techniques count as latent variable models, because it is in the nature of statistical techniques to specify a relation between the expectation of one set of variables and another set of variables. Hence, on this basis a latent variable model would be indistin- guishable from, say, analysis of variance, a technique that one intuitively feels should not be included as a latent variable model. If one wants to explain what binds latent variable models, an appeal to the mathematical structure of the model does not do the trick. Clearly, what makes a latent variable model a latent variable model is not the mathematical structure that is being used to link different sets of variables. Rather, the important feature of the regression function central to latent variable models is that the left-hand side of the equation contains a set of observed variables, whereas the right-hand side contains a latent structure. Hence, if we insist on distinguishing latent variable models from observed variable models, we need to make clear what this distinction consists in. LATENT AND OBSERVED VARIABLES What is the difference between latent and observed variables? The use of the term latent, but especially the term observed, suggests that this distinction is of an epistemological character. Observed variables are variables that are somehow epistemically accessible to the researcher, whereas latent variables are not epistemically accessible. It is customary to illustrate this distinction with substantive examples. Thus, one says that IQ scores are recorded, but general intelligence is not; hence general intelligence is a latent variable and IQ an observed variable. However, in order to characterize latent variable models generally, it is not sufficient to point to some illustrative examples. Neither is 28 BORSBOOM it clarifying to give a tautological, and hence not very informative, characteri- zation of latent variables, as is not uncommon in the literature, for instance when scholars say that “a latent variable is a variable that is not directly measured,” or “a latent variable is a theoretical construct,” or “a latent variable is a variable that underlies the observations.” It is important to understand somewhat more precisely what the distinction between observed and latent variables amounts to. This matter is not entirely straightforward. The reason for this is not so much that the concept of a latent variable, as a hypothetical structure of inter- or intraindividual differences, is problematic, but rather that it is difficult to grasp the idea that a variable might be observed. Take familiar examples of variables that, in statistical analyses, are commonly conceptualized as observed variables, such as sex or age. It is hard to uphold the idea that the distinction between these variables and variables that are seen as latent, such as general intelligence, lies in the fact that the first are observed whereas the second are not. In a strict reading of the word observed, nobody can claim to have observed sex, length, or age. These are theoretical constructs just as well as general intelligence is. Age is not a concrete object, subject to our perceptual processes, like stones or trees or people might be taken to be, but a theoretical dimension. Theoretical dimensions do not fall in the category of observable things. Thus, when one says that one has learned, upon interrogation of the twins John and Jane, that John is 15 minutes older than Jane, one cannot claim to have thereby observed the variable age. On the basis of one’s observation of John and Jane, one has made an inference regarding their relative positions on the dimension age, but it is not thereby true that one has observed age itself.
Details
-
File Typepdf
-
Upload Time-
-
Content LanguagesEnglish
-
Upload UserAnonymous/Not logged-in
-
File Pages29 Page
-
File Size-