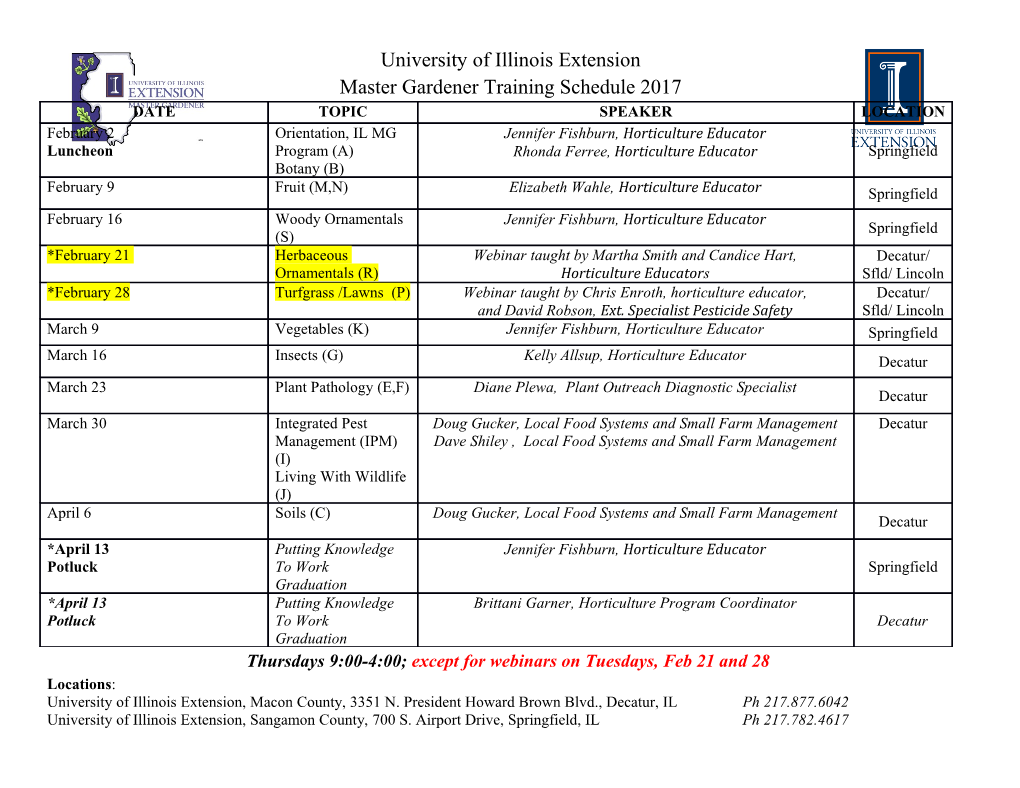
Distinguishability notion based on Wootters statistical distance: application to discrete maps Ignacio S. Gomez,1, ∗ M. Portesi,1, y and P. W. Lamberti2, z 1IFLP, UNLP, CONICET, Facultad de Ciencias Exactas, Calle 115 y 49, 1900 La Plata, Argentina 2Facultad de Matem´atica, Astronom´ıa y F´ısica (FaMAF), Universidad Nacional de C´ordoba, Avenida Medina Allende S/N, Ciudad Universitatia, X5000HUA, C´ordoba, Argentina (Dated: August 10, 2018) We study the distinguishability notion given by Wootters for states represented by probability density functions. This presents the particularity that it can also be used for defining a distance in chaotic unidimensional maps. Based on that definition, we provide a metric d for an arbitrary discrete map. Moreover, from d we associate a metric space to each invariant density of a given map, which results to be the set of all distinguished points when the number of iterations of the map tends to infinity. Also, we give a characterization of the wandering set of a map in terms of the metric d which allows to identify the dissipative regions in the phase space. We illustrate the results in the case of the logistic and the circle maps numerically and theoretically, and we obtain d and the wandering set for some characteristic values of their parameters. PACS numbers: 05.45.Ac, 02.50.Cw, 0.250.-r, 05.90.+m Keywords: discrete maps { invariant density { metric erarchy [11], with quantum extensions [12{16] that allow space { wandering set to characterize aspects of quantum chaos [17]. The rele- vance of discrete maps lies in the fact that they serve as simple but useful models in biology, physics, economics, I. INTRODUCTION etc [18{20]. Specifically, they have proven to be powerful tools for testing features of chaotic and complex phenom- ena [21{24]. The concept of distance constitutes, in mathematics but also in physics, a measure of how apart two \objects" In the case of the logistic map [18, 19], from the in- are. Depending on the context, a distance may refer to a variant density Johal [25] proposed a statistical distance physical length or to a metric function which gives place which serves to characterize the chaotic regime. In this to the theory of metric spaces [1{3]. In physics, it is well paper we explore, analytically and numerically, this idea known that the information about a system is contained by redefining the distance given in Ref. [25] as a metric, in the state function, the evolution of which accounts for and we apply this to characterize two emblematic chaotic the features of the dynamics. When there is a limitation maps: the logistic map and the circle map. We focus or uncertainty about the knowledge of the system (clas- on some values of their parameters which are in corre- sical or quantum), it is common to consider the states as spondence to the characteristic regimes of the dynamics. represented by probability density functions. Distances Moreover, we define a metric space associated to values between probability density functions give so called sta- of the parameters of each map that takes into account tistical measures of distinguishability between states [4]. the topology of the phase space according to dynamics. Many statistical distances have been defined and for sev- eral purposes, like Kullback divergence [5], Wootters [6] The work is organized as follows. In Section II we in- and Monge [7] distances in quantum mechanics, or the arXiv:1610.07941v2 [nlin.CD] 18 Mar 2017 troduce the notions used throughout the paper. Section metric distance given by the Fisher{Rao tensor in infor- III is devoted to recall the proposal of a statistical dis- mation geometry [8]. tance made by Johal [25] and, using equivalence classes, In the context of discrete maps, one of the most impor- we redefine it as a metric. Next, we propose a metric tant statistical features of underlying dynamics is given space, induced by this metric, composed by all the phase by the probability distribution that is a fixed point of space points that can be distinguished in the limit of the corresponding Frobenius{Perron operator associated large number of iterations of the map. From the metric, to the map [9, 10]. This is the so called invariant den- we give a characterization of the wandering set of a map sity which plays an important role in ergodic theory, i.e. that allows one to classify the dissipative regions of the the study of the measures that are invariant under the dynamics in phase space. Considering some characteris- invariant densities [11]. Relevant properties that lay at tic values of their parameters, in Section IV we illustrate the foundations of statistical mechanics like ergodicity the results for the logistic map and for the circle map. In and mixing are described in terms of invariant densities section V we draw some conclusions and outline future by means of the corresponding levels of the ergodic hi- research directions. 2 II. PRELIMINARIES way. The Frobenius{Perron operator P : L1(Γ) ! L1(Γ) associated to the map (3) is given by [9] We recall some notions from probability theory, dis- Z Z crete maps and metric spaces used throughout the paper. P φ(x)dx = φ(x)dx A f −1(A) for all φ 2 1(Γ) and A ⊆ Γ, where f −1(A) is the preim- A. Probability density and cumulative distribution L age of A. Any nonnegative function ρ 2 1(Γ), normal- functions L ized to 1 in Γ, and such that A probability density function defined over an abstract P ρ(x) = ρ(x) 8 x 2 Γ space Γ (typically, a subset of Rm) is any nonnegative is called invariant density of the map. Mathematically, function p :Γ ! R+ such that invariant densities are a special case of invariant measures Z p(x)dx = 1 (1) [9]. In dynamical systems theory, the invariant densities Γ represent stationary states which allows one to study the where Γ is called the space of events. For instance, in an system in the asymptotic limit of large times [26]. As experiment, Γ represents the set of all possible outcomes an example, in the case of the logistic map in its chaotic of the phenomenon being observed. If Γ is composed by regime one has f(x) = 4x(1 − x) and Γ = [0; 1]. It is a discrete, say N, number of results then one has a dis- found that [9, p. 7] crete probability distribution which can be represented t P f(x) by a column vector (p1; p2; ··· ; pN ) , where pi stands for p p = p1 f 1 − 1 1 − x) + f( 1 + 1 1 − x the probability that the ith result occurs, and the nor- 4 1−x 2 2 2 2 malization condition (1) now reads PN p = 1. i=1 i for all f 2 1([0; 1]). By successively applying the op- Given the probability distribution p with Γ ⊆ , the L R erator P over any initial distribution f (i.e. P f; P 2f = so called cumulative distribution function associated to P (P f);P 3f = P (P (P f));:::) one obtains an analytical p(x) is defined as expression for the invariant density ρ(x) in the limit as Z [9, p. 7] C(x) = p(t)dt (2) ft≤xg 1 ρ(x) = lim P nf(x) = ; x 2 (0; 1) C(x) is the probability that the variable takes a value less n!1 πpx(1 − x) than or equal to x. When Γ is composed by a number Pi In general, except for some particular maps, the invariant N of results, the definition (2) reads as Ci = j=1 pj. The cumulative distribution function is frequently used density has no analytical expression, and therefore one in statistical analysis, since an estimation of C(x) can be has to compute it numerically [24]. given directly from the empirical distribution function in The method consists of constructing an histogram to terms of the experimental data. show the frequency with which states along a sequence fxng fall into given regions of phase space Γ. In order to illustrate the procedure, let us consider Γ = [0; 1]. B. Discrete maps and invariant densities Then, we simply divide the interval [0; 1] into M discrete nonintersecting intervals of the form Given a set Γ and a continuous function f :Γ ! Γ, it i − 1 i [ ; ) i = 1;:::;M is said that the sequence fxngn2N0 ⊆ Γ such that M M xn+1 = f(xn) 8 n 2 N0 ; x0 2 Γ (3) Next step is to consider an initial state x0 and calculate the trajectory defines a discrete map. More generally, one can allow f T to have finite discontinuities. From the physical view- x0 ; x1 = f(x0) ; x2 = f(f(x0)) ; : : : ; xT = f (x0) point, a discrete map models a system, the dynamics of which is given by iterating Eq. (3) where each iteration of length T with T M. The fraction ρi of the T states corresponds to a time step. That is, if the system is ini- visiting the ith interval is tially in a state x0, then xn represents the state after n i−1 i ]fxτ 2 [ ; ) j τ = 0; 1;:::;T g time steps. If there exists an element c 2 Γ such that ρ = M M ; i = 1;:::;M i T f(c) = c, it is said that c, is a fixed point of f. Fixed points are physically interpreted as stationary states of Finally, the invariant density ρ is given by the system subject to the map.
Details
-
File Typepdf
-
Upload Time-
-
Content LanguagesEnglish
-
Upload UserAnonymous/Not logged-in
-
File Pages9 Page
-
File Size-