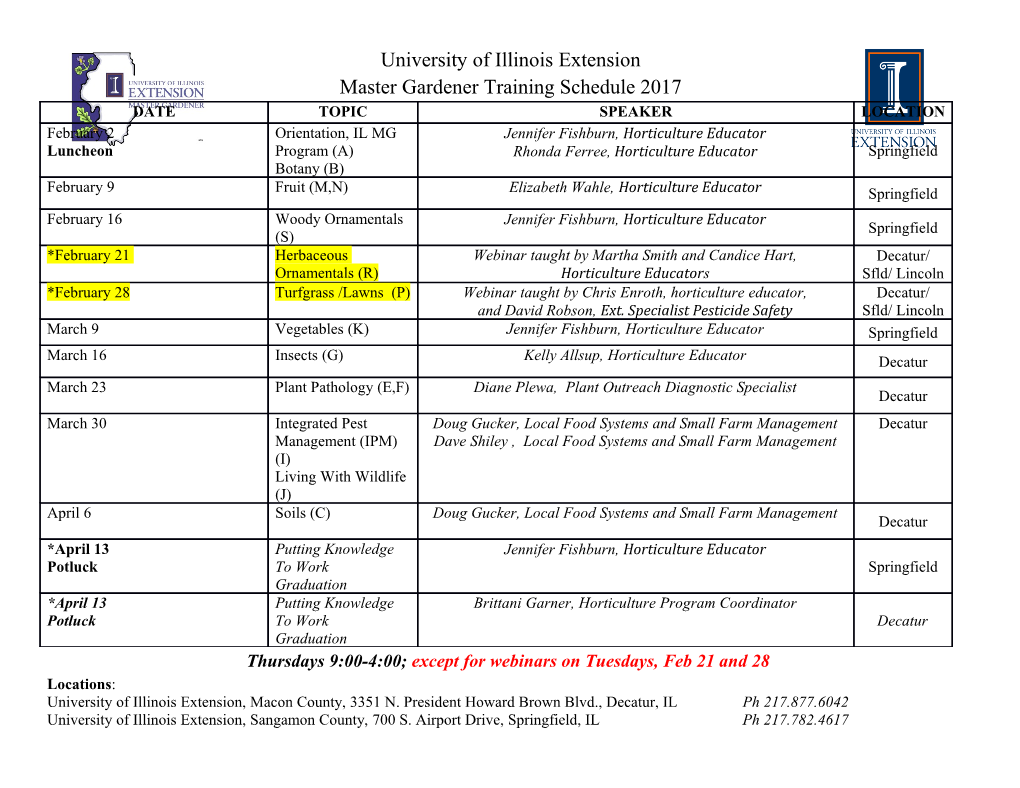
Anomaly Detection in Ethereum Transactions Using Network Science Analytics A thesis submitted to the Graduate School of the University of Cincinnati in partial fulfillment of the requirements for the degree of Master of Information Technology in the School of Information Technology of the College of Education, Criminal Justice and Human Services. by Yusuf Lanre Lawal B.Sc University of Lagos April 2014 Committee Chair: Bilal Gonen, Ph.D. Abstract Since the introduction of Bitcoin, the rate of adoption of blockchain technology has exponentially increased. Consequently, numerous other types of cryptocurrencies, such as Ethereum, have been introduced. The high rate of adoption of cryptocurrencies has resulted in the generation of enor- mous amounts of data. In this paper, we focus on detecting anomaly or outliner in the daily Ethereum network using network properties. We were able to use the network properties and data mining in getting the required results. Wallets or accounts acting on the blockchain are repre- sented as nodes, while interactions between wallets or accounts are represented as links or edges. Based on the explanation, we were able to discover how the network properties have an impact on transaction behavior within the network. we propose how this analysis would be useful in real-life events. Acknowledgements I would like to thank my Supervisor, Dr. Bilal Gonen, for his support and Dr. Ki Jung Lee for their guidance during this project. I would also like to send a warm thank you to Lauren Kirgis (Graduate Coordinator). I would send a big shout out to Adedapo Alabi who showed me support from day one and to my other friends who support me even though it can be annoying sometimes. Finally, I am indebted to my mum, Mr & Mrs Akinde, and siblings for being supportive. Thank you all for showing me love, for always lifting up my spirit and also for providing financial support throughout my gradate school journey. Contents 1 Introduction 6 1.1 Background of the Study . .6 1.2 Motivation of the Study . .7 1.3 Structure of the Study . .7 2 Literature Review 8 2.1 Introduction . .8 2.2 Related Work and Data Mining Background . .8 2.3 Applicable Data Mining Algorithms . 10 2.4 Overview of the Clustering Algorithms . 10 2.4.1 The Spectral Clustering Algorithm . 10 2.4.2 K-Means Clustering . 11 2.4.3 Ordering Points to Identify the Clustering Structure (OPTICS) Algorithm . 11 2.4.4 The Mean Shift Algorithm . 12 2.4.5 Mini-Batch K-Means . 12 2.5 Overview of Blockchain Technology . 12 2.5.1 The Architecture of Blockchain . 13 2.5.2 Digital Signatures in Blockchain . 13 2.5.3 The Primary Characteristics of Blockchain . 14 2.6 Background of Cryptocurrencies . 14 2.6.1 Overview of Ethereum . 14 2.6.2 Technical Background of Ethereum . 16 2.6.3 The Ethereum Transaction . 17 2.7 Application of Network Science in the Project . 20 2.7.1 Assortativity Coefficient . 21 2.7.2 Size . 21 2.7.3 Order . 21 2.7.4 Average Degree . 21 2.7.5 Degree of Centrality . 21 3 Methodology 22 3.1 Design . 22 3.1.1 Selection of a Suitable Algorithm . 22 3.1.2 Silhouette Analysis . 22 3.1.3 Procedure for Algorithm Selection . 23 3.1.4 Determining the Number of Clusters . 23 3.1.5 Data Dimensionality . 24 2 3.1.6 Ethereum Data Selection and Storage Formats . 25 3.1.7 Choice Programming Language . 25 3.2 Data Collection . 25 3.2.1 Procedure . 25 3.2.2 External Data Collection . 27 3.3 Challenges Encountered During the Mining process . 27 4 Results and Analysis 28 4.1 Discussion of Results . 28 4.1.1 Centrality degree . 30 4.1.2 Degree Assortativity . 32 4.1.3 Average Degree . 33 4.1.4 Size & Order . 33 5 Discussion 34 5.1 Application in Attack Forensics . 34 5.2 Application in Detection of Anomalies on the Network . 34 5.3 Deanonymization of Transactions . 35 5.4 Limitation of the Study . 35 6 Conclusion and Future Works 36 6.1 Conclusion . 36 6.2 Recommendations . 36 Bibliography 40 A Code for Extracting the Data 41 3 List of Figures 2.1 Weighted and Unweighted Edges . 10 2.2 An illustration of the blockchain. 13 2.3 A descriptive picture of how cryptocurrency works. 15 2.4 An Illustration of the Ethereum Transaction . 17 2.5 A visualization of the Ether transactions. 20 3.1 Graph Showing how the Number of Clusters Correlates with Silhouette Score. 24 4.1 The graph shows the ETH-USD Timeline. 28 4.2 The image shows the period of which they were anomalies in the cluster. 29 4.3 DELEGATECALL METHOD . 30 4.4 A FALLBACK METHOD . 30 4.5 An image showing the high activities for Degree Centrality . 31 4 List of Tables 3.1 Table showing the Silhouette Score all the Suitable Clustering Algorithms ...... 23 3.2 Table Showing Silhouette Scores of Various Number of Clusters ........... 24 4.1 The values of the various metrics are as presented in the table ............ 29 5 Chapter 1 Introduction 1.1 Background of the Study Blockchain was revolutionary when it was implemented in 2009 and was not widely accepted from its inception. It was introduced with Bitcoin to solve the double-spending problem. Like any new disruptive technology, they were concerns about the volatility of the cyrptocurrency and ease of use. Bitcoin was an invention of a computer scientist or it can be a group of people called Satoshi Nakamoto. The digital currency was invented to do away with the intermediary parties involved in transactions. Bitcoin used public keys address for sending and receiving bitcoin, the recorded transactions and the personal ID will remain anonymous [1]. Bitcoin transactions utilize cryptographic protocols to provide a secure process while striving to preserve the privacy of both the buyer and seller [2]. The popularity of cryptocurrencies has been exponentially growing since the introduction of Bitcoin. Although there are numerous types of cryptocurrencies, Bitcoin and Ethereum are the most popular cryptocurrencies [3]. The two popular cryptocurrencies are used to complete numer- ous types of transactions, including shopping, banking, forex trading, etc. According to Statista, a significant percentage of cryptocurrency transactions are done using Ethereum[4]. Statista further estimates that, on average, in the first quarter of 2020, there were approximately 753,510 daily Ethereum transactions [4]. Every successful Ethereum transaction is accompanied by valuable data. Since thousands of transactions are carried out daily, a large amount of data is generated. A significant percentage of the data is of no use until it is further processed into useful information. Therefore, it is essential to analyze the large dataset using data mining techniques to unearth use- ful and interesting data structures from the transactions. Data mining on Ethereum transactions is essential for numerous purposes. Using data mining, it is possible to detect anomalies, such as fraud, in the transactions. Although transactions using Ethereum are anonymous, data mining can be used to classify the various types of transactions [5]. 6 In this study, the aim is to use network science and unsupervised machine learning to detect anomaly or outliner in the daily Ethereum transaction. The flow of transaction is represented the daily transaction of Ether on the Ethereum network. In the Ethereum scenario, a transaction can represent some cryptocurrency transfer [6]. For every transaction that occurs in the Ethereum network formed a link within the network. The reason behind the analysis is that complex network provide network properties to show the structure of the network and using data mining algorithm to find patterns within the network [7][6][8]. The sample of the transactions extracted was done at random, from the Ethereum network. The analysis done in this project leads to an observation that an external event can change the structure of the network properties. The sample of the transactions extracted was done at random, from the Ethereum network. The analysis done in this project shows an event can change the structure of the network properties. For example, It was observed that they were periods with high volumes of transactions between hubs and nodes. The hubs have a higher presence of higher degree, likewise some period with lower degree, meaning the transactions between hub and nodes was lower. 1.2 Motivation of the Study The aim of this project is to use network science and unsupervised machine learning to detect anomaly or outlier in the daily Ethereum transactions. The network properties used in the analysis include the degree of centrality, order, size, average degree, and assortativity coefficient. 1.3 Structure of the Study The study is divided into six distinct sections: the introduction, literature review and related work, methodology, results and analysis, discussion, conclusion, and future work. The introduction section presents a brief overview of the research background and motivations. The Literature review and related work section presents the technical background essential in effectively understanding and interpreting the study results. The results and analysis section presents the outcomes obtained from the data mining process. The discussion section critically discusses the analyzed results in relation to existing peer-reviewed literature. Lastly, the conclusion summarizes the findings of the study and presents the significant inferences identified it. 7 Chapter 2 Literature Review 2.1 Introduction In this section, the essential information required in the implementation of the project is presented. The section is mainly divided into three sections: background information on blockchain and cryp- tocurrencies, background information on data mining and clustering algorithms, and the project’s potential implementation matrices. 2.2 Related Work and Data Mining Background Since the Blockchain was established, it has become the focal point of different research topics.
Details
-
File Typepdf
-
Upload Time-
-
Content LanguagesEnglish
-
Upload UserAnonymous/Not logged-in
-
File Pages57 Page
-
File Size-