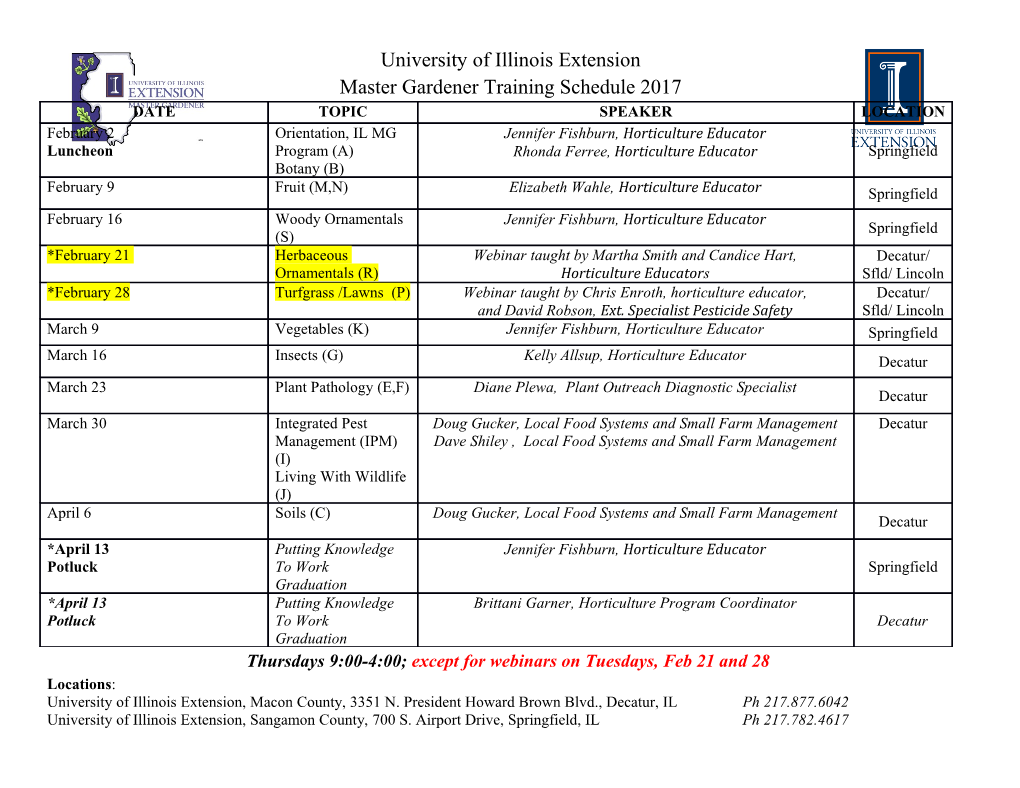
The mechanoresponse of bone is closely related to the osteocyte lacunocanalicular network architecture Alexander Franciscus van Tola,b,1, Victoria Schemenza,b, Wolfgang Wagermaiera, Andreas Roschgera,c, Hajar Razia, Isabela Vitienesd,e, Peter Fratzla, Bettina M. Willied,e, and Richard Weinkamera,1 aDepartment of Biomaterials, Max Planck Institute of Colloids and Interfaces, 14476 Potsdam, Germany; bBerlin-Brandenburg School for Regenerative Therapies, Charité–Universitätsmedizin Berlin, 13353 Berlin, Germany; cDepartment of Chemistry and Physics of Materials, Paris Lodron University of Salzburg, A-5020 Salzburg, Austria; dResearch Centre, Shriners Hospital for Children-Canada, Montreal, H4A 0A9, Canada; and eDepartment of Pediatric Surgery, McGill University, Montreal, H3A 0G4, Canada Edited by Lia Addadi, Weizmann Institute of Science, Rehovot, Israel, and approved November 1, 2020 (received for review June 4, 2020) Organisms rely on mechanosensing mechanisms to adapt to changes materials, where the fluid-filled network should impart self-healing in their mechanical environment. Fluid-filled network structures not properties to the material (10, 11). only ensure efficient transport but canalsobeemployedformecha- In bone, fluid flow occurs in the lacunocanalicular network nosensation. The lacunocanalicular network (LCN) is a fluid-filled net- (LCN), a porous network of micrometer-sized lacunae con- work structure, which pervades our bones and accommodates a cell nected by roughly 300-nm-wide canals, called canaliculi (12, 13). network of osteocytes. For the mechanism of mechanosensation, it The lacunae accommodate the cell bodies of osteocytes, while was hypothesized that load-induced fluid flow results in forces that their cell processes run within the canaliculi. The pericellular can be sensed by the cells. We use a controlled in vivo loading ex- space between the cell membrane and the mineralized bone periment on murine tibiae to test this hypothesis, whereby the tissue is filled by a proteoglycan-rich matrix (glycocalyx) and by mechanoresponse was quantified experimentally by in vivo micro- an interstitial fluid. Deformations occurring through the bone computed tomography (μCT) in terms of formed and resorbed bone matrix are too small to be directly sensed by bone cells since volume. By imaging the LCN using confocal microscopy in bone vol- bone has a high stiffness, compared to cells, of roughly 10 GPa. umes covering the entire cross-section of mouse tibiae and by calcu- Therefore, a strain amplification mechanism involving the in- lating the fluid flow in the three-dimensional (3D) network, we could terstitial fluid was proposed (12). The fluid flow hypothesis states perform a direct comparison between predictions based on fluid that external loading deforms the bone including the porous BIOPHYSICS AND COMPUTATIONAL BIOLOGY flow velocity and the experimentally measured mechanoresponse. network. The deformation of the porous network induces a flow While local strain distributions estimated by finite-element analysis of the fluid through the LCN toward or away from free bone incorrectly predicts preferred bone formation on the periosteal sur- surfaces (14). With typical loading frequencies (such as walking) face, we demonstrate that additional consideration of the LCN archi- being in the 1-Hz range, the load-induced fluid flow should not tecture not only corrects this erroneous bias in the prediction but be imagined as a substantial fluid transport, but rather as fluid also explains observed differences in the mechanosensitivity be- oscillating back and forth. The oscillating fluid flow results in tween the three investigated mice. We also identified the presence drag forces on osteocytes that are sufficiently strong to trigger a of vascular channels as an important mechanism to locally reduce fluid flow. Flow velocities increased for a convergent network struc- ture where all of the flow is channeled into fewer canaliculi. We Significance conclude that, besides mechanical loading, LCN architecture should be considered as a key determinant of bone adaptation. The explanation of how bone senses and adapts to mechanical stimulation still relies on hypotheses. The fluid flow hypothesis bone adaptation | mechanobiology | fluid flow | lacunocanalicular claims that a load-induced fluid flow through the lacunocana- network | in vivo μCT licular network can be sensed by osteocytes, which reside within the network structure. We show that considering the network architecture results in a better prediction of bone luid-filled network structures are used in many organisms for remodeling than mechanical strain alone. This was done by transport and signaling by making use of their excellent per- F calculating the fluid flow through the lacunocanalicular net- vasion of tissues, while requiring only a limited volume. A variety work in bone volumes covering the complete cross-sections of of tissues and organs use fluid flow in networks to sense the me- mouse tibiae, which underwent controlled in vivo loading. The chanical environment, thereby contributing to their morphogene- established relationship between mechanosensitivity and net- sis, active maintenance, and adaptation to changing demands (1). work architecture in individual animals implies possibilities for Important examples include the formation of the circulation and patient-specific therapies. A new connectomics approach to nervous system (2, 3), both the rapid and long-term adaptation of analyze lacunocanalicular network properties is necessary to the lungs (4), and the adaptation of bone to mechanical loads understand skeletal mechanobiology. (5–7). Important distinguishing characteristics between networks Author contributions: W.W., P.F., B.M.W., and R.W. designed research; A.F.v.T. and H.R. are the mechanical flexibility of the walls of their channels and their performed research; A.F.v.T., V.S., A.R., H.R., and B.M.W. contributed new reagents/ana- network architecture. The cardiovascular circulation is an example lytic tools; A.F.v.T., I.V., P.F., B.M.W., and R.W. analyzed data; and A.F.v.T. and R.W. wrote of a tree-like network with channels repeatedly branching, which the paper with contributions from all authors. induces constraints on the diameters of the vessels for an efficient The authors declare no competing interest. flow distribution (8). The circulation network is not static but can This article is a PNAS Direct Submission. adapt its network architecture, for example, by maintaining or dis- This open access article is distributed under Creative Commons Attribution License 4.0 connecting arterial side branches based on locally sensed blood flow (CC BY). 1To whom correspondence may be addressed. Email: [email protected] or velocities (2). The multifunctionality of fluid-filled networks is in- [email protected]. teresting from an evolutionary viewpoint, since most likely the This article contains supporting information online at https://www.pnas.org/lookup/suppl/ functions were not established at the same point in time (9). Their doi:10.1073/pnas.2011504117/-/DCSupplemental. potential has also been explored in man-made so-called vascular First published December 7, 2020. www.pnas.org/cgi/doi/10.1073/pnas.2011504117 PNAS | December 22, 2020 | vol. 117 | no. 51 | 32251–32259 Downloaded by guest on September 28, 2021 mechanoresponse (15). Important findings in favor of the fluid performed using a custom software (36). 6) Circuit theory was ap- flow hypothesis are as follows: 1) osteocytes are the most mecha- plied to calculate the load-induced fluid flow in each of the millions nosensitive cells in bone (16, 17), in particular to pulsatile fluid flow of imaged canaliculi (45), and a mechanical stimulus was inferred (18) with their cell processes sensing shear forces (19); the primary (41), which is used as a predictor of the mechanoresponse of cilium could act as an additional mechanosensor for fluid flow (20); the tibia. 2) dynamic loads induce fluid displacement through the LCN in vivo (21); 3) theoretical models predict load-induced fluid flow Results velocities of magnitudes, which osteocytes respond to in vitro (22); Structural Heterogeneity of the Mouse LCN. The tibiae of three 4) specific detection mechanisms have been proposed, which stress 26-wk-old female C57BL/J6 mice underwent 2 wk of controlled the importance of the glycocalyx with its tethering fibers for the loading, and the LCN within these tibiae was imaged afterward. transmission of forces to cell processes (23). In addition, the cell The 3D structure obtained by confocal imaging of the LCN in process is attached by integrins to canalicular projections, which are whole cross-sections of the tibia (Fig. 1A) revealed a heterogeneity infrequent, discrete locations along the canalicular wall (19, 24, 25). of the LCN with regions of looser (Fig. 1B) and denser network While important progress was made in characterizing molec- (Fig. 1C) (for videos of the full image stacks, see clsm_mouse1/2/ ular and cellular aspects of bone’s mechanotransduction, the connection between mechanical stimulation of the bone and its 3.avi in ref. 46). A quantitative analysis of the network density in mechanoresponse in the form of bone formation and resorption terms of canalicular density, Ca.Dn, (i.e., total length of canaliculi is still not satisfactorily established. The challenge is to define a mechanical stimulus, which can be spatially correlated to loca- tions of bone formation and resorption.
Details
-
File Typepdf
-
Upload Time-
-
Content LanguagesEnglish
-
Upload UserAnonymous/Not logged-in
-
File Pages9 Page
-
File Size-