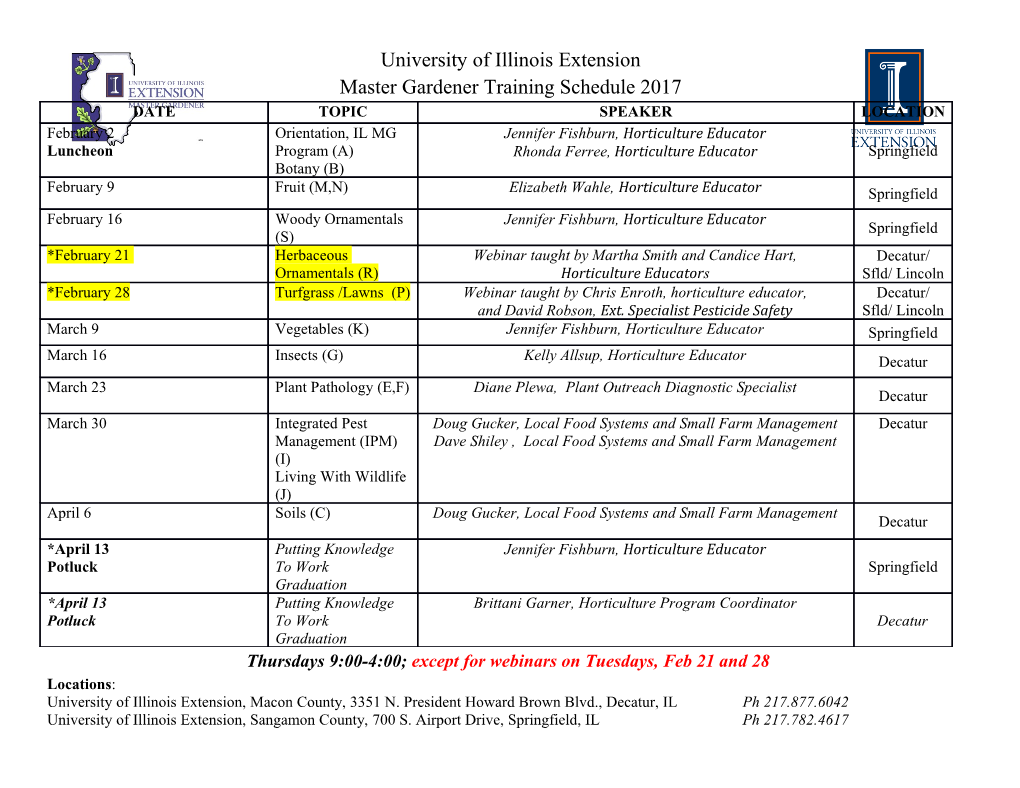
Journal of Experimental Psychology: © 2018 American Psychological Association Learning, Memory, and Cognition 0278-7393/18/$12.00 http://dx.doi.org/10.1037/xlm0000548 2018, Vol. 44, No. 12, 1880–1910 Time in Causal Structure Learning Neil R. Bramley Tobias Gerstenberg New York University Massachusetts Institute of Technology Ralf Mayrhofer David A. Lagnado University of Göttingen University College London A large body of research has explored how the time between two events affects judgments of causal strength between them. In this article, we extend this work in 4 experiments that explore the role of temporal information in causal structure induction with multiple variables. We distinguish two qualita- tively different types of information: The order in which events occur, and the temporal intervals between those events. We focus on one-shot learning in Experiment 1. In Experiment 2, we explore how people integrate evidence from multiple observations of the same causal device. Participants’ judgments are well predicted by a Bayesian model that rules out causal structures that are inconsistent with the observed temporal order, and favors structures that imply similar intervals between causally connected components. In Experiments 3 and 4, we look more closely at participants’ sensitivity to exact event timings. Participants see three events that always occur in the same order, but the variability and correlation between the timings of the events is either more consistent with a chain or a fork structure. We show, for the first time, that even when order cues do not differentiate, people can still make accurate causal structure judgments on the basis of interval variability alone. Keywords: causal learning, structure induction, time, order, Bayesian model Many aspects of higher level cognition, including prediction, system (Bramley, Lagnado, & Speekenbrink, 2015; Meder, May- explanation, and goal-directed action, depend on representing the rhofer, & Waldmann, 2014; Sloman & Lagnado, 2005; Steyvers, causal structure of the world (Sloman, 2005; Tenenbaum, Kemp, Tenenbaum, Wagenmakers, & Blum, 2003). However, people Griffiths, & Goodman, 2011). But, how do people learn this utilize a range of sources of information in causal learning (Lag- structure? Research has predominantly focused on learning from nado, Waldmann, Hagmayer, & Sloman, 2007) and human causal statistical covariation between variables (Cheng, 1997; Deverett & knowledge goes beyond mere expectations about covariation (Ger- Kemp, 2012; Gopnik, Sobel, Schulz, & Glymour, 2001; Perales & stenberg, Goodman, Lagnado, & Tenenbaum, 2015; Sloman & Shanks, 2007) sometimes involving active interventions on the Lagnado, 2015). In this article, we focus on the role of time in causal structure induction. To be able to predict and diagnose causality in real- world situations, we must form expectations about how long dif- This article was published Online First May 10, 2018. ferent relationships take to work (Griffiths & Tenenbaum, 2009; Neil R. Bramley, Department of Psychology, New York University; Lagnado & Sloman, 2006). For example, we learn to expect a Tobias Gerstenberg, Department of Brain and Cognitive Sciences, Massa- radiator to heat up within a few minutes of turning on the boiler; chusetts Institute of Technology; Ralf Mayrhofer, Institute for Psychology, to feel an effect of a pill after around half an hour; and for the TV University of Göttingen; David A. Lagnado, Department of Experimental to come on a second or two after we press “power” on the remote. This document is copyrighted by the American Psychological Association or one of its alliedPsychology, publishers. University College London. Such expectations, in turn, support structure inference (Buehner & This article is intended solely for the personal use of the individual userWe and is not to be dedicate disseminated broadly. this article to Jon Oberlander, an inspiring, original cogni- tive scientist and philosopher who was an important early influence for McGregor, 2006; Griffiths & Tenenbaum, 2009; Hagmayer & Neil R. Bramley but whose time was all too short. Waldmann, 2002; Kemp, Goodman, & Tenenbaum, 2010). If the We also thank Christos Bechlivanidis, Michael Pacer, and Toby Pilditch stereo comes on shortly after we press “power” on the remote, we for helpful comments. Neil R. Bramley is supported by the Moore-Sloan wonder whether we have picked up the wrong remote. If the TV Data Science Environment at NYU. Tobias Gerstenberg is supported by the comes on soon after, we might infer that both the TV and stereo are Center for Brains, Minds, and Machines (CBMM), funded by NSF STC tuned to the same signal, or start looking for another cause for the award CCF-1231216. Ralf Mayrhofer is supported by a grant from the stereo’s activation. In general, consistency between an observed Deutsche Forschungsgemeinschaft (Ma 6545/1-2) as part of the priority sequence of events and the predictions of different causal models program “New Frameworks of Rationality” (SPP 1516). David A. Lagnado provides evidence about the underlying relationships. This tempo- is supported by an Economic and Social Research Council UK grant (RES 062330004). ral information is unavailable to a purely contingency-based Correspondence concerning this article should be addressed to Neil R. learner who only uses the statistical associations between events. Bramley, Department of Psychology, New York University, 627, 60 5th Much of the extant research has focused on judgments of causal Avenue, New York, NY 10003. E-mail: [email protected] strength. Here, the causal structure is known and the question is 1880 TIME IN CAUSAL STRUCTURE LEARNING 1881 how strong or reliable the relationship between cause and effect is. constant but the timings of the events are either more consistent However, in everyday life, variables do not normally come neatly with a chain or a fork structure. packaged as causes and effects—one must first learn the causal structure relating the variables. A number of recent articles have Existing Research explored this more general problem. This work often compares Temporal information is relevant for causal inference in at least human judgments with the normative predictions of Pearl’s (2000) two respects. First, the temporal order of events places hard causal Bayesian network (CBN) framework (see also Spirtes, constraints on what could have caused what. Second, within these Glymour, & Scheines, 2000). The CBN framework supports prob- constraints event timings provide additional evidence for distin- abilistic inference on structured representations, and provides a guishing further between order-consistent causal structures. normative calculus for reasoning both about the consequences of observations as well as hypothetical (or counterfactual) interven- tions. Temporal Order By defining a language for expressing possible causal models, the Because effects cannot precede their causes, the order in CBN approach allows causal learning to be framed as a Bayesian which events occur is an important cue to causality. In line with model induction problem, where the learner uses observed evidence this, much of the human and animal learning literature is built to infer an underlying causal structure. From this perspective, around the notion that learners readily form associations from one people are generally found to be effective causal learners making event to the next (Pavlov, 1928; Skinner, 1938; Watson, 1913). inferences that are broadly normative (e.g., Griffiths & Tenen- Associative theories try to account for learning as an automatic baum, 2005; Lagnado & Sloman, 2002, 2004, 2006; Steyvers et pairwise association of stimuli. However, recent reanalysis of al., 2003) but also exhibiting the signatures of inductive biases and classical conditioning phenomena have suggested that learning is cognitive limitations (Bramley, Dayan, Griffiths, & Lagnado, often better understood as involving inferences about the causal 2017; Bramley, Dayan, & Lagnado, 2015; Bramley et al., 2015; structure that is responsible for the observed events (Courville, Coenen, Rehder, & Gureckis, 2015; Mayrhofer & Waldmann, 2006; Courville, Daw, Gordon, & Touretzky, 2004; Courville, 2016; Rehder, 2014; Rottman & Hastie, 2013, 2016). Daw, & Touretzky, 2002, 2006; Gershman & Niv, 2012). A shortcoming of the CBN framework is that Bayesian net- In the causal learning tradition, several articles have explored works do not naturally encode the temporal or spatial dimensions the role of temporal information in structure inference. Rottman of causal beliefs (cf. Gerstenberg & Tenenbaum, 2017). Conse- and Keil (2012) investigated how people infer the causal structure quently, many studies have focused on situations where informa- of multiple variables measured at discrete time points at which tion about time and space is nondiagnostic or abstracted away. variables may be subject to exogenous influences or interventions. When temporal cues have been pitted against statistical cues For example, suppose you are interested in an amoeba that occa- experimentally, judgments tended to be dominated by temporal sionally produces two different hormones. Suppose it is producing information (Burns & McCormack, 2009; Frosch, McCormack, neither hormone at time t Ϫ 1. If, at time t, you stimulate the Lagnado, & Burns, 2012; Lagnado & Sloman, 2004, 2006; production of one of the hormones and the other hormone is also Schlottmann, 1999). Furthermore, when researchers have tried to produced,
Details
-
File Typepdf
-
Upload Time-
-
Content LanguagesEnglish
-
Upload UserAnonymous/Not logged-in
-
File Pages31 Page
-
File Size-