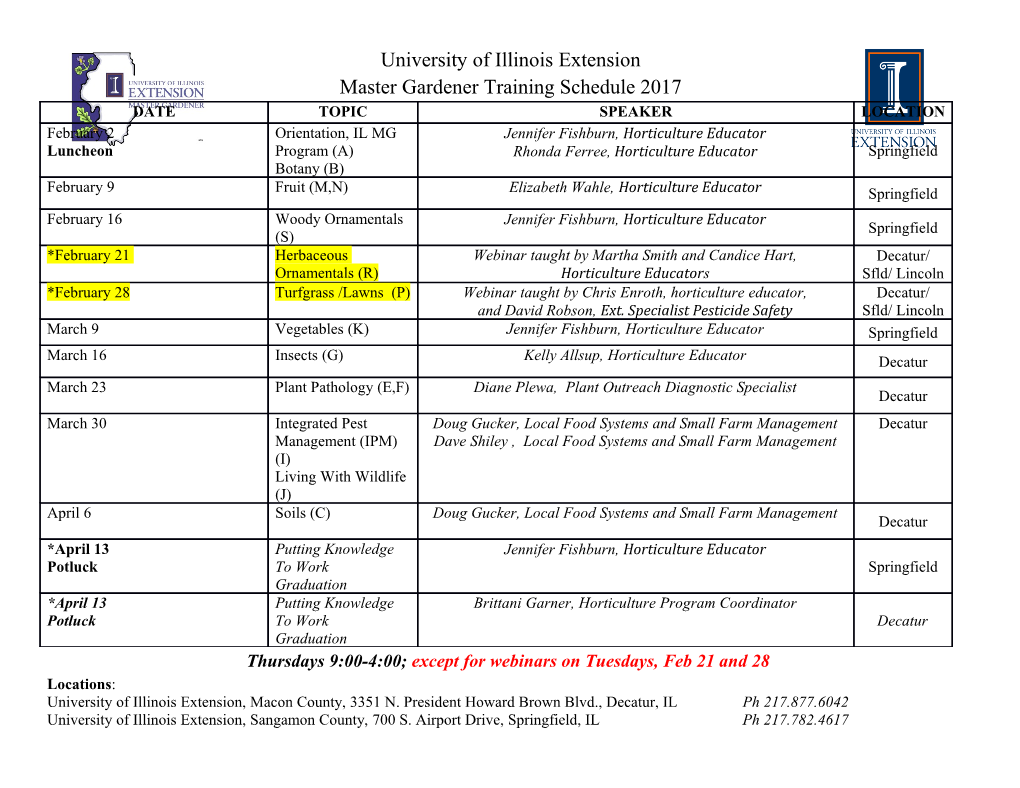
Time-Varying Vector Autoregressive Models with Structural Dynamic Factors Paolo Gorgi, Siem Jan Koopman, Julia Schaumburg http://sjkoopman.net Vrije Universiteit Amsterdam School of Business and Economics CREATES, Aarhus University ECB Workshop on \Advances in short-term forecasting" 29 September 2017 Introduction Econometric model Simulation Application Conclusion References The VAR model Consider the vector autoregressive (VAR) model of order p, the VAR(p) model: yt = Φ[1]yt−1 + ::: + Φ[p]yt−p + "t ;"t ∼ NID(0; H); t = p + 1;:::; T ; where Φ[i] is coefficient matrix, i = 1;:::; p, and H is variance matrix. For parameter estimation, forecasting, impulse response analysis, etc., we refer to Hamilton (1994) and Lutkepohl (2005), amongst many others. The VAR(p) model can be efficiently formulated as 0 0 0 yt = ΦYt−1:p + "t ; Φ = Φ[1];:::; Φ[p] ; Yt−1:p = yt−1;:::; yt−p We assume that the initial observation set fy1;:::; ypg is fixed and given. 2 / 32 Introduction Econometric model Simulation Application Conclusion References Motivation for time-varying parameters in VAR model In macroeconometrics, vector-autoregressive (VAR) models are often extended with time-varying parameters, we have yt = Φt Yt−1:p + "t ;"t ∼ NID(0; Ht ); t = p + 1;:::; T : Earlier literature: • Cogley/Sargent (2001 NBER): inflation-unemployment dynamics in changing monetary policy regimes • Primiceri (2005 RES): role of monetary policy for macroeconomic performance in the 1970s and 1980s • Canova/Ciccarelli (2004 JE, 2009 IER): Bayesian panel VAR models, multi-country analyses; • Hubrich/Tetlow (2015 JME): amplification and feedback effects between financial sector shocks and economy in crises vs. normal times • Prieto/Eickmeier/Marcellino (2016, JAE): role of financial shocks on macroeconomic variables during crisis 2008/09 3 / 32 Introduction Econometric model Simulation Application Conclusion References Estimation for TV-VAR models • The common approach to parameter estimation for VAR models with time-varying coefficients is based on Bayesian methods • We develop an alternative methodology based on dynamic factors for VAR coefficient matrices and score-driven dynamics for the variance matrices: • flexible modeling setup: it allows for wide variety of empirical specifications; • simple, transparent and fast implementation: least squares methods and Kalman filter; • relatively easy for estimation, impulse response, analysis and forecasting. • Our approach is explored in more generality by Delle Monache et al. (2016): adaptive state space models 4 / 32 Introduction Econometric model Simulation Application Conclusion References Outline • Introduction • Econometric Model • Simulations • Empirical application: Macro-financial linkages in the U.S. economy • Conclusion 5 / 32 Introduction Econometric model Simulation Application Conclusion References Time-varying autoregressive coefficient matrix • TV-VAR model : yt = Φt Yt−1:p + "t ;"t ∼ NID(0; Ht ); where we assume that sequence of variance matrices Hp+1;:::; HT is known and fixed, for the moment. • The time-varying VAR coefficient is a matrix function, c f f Φt = Φ(ft ) = Φ + Φ1f1;t + ··· + Φr fr;t ; where the unobserved r × 1 vector ft has dynamic specification ft+1 = 'ft + ηt ; ηt ∼ N(0; Ση); 0 where ' is r × r diagonal matrix of coefficients and Ση = Ir − '' . 6 / 32 Introduction Econometric model Simulation Application Conclusion References Linear Gaussian state space form c f f We have yt = Φt Yt−1:p + "t and Φt = Φ(ft ) = Φ + Φ1f1;t + ··· + Φr fr;t . c Definey ~t = yt − Φ Yt−1:p and consider the following equation equalities h f f i y~t = Φ1ft;1 + ::: + Φr ft;r Yt−1:p + "t h f f i = Φ1; ··· ; Φr ft ⊗ INp Yt−1:p + "t 0 h f f i = Yt−1:p ⊗ Φ1; ··· ; Φr vec ft ⊗ INp + "t 0 h f f i = Yt−1:p ⊗ Φ1; ··· ; Φr Q ft + "t : We let 0 h f f i Zt = Yt−1:p ⊗ Φ1; ··· ; Φr Q; to obtain the linear Gaussian state space form y~t = Zt ft + "t ; ft+1 = 'ft + ηt ; where the properties of the disturbances "t and ηt are discussed above. 7 / 32 Introduction Econometric model Simulation Application Conclusion References Kalman filter Prediction error is defined as vt = yt − E(yt jFt−1; ): • Ft−1 is set of all past information, including past observations; • is the parameter vector that collects all unknown coefficients in c f f Φ ,Φ1, :::,Φr , Hp+1, :::, HT , '; • when model is correct, the sequence fvp+1;:::; vT g is serially uncorrelated; • variance matrix of the prediction error is Ft = Var(vt jFt−1; ) = Var(vt ; ). For a given vector , the Kalman filter is given by 0 vt =y ~t − Zt at ; Ft = Zt Pt Zt + Ht ; 0 −1 Kt = 'Pt Zt Ft ; 0 at+1 = 'at + Kt vt ; Pt+1 = 'Pt (' − Kt Zt ) + Ση; with at = E(ft jFt−1; ) and variance matrix Pt = Var(ft − at jFt−1; ), for t = p + 1;:::; T . Loglikelihood function is T X N 1 1 0 −1 `( ) = `t ( ); `t ( ) = − log 2π − log jFt j − v F vt : 2 2 2 t t t=p+1 8 / 32 Introduction Econometric model Simulation Application Conclusion References Parameter estimation (1) For model c f f yt = Φt Yt−1:p + "t ; Φt = Φ(ft ) = Φ + Φ1f1;t + ··· + Φr fr;t ; ft+1 = 'ft + ηt ; c f parameter estimation concentrates on ',Φ and Φi , for i = 1;:::; r. MLE is maximisation of `( ) wrt , heavy task. Our strategy : c f Step 1: Use economic information (if available) to restrict entries of Φ and Φi . Step 2: Obtain estimates of Φc via least squares method on static VAR. f Step 3: Only place coefficients of ' and Φi in . Step 4: Estimate this by MLE using the Kalman filter. Least squares estimate of Φ from static VAR is consistent estimate of Φc . Notice that E(ft ) = 0. MLE is for a small dimension of . 9 / 32 Introduction Econometric model Simulation Application Conclusion References Time-varying variance matrix Each Kalman filter step at time t requires a value for Ht . We use score-driven approach of Creal et al. (2013) to let variance matrix Ht change recursively over time. ∗ σ ∗ We have N × 1 vector ft = vech(Ht ) with N = N(N + 1)=2 and dynamic specification σ σ ft+1 = ! + B ft + A st ; where ! is constant vector, A and B are square coefficient matrices and st is innovation vector. Distinguishing feature of score-driven model is definition of st as the scaled score σ vector of `t ≡ `t ( ) with respect to ft . We have st = St rt where St is scaling matrix and rt is gradient vector. 10 / 32 Introduction Econometric model Simulation Application Conclusion References Score-driven model for time-varying variance matrix The transpose of the gradient vector is given by @` @` @vec(F ) @` @vec(H ) r0 = t = t · t = t · t ; t σ 0 0 0 0 0 @ft @vec(Ft ) @vech(Ht ) @vec(Ft ) @vech(Ht ) 0 last equality holds since Ft = Zt Pt Zt + Ht and Zt does not depend on Ht and Pt is function of Hp+1;:::; Ht−1, but not Ht . @` 1 @vec(H ) t = vec(v v 0)0 − (vec(F ))0 F −1 ⊗ F −1 ; t = D ; 0 t t t t t 0 N @vec(Ft ) 2 @vech(Ht ) 2 ∗ where DN is the N × N duplication matrix, see Magnus and Neudecker (2007). It follows that 1 0 −1 −1 0 rt = D F ⊗ F vec(vt v ) − vec(Ft ) : 2 N t t t 11 / 32 Introduction Econometric model Simulation Application Conclusion References Score-driven model for time-varying variance matrix The inverse of the information matrix is taken as scaling matrix St for gradient vector. Information matrix: 0 It = E[rt rt jFt−1] 1 0 −1 −1 0 −1 −1 = D F ⊗ F Var vec(vt v ) − vec(Ft )jFt−1 F ⊗ F 4 N t t t t t 1 0 −1 −1 = D F ⊗ F (I 2 + C )D 4 N t t N N N 1 = D0 F −1 ⊗ F −1 D ; 2 N t t N 0 since Var[vec(vt vt ) − vec(Ft )jFt−1] = (IN2 + CN )(Ft ⊗ Ft ) and 2 2 (IN2 + CN )DN = 2 DN , where CN is the N × N commutation matrix. The inverse of the information matrix: −1 + + 0 It = 2Dn (Ft ⊗ Ft )DN ; + 0 −1 0 where DN = (DN DN ) DN is the elimination matrix for symmetric matrices. 12 / 32 Introduction Econometric model Simulation Application Conclusion References Score-driven model for time-varying variance matrix −1 −1 We set the scaling as St = It . The scaled score st = It rt becomes + + −1 −1 0 st = DN (Ft ⊗ Ft )DN DN (Ft ⊗ Ft )[vec(vt vt ) − vec(Ft )] + 0 = DN vec(vt vt ) − vec(Ft ) 0 = vech(vt vt ) − vech(Ft ): σ For the score-driven update of the variance factors in ft , we obtain σ 0 σ ft+1 = ! + A vech(vt vt ) − vech(Ft ) + B ft ; for t = p + 1;:::; T . The score updating function can easily be incorporated in the Kalman filter: 0 vt =y ~t − Zt at ; Ft = Zt Pt Zt + Ht ; 0 −1 Kt = 'Pt Zt Ft ; 0 at+1 = 'at + Kt vt ; Pt+1 = 'Pt (' − Kt Zt ) + Ση; 0 Ut = vt vt − Ft ; σ σ σ ft+1 = ! + Avech(Ut ) + Bft ; Ht+1 = unvech(ft+1): 13 / 32 Introduction Econometric model Simulation Application Conclusion References Direct updating for time-varying variance matrix σ We have vec(Ht ) = DN vech(Ht ) = DN ft and we obtain + 0 + vec(Ht+1) = DN ! + DN ADN [vec(vt vt ) − vec(Ft )] + DN BDN vec(Ht ); for t = p + 1;:::; T . + ∗ ∗ + ∗ ∗ When we specify DN ADN = A ⊗ A and DN BDN = B ⊗ B , we have ∗ 0 ∗ 0 ∗ ∗ 0 Ht+1 = Ω + A vt vt − Ft A + B Ht B ; with vech(Ω) = !.
Details
-
File Typepdf
-
Upload Time-
-
Content LanguagesEnglish
-
Upload UserAnonymous/Not logged-in
-
File Pages33 Page
-
File Size-