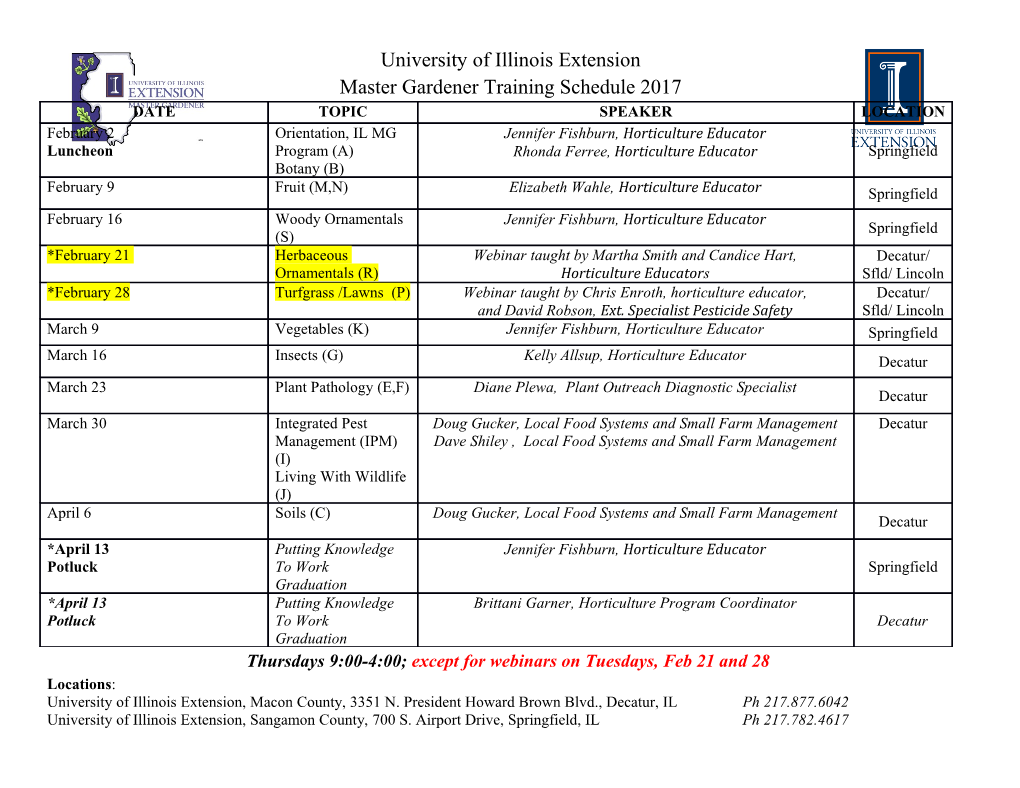
Journal of Modern Applied Statistical Methods Volume 10 | Issue 2 Article 8 11-1-2011 Probabilistic Inferences for the Sample Pearson Product Moment Correlation Jeffrey R. Harring University of Maryland, [email protected] John A. Wasko University of Maryland, [email protected] Follow this and additional works at: http://digitalcommons.wayne.edu/jmasm Part of the Applied Statistics Commons, Social and Behavioral Sciences Commons, and the Statistical Theory Commons Recommended Citation Harring, Jeffrey R. and Wasko, John A. (2011) "Probabilistic Inferences for the Sample Pearson Product Moment Correlation," Journal of Modern Applied Statistical Methods: Vol. 10 : Iss. 2 , Article 8. DOI: 10.22237/jmasm/1320120420 Available at: http://digitalcommons.wayne.edu/jmasm/vol10/iss2/8 This Regular Article is brought to you for free and open access by the Open Access Journals at DigitalCommons@WayneState. It has been accepted for inclusion in Journal of Modern Applied Statistical Methods by an authorized editor of DigitalCommons@WayneState. Journal of Modern Applied Statistical Methods Copyright © 2011 JMASM, Inc. November 2011, Vol. 10, No. 2, 476-493 1538 – 9472/11/$95.00 Probabilistic Inferences for the Sample Pearson Product Moment Correlation Jeffrey R. Harring John A. Wasko University of Maryland, College Park, MD Fisher’s correlation transformation is commonly used to draw inferences regarding the reliability of tests comprised of dichotomous or polytomous items. It is illustrated theoretically and empirically that omitting test length and difficulty results in inflated Type I error. An empirically unbiased correction is introduced within the transformation that is applicable under any test conditions. Key words: Correlation coefficients, measurement, test characteristics, reliability, parallel forms, test equivalency. Introduction correctly utilizing the Fisher transformation to It has been well-established that the sample provide accompanying probabilistic inferences correlation coefficient, r, is a biased estimator of (Fouladi, 2002). the population correlation coefficient, ρ , for The motivation for this study centers on normal populations, and this bias can be as much the failure of Fisher’s transformation to as 0.05 in absolute value under realistic research incorporate either test length or test difficulty conditions (Zimmerman, Zumbo & Williams, into confidence interval calculations. Without 2003). This difference may not be vital if the correction, test statistics and confidence research question is to simply ascertain whether intervals from utilizing the Fisher transformation a non-zero correlation exists. However, if the become increasingly imprecise ultimately focus is on a precise estimate of the magnitude resulting in inflated Type I error. To date, of a non-zero correlation in test and research has neither demonstrated the measurement procedures, then this discrepancy inefficiencies of utilizing this method, nor may be of concern. The Pearson product further advocated a test statistic inclusive of test moment correlation is still commonly used as an properties upon which to draw more accurate index of reliability, exampled with parallel test inferences about the population. In this article, forms (Coleman, 2001), test-retest conditions an empirical demonstration of systemic errors (Robinson-Kuropius, 2005), and inter-rater between the empirical distribution and the Fisher consistency (Lebreton, 2007). In such cases, transformation is presented which can be traced calculations use a total score comprised of to test properties of length and difficulty. Based dichotomous or polytomous items (Kline, 2005). on the results, a correction factor inclusive of With increasing frequency, practitioners test properties is introduced and examined using working in these contexts recognize sample a Monte Carlo simulation study to explore the estimates are insufficient and, therefore, are performance of the corrected statistic to the existing Fisher transformation. Methodology Jeffrey Harring is an Associate Professor in the Pearson Correlation Department of Measurement, Statistics and The Pearson’s correlation coefficient is Evaluation. Email him at: [email protected]. a measure of the strength of the linear relation John Wasko is a Colonel in the U.S. Army. between two continuous variables and is defined Email him at: [email protected]. as 476 HARRING & WASKO Cov(,)xy items, independent and identically distributed ρρ==(,)xy (1) σσ from any distribution, then their sum xy ii++.... + i = T ~ NJJ (μ ,22σ ) 12J 0 where x and y are vectors of scores of size is approximately normal for sufficiently large n,Cov(,)x y represents the population values of J. Although sufficiently large is not a covariance and σσ and are population xy quantifiable number, this requirement is standard deviations. Invariably researchers important given the need for a bivariate normal report a point estimate for reliability using the distribution upon which correlation inferences form are predicated (Quereshi, 1971). A rule of thumb of J exceeding 30 items has been suggested. Not s to be overlooked are the other requirements for ρρˆ ===(,xy ) r xy , s s use of the CLT. First is the requirement of x y independence. Conditional independence is assumed, where the likelihood a respondent where sxy, sx and sy are sample statistics answers an item correctly or incorrectly is corresponding to the population quantities in (1). independent of their response to any other test For test-retest reliability let, item. Second is the concept of identically distributed, where the collection of J items iid = μσ2 should all be dichotomously scored, i = [0,1] , or x (,,)xxNTTnxTxT1 ( , ) iid polytomously scored iR= [0,1,...., ] . = μσ2 y (,,)yyNTTnyTyT1 ( , ) Even if the total score is well approximated by a normal distribution, the total represent the total scores of n respondents score random variable is still discrete. In such administered the same test on different cases, when making probabilistic inferences occasions. For parallel forms, let with a continuous distribution with discrete data, a continuity correction is often applied (Devore, iid 2000). Recall that Pearson’s correlation is x = (xxN ,..., ) (μσ ,2 ) AAnxAxA1 designed for continuous random variable pairs iid y = (yyN ,..., ) (μσ ,2 ) that follow a bivariate normal distribution. B1 Bn yB yB Without a sufficient number of J items, the total score distributions depart from univariate represent the total scores of n respondents normality. administered different tests on different This condition is further exacerbated in occasions. By letting A and B represent two extremely easy or difficult shorter tests resulting raters scoring the same test for n respondents in highly skewed total scores; although this would constitute inter-rater reliability. Particular becomes less of an issue as test length increases, to test-retest and parallel forms, it is assumed test difficulty affects the rate of asymptotic that no learning has occurred as a result of the convergence to a normal distribution. Further, first exam or in the interim prior to the total score variable is not continuous, it is administration of the second exam. discrete. With all statistics, when underpinning assumptions are violated, the accuracy of the Central Limit Theorem Application results becomes increasingly questionable. Such The Pearson’s correlation coefficient inaccuracies are often commensurate with assumes total scores to be normally distributed; inflated Type I error rates. It is within this this is made possible by the central limit framework that the need for an item-type theorem (CLT) (see Hogg & Craig, 1995 for a correction encompassing test length and full description). Reviewing its application, if difficulty and a continuity correction may be ii12, ,.... iJ represent the scores for a test of J advocated. 477 PROBABALISTIC CORRELATION INFERENCE Fisher Transformation exp(2Z )− 1 exp(2Z )− 1 With (1−=α )%CI L , U . exp(2ZZ )++ 1 exp(2 ) 1 LU μ Σ (xy11 , ),( xy 2 , 2 ),....,( xynn , ) ~ N ( , ), Empirical Demonstration of Theoretical following a bivariate normal distribution, define Findings a random variable Z as To illustrate the need to account for the number of test items for asymptotic convergence to a normal distribution, two empirical 11+ r Z = ln , experiments are conducted. Conditions for the 21− r first simulation are a test length of J = 25 items, ρ a population correlation of = 0.8, approximated by the following normal administered to n = 100 respondents, where each distribution characterized by its mean and item is an independent dichotomous response variance with a p-value of 0.60. Conditions for the second simulation are 11+ ρ 1 J = 35, ρ = 0.7, n = 100, and a p-value of 0.70. ZN~ln, . −−ρ 21n 3 For each simulation, responses for J items for respondent i (i = 1, 2, …, n) were created Being normally distributed, these relations can according to a particular p-value representing a be used in the traditional construction of test. A second set of responses, representing a confidence intervals and hypothesis tests. The second test, were created such that each item was correlated with its first test equivalent transformation of the r random variable is called ρ the Fisher transformation; the immediate according to a particular . The item scores discussion centers on confidence intervals, were totaled for each test for each respondent, presentation of appropriate hypothesis tests are resulting in a paired set of total scores
Details
-
File Typepdf
-
Upload Time-
-
Content LanguagesEnglish
-
Upload UserAnonymous/Not logged-in
-
File Pages19 Page
-
File Size-