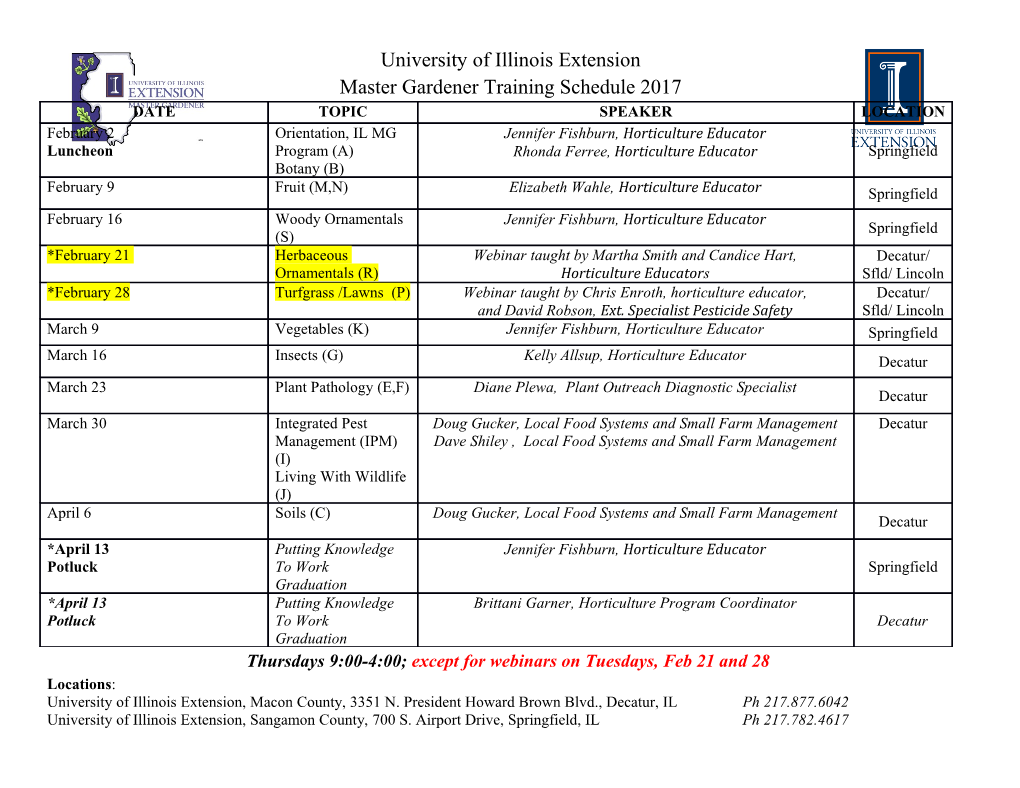
Maximum Likelihood Estimation of Dirichlet Distribution Parameters Jonathan Huang Abstract. Dirichlet distributions are commonly used as priors over propor- tional data. In this paper, I will introduce this distribution, discuss why it is useful, and compare implementations of 4 different methods for estimating its parameters from observed data. 1. Introduction The Dirichlet distribution is one that has often been turned to in Bayesian statistical inference as a convenient prior distribution to place over proportional data. To properly motivate its study, we will begin with a simple coin toss example, where the task will be to find a suitable distribution P which summarizes our beliefs about the probability that the toss will result in heads, based on all prior such experiments. 16 14 12 10 8 6 4 2 0 0 0.2 0.4 0.6 0.8 1 H/(H+T) Figure 1. A distribution over possible probabilities of obtaining heads We will want to convey several things via such a distribution. First, if we have an idea of what the odds of heads are, then we will want P to reflect this. For example, if we associate P with the experiment of flipping a penny, we would hope that P gives strong probability to 50-50 odds. Second, we will want the distribution to somehow reflect confidence by expressing how many coin flips we have witnessed 1 2 JONATHAN HUANG in the past, the idea being that the more coin flips one has seen, the more confident one is about how a coin must behave. In the case where we have never seen a coin flip experiment, then P should assign uniform probability to all odds. On the other hand, if we have seen many experiments before, then we will have a good idea of what the odds are, and P will be strongly peaked at this value. Figure 1 shows one possibility for P where probability density is plotted against probability of flipping heads. Here, the prior belief is fairly certain that the odds of obtaining heads is about 50-50. The form of the distribution for this particular graph is given by: p(x) ∝ x199 (1 − x)199 and is an example of the so-called beta distribution. 2. The Dirichlet Distribution This section will show that a generalization of the beta distribution to higher dimensions leads to the Dirichlet. In the coin toss example, we only considered the odds of getting heads (or tails) and placed a distribution on these odds. An m-dimensional Dirichlet will be defined as a distribution over multinomials, which are m-tuples p = (p1,...,pm) that sum to unity. For the two dimensional case, this is just pairs (H,T ) such that H + T = 1. The space of all m-dimensional multinomials is an (m − 1)-simplex by definition, and so the Dirichlet distribution can also be thought of as a distribution over a simplex. Algebraically, the distribution is given by 1 − Dir(p|α ,...,α )= pαk 1 1 m Z k k Ém Y k=1 Γ(αk ) 1 where Z = Èm is a normalization factor. There are m parameters αk Γ( k=1 αk) which are assumed to be positive. Figure 2 plots several examples of a three- dimensional Dirichlet. Yet another way to think about the Dirichlet distribution is in terms of mea- sures. Essentially, a Dirichlet is a measure over the space of all measures over a set of m elements. This is interesting because the idea can be extended in a rig- orous way to the concept of Dirichlet processes, which are measures over measures on more general sets. The Dirichlet process is, in some sense, an infinite dimen- sional version of the Dirichlet distribution. This is a useful prior to put over mixing weights of a Gaussian mixture model and is used for automatically picking out the number of necessary clusters as opposed to the approach of trying to fit the data several times to different numbers of clusters to find the best number [4]. 2.1. An Intuitive Reparameterization. A simple reparameterization of the Dirichlet is given by setting: m s = αk Xk=1 1 Ê ∞ x−1 −t Γ(x) denotes the Gamma function and is defined to be: 0 t e dt. Integrating this parts gives the functional definition: Γ(x +1) = xΓ(x). Since Γ(1) = 1, we see that this function satsifies Γ(n +1) = n! for n ∈ N and is a generalization of the factorial to the real line. MAXIMUM LIKELIHOOD ESTIMATION OF DIRICHLET DISTRIBUTION PARAMETERS 3 Figure 2 and α α m = 1 ,..., m s s The vector m sums to unity and hence is a point on the simplex. It turns out to be exactly the mean of the Dirichlet distribution. s is commonly referred to as the precision of the Dirichlet (and sometimes as the concentration parameter) and as its name implies, controls how concentrated the distribution is around its mean. For example, on the right hand side of Figure 2, s is small and hence yields a diffuse distribution, whereas the center plot on Figure 2 has a large s and is hence concentrated tightly about the mean. As will be discussed later, it is sometimes useful to estimate mean independently of precision or vice-versa. 2.2. The Exponential Family. It is illuminating to study the Dirichlet as a special case of a larger class of distributions called the exponential family, which is defined to be all distributions which can be written as p(x|η)= h(x)exp{ηT T (x) − A(η)} where η is called the natural or canonical parameter, T (x) the sufficient statistic, and A(η) the log normalizer. Some common distributions which belong to this family are the Gaussian, Bernoulli and Multinomial distributions. It is easy to see that the Dirichlet also takes this form by writing: h(x) = 1 η = α − 1 T (x) = log p A(η) = N log Γ(αk) − logΓ αk !! Xk Xk Besides being well understood, there are several reasons why distributions from this family are commonly employed in statistics. As shown by the Pitman- Koopman-Darmois theorem, it is only in this family that the dimension of the 4 JONATHAN HUANG sufficient statistic is bounded even as the number of samples goes to infinity. This leads to efficient point estimation methods. Bayesians are particularly indebted to the exponential family due to the fact that if a likelihood function belongs to it, then a conjugate prior must exist. 2 Existence of such a prior simplifies computations immensely and the lack of one often requires one to resort to numerical techniques for estimating a posterior. A final noteworthy point is that A(η) is the cumulant generating function for the sufficient statistic, so in particular, A0(η) is the expectation, and A00(η) is the variance. This implies that A is convex, which further implies that the log-likelihood function of data drawn from these distributions is convex in η. 2.3. The Dirichlet as a Prior. The most common reason for using a Dirich- let distribution is as a prior on the parameters to a multinomial distribution. The multinomial distribution also happens to be a member of the exponential family, and accordingly, has an associated conjugate prior. The multinomial distribution is a generalization of the binomial distribution and is defined over m-tuples of “counts”, which are just nonnegative integers: m ( k xk)! xk Mult(x|θ)= m θk k=1(xk!) P kY=1 where the parameters θ are probabilitiesQ of falling into one of m classes and hence θ is a point on an (m − 1)-simplex. It is not difficult to explicitly show that the Multinomial and Dirichlet distributions form a conjugate prior pair: p(x|θ)p(θ) = Mult(x|θ)Dir(θ|α) m m xk αk −1 ∼ θk θk kY=1 kY=1 − ∼ θxk +αk 1 Yk = Dir(x + α) The last line follows by observing that the posterior is a distribution, so when normalized, must yield an actual Dirichlet. What is very nice about this expression is that it mathematically formalizes the intuition that the parameters to the prior, α, can be thought of as pseudocounts. Going back to the two dimensional case, we see that α encodes a tally of the results of all prior coin flips. 3. Estimating Parameters Given a set of observed multinomial data, D = {p1, p2,..., pN }, the parame- ters for a Dirichlet distribution can be estimated by maximizing the log-likelihood 2A conjugate prior for a likelihood function is defined to be a prior for which posterior and prior are of the same distribution type. MAXIMUM LIKELIHOOD ESTIMATION OF DIRICHLET DISTRIBUTION PARAMETERS 5 function of the data, which is given by: F (α) = log p(D|α) = log p(pi|α) i Y Γ ( α ) − = log k k pαk 1 Γ(α ) ik i k k Y P Yk Q = N logΓ αk − log Γ (αk)+ (αk − 1)logp ˆk ! ! Xk Xk Xk 1 where logp ˆk = N i log pik and are the observed sufficient statistics. P Figure 3. Examples of log-likelihood functions of a three dimen- sional Dirichlet The following sections will provide an overview of several methods for numer- ically maximizing this objective function, F as there is no closed form solution to this. As discussed above, they will all use the fact that the log-likelihood is convex in α to guarantee a unique optimum. 3.1. Gradient Ascent. The first method to try is Gradient Ascent, which iteratively steps along positive gradient directions of F until convergence.
Details
-
File Typepdf
-
Upload Time-
-
Content LanguagesEnglish
-
Upload UserAnonymous/Not logged-in
-
File Pages9 Page
-
File Size-