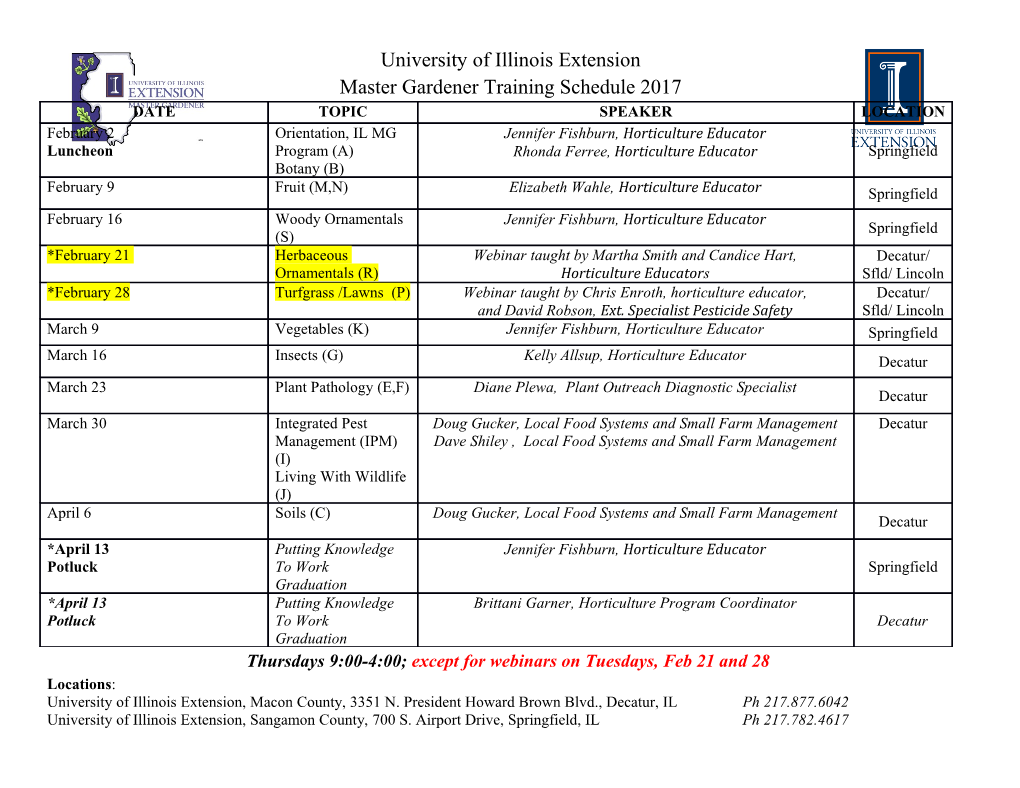
Cheminformatics in Drug Discovery, an Industrial Perspective Hongming Chen, Thierry Kogej, Ola Engkvist Hit Discovery, Discovery Sciences, Astrazeneca R&D Gothenburg Drug Discovery Today An industry perspective of Today’s Challenge We need to become better, faster & cheaper Source: Paul et al., Nat. Rev. Drug Disc. (2010) 9:March Source: PhRMA profile 2016 2 IMED Biotech Unit I Discovery Sciences Cheminformatics @ AstraZeneca • HTS work-up • Library design • Virtual screening • Machine learning & AI 3 High Throughput Screening From Millions to just a few Low cost/compound High cost/compound Slide modified from Mark Wigglesworth, AZ, with permission HTS Analysis: Clustering analysis iHAT: An Spotfire add-in for HTS Analysis Early days • Heavily dependent on computational chemistry resources • Leverage the powerful visualization function of • Linux, scripts, static workflows, data in flat files Spotfire • Cutting, pasting and reformatting between • Annotation of compounds with in-house applications experimental and predicted data • Difficult to revisit or take over an analysis from a • Data integration from multiple sources colleague • Clustering of compounds • Time-consuming • Visualization and manipulation of cluster tree • NN search 5 iHAT: Clustering and Reclustering 6 Library design @ AstraZeneca • Diversity library is generally out of fashion • Focused library fit for specific project need • DNA encoded libraries become popular, but analysis is challenging, >60M to 8B library sizes Currently, use classical library design method to reduce to 50M preferred AZ library size Vendors reagents Only AZ reagents Fsp3 7 Definition of VS • Virtual screening refers to any in-silico techniques used to search large compound databases (available chemicals or virtual libraries) to select a smaller number for biological testing • Virtual screening can be used to − Select compounds for screening from in-house databases − Choose compounds to purchase from external suppliers − Select compounds from virtual libraries to be synthesized • The technique applied depends on the amount of information available about the particular disease target and the desired outcome 8 VS methods 3D Structure of Target Unknown Known Ligand-based methods structure-based methods Lots of actives and Actives known inactives known Co-crystallized Aligned Protein structure conformation ligand conf. QSAR models 2D ligand 3D ligand Binding pocket •Substructure search; •Shape similarity search •Docking and scoring •Fingerprint-based •Pharmacophore mapping •Pharmacophore mapping similarity search •core replacement •shape similarity •Scaffold hopping •filter, improve hit rate •Expand SAR •filter, improve hit rate •Improve affinity VS example IC50 (NMR) 20uM • Identification of sPLA2X inhibitors using ligand and structure based virtual screening 10 Virtual screening platform @ AZ Iterative virtual screening workflow VS chemical 15 Chemical space space 10 Sub-sets 108-11 Theoretical chemical space (1060) Ligand Primary VS 108-11 AZ-Virtual space 1015 Diverse: > 4000 libraries Virt. hits 106 Virt. NN 106-8 Synthesizable: historic Known existing AZ-reactions compounds Searchable in subsets of 108 AZ Structure, Secondary VS 8-11 106 10 Ligand, 106 Properties, Novelty MJ Vainio J. Chem. Inf. Model., 2012, 52 (7), pp 1777–1786. 20-30 virt. lib.s Reactions, reagents 1-3 libs./50-100 11 IMED Biotech Unit I Discovery Sciences cpd. ordered Computational strategy Few libraries are enriched specifically to the query 3D similarity search project Structure based workup 105 108 Selection of libraries Representative subset (3D similarity and (as large as possible) structure based workup) 12 IMED Biotech Unit I Discovery Sciences AI & Machine Learning Today Context, Definition & Advances Source: http://www.geeksforgeeks.org/artificial- intelligence-an-introduction/ 13 IMED Biotech Unit I Discovery Sciences Source: http://hduongtrong.github.io The rise of deep learning in drug discovery • Deep learning technologies have been adopted in drug discovery • Various forms of NN have been applied so far 14 De novo molecular generation with deep learning has developed very rapidly 15 Deep learning @ AstraZeneca: Vision • Creating an integrate AI platform to impact drug discovery projects Data AI design platform foundation Augmented design AI reaction platform Formalize chemical Autonomous intuition design Automatic make and test (iLab) “Learn from Automatic everything” design Integration Segler M.H.S. et al. Neural‐Symbolic Machine Learning for Retrosynthesis and Reaction Prediction, Chemistry, 2017, 23(25), 5966-5971 Segler M.H.S. et al. Planning chemical syntheses with deep neural networks and symbolic AI, Nature, 2018, 555, 604-610 16 Deep learning @ AZ: De Novo Molecular Augmented Design Platform (REINVENT) Generation of novel Reinforcement learning to generate chemical space project relevant compounds Agent model AZ+ Pub Iterations Desirability function Σ IC50, LogP, Novelty etc. Prior model 17 Iterations of design and compound synthesis Deep learning at AstraZeneca: Reaction informatics • First steps, building: – World-class Reaction Knowledge Base – On our work (past collaboration with M. Segler) MedChem ELN PharmSci ELN Predictive chemical AZ ChemistryConnect reaction models Flat files ReaxysFlatFlat filesfiles Make iLab AZ Reaction Connect ~20mill reactions Design DMTA Test FlatPatentFlatFlat filesfiles files data Analyse Becoming FASTER with AI Through unsupervised learning for hit identification 0 4 0 2 y 0 - e n s t 0 2 - 0 4 - -40 -20 0 20 40 60 Manifold Deep CNN tsne-x Deep CNN Learning autoencoder classifier (t-SNE) 19 Becoming CHEAPER with ML/AI By only conducting experiments when needed ) Extract features Build/Apply ML model (signature descriptor) (Mondrian TCP on SVM) (class (class Don’tsynthesize value value - p Don’t test p-value (class ) 20 Becoming FASTER and CHEAPER with AI AI augmented de novo molecule design RNN + Reinforcement learning 21 AZ’s first DMTA automation platform Reformatting • First protype built during 2017 Make • All DMTA steps fully integrated • Suited for 100s of uninterrupted DMTA cycles. Test Purification & ML/AI module is integrated. Analysis Screening data • Cycle times of ca. 2h analysis & Compound design • Successfully applied in ongoing research project 22 Conclusions • Cheminformatics is widely applied in Pharmaceutical industry • Cheminformatics includes various aspects across different disciplines • Adoption of machine learning and AI technologies will help Cheminformatics to better fit current and future research needs 23 Acknowledgement • Marcus Olivercrona • EU Fundings • Thomas Blaschke • Isabella Feierberg • Christophe Grebner • Erik Malmerberg • Christian Tyrchan • Garry Pairaudeau • Clive Green • Lars Carlsson • Peter Varkonyi • Michael Kossenjans 24 Confidentiality Notice This file is private and may contain confidential and proprietary information. If you have received this file in error, please notify us and remove it from your system and note that you must not copy, distribute or take any action in reliance on it. Any unauthorized use or disclosure of the contents of this file is not permitted and may be unlawful. AstraZeneca PLC, 1 Francis Crick Avenue, Cambridge Biomedical Campus, Cambridge, CB2 0AA, UK, T: +44(0)203 749 5000, www.astrazeneca.com 25.
Details
-
File Typepdf
-
Upload Time-
-
Content LanguagesEnglish
-
Upload UserAnonymous/Not logged-in
-
File Pages25 Page
-
File Size-