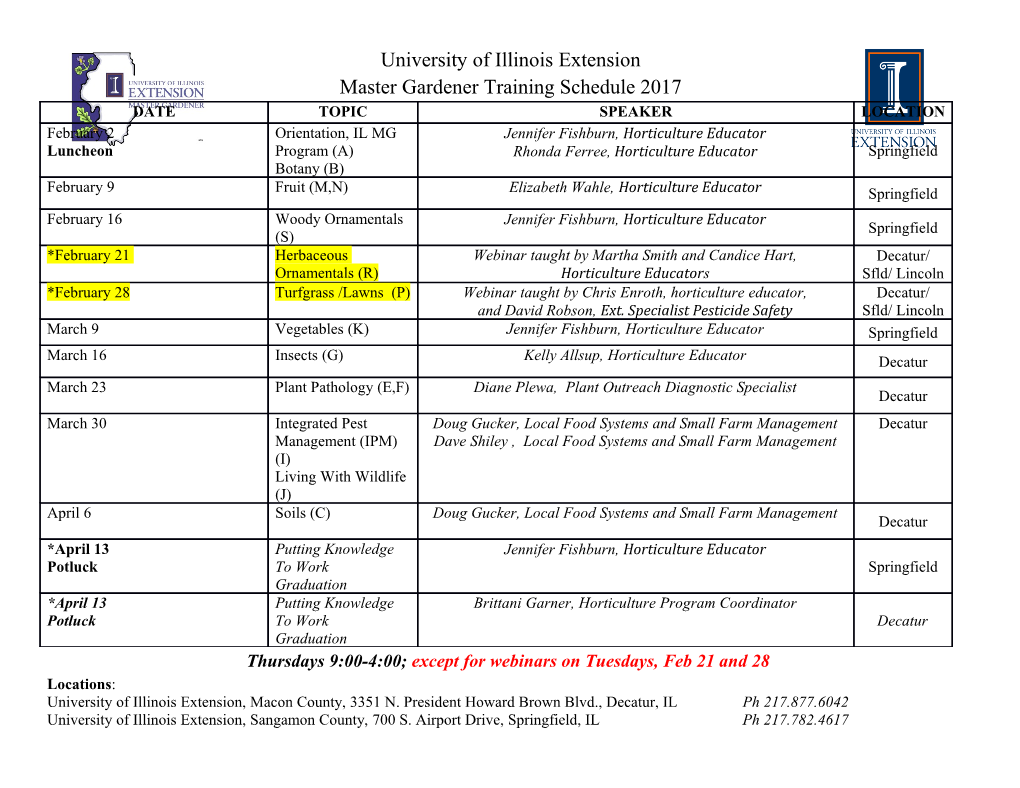
OpenMS { A framework for computational mass spectrometry Dissertation der Fakult¨atf¨urInformations- und Kognitionswissenschaften der Eberhard-Karls-Universit¨atT¨ubingen zur Erlangung des Grades eines Doktors der Naturwissenschaften (Dr. rer. nat.) vorgelegt von Dipl.-Inform. Marc Sturm aus Saarbr¨ucken T¨ubingen 2010 Tag der m¨undlichen Qualifikation: 07.07.2010 Dekan: Prof. Dr. Oliver Kohlbacher 1. Berichterstatter: Prof. Dr. Oliver Kohlbacher 2. Berichterstatter: Prof. Dr. Knut Reinert Acknowledgments I am tremendously thankful to Oliver Kohlbacher, who aroused my interest in the field of computational proteomics and gave me the opportunity to write this thesis. Our discussions where always fruitful|no matter if scientific or technical. Furthermore, he provided an enjoyable working environment for me and all the other staff of the working group. OpenMS would not have been possible without the joint effort of many people. My thanks go to all core developers and students who contributed to OpenMS and suffered from the pedantic testing rules. I especially thank Eva Lange, Andreas Bertsch, Chris Bielow and Clemens Gr¨oplfor the tight cooperation and nice evenings together. Of course, I'm especially grateful to my parents and family for their support through- out my whole life. Finally, I thank Bettina for her patience and understanding while I wrote this thesis. iii Abstract Mass spectrometry coupled to liquid chromatography (LC-MS) is an analytical technique becoming increasingly popular in biomedical research. Especially in high-throughput proteomics and metabolomics mass spectrometry is widely used because it provides both qualitative and quantitative information about analytes. The standard protocol is that complex analyte mixtures are first separated in liquid chromatography and then analyzed using mass spectrometry. Finally, computational tools extract all relevant information from the large amounts of data produced. This thesis aims at improving computational analysis of LC-MS data|we present two novel computational methods and a software framework for the development of LC-MS data analysis tools. In the first part of this thesis we present a quantitation algorithm for peptide signals in isotope-resolved LC-MS data. Exact quantitation of all peptide signals (so-called peptide features) is an essential step in most LC-MS data analysis pipelines. Our algorithm detects and quantifies peptide features in centroided peak maps using a multi-phase approach: First, putative feature centroid peaks, so-called seeds, are determined based on signal properties that are typical for peptide features. In the second phase, the seeds are extended to feature regions, which are compared to a theoretical feature model in the third phase. Features that show a high correlation between measured data and the theoretical model are added to a feature candidate list. In a last phase, contradicting feature candidates are detected and contradictions are resolved. In a comparative study, we show that our algorithm outperforms several state-of-the-art algorithms, especially on complex datasets with many overlapping peaks. The second part of this thesis introduces a novel machine learning approach for model- ing chromatographic retention of DNA in ion-pair reverse-phase liquid chromatography. The retention time of DNA is of interest for many biological applications, e.g., for quality control of DNA synthesis and DNA amplification. Most existing models use only the base composition to model chromatographic retention of DNA. Our model complements the base composition with secondary structure information to improve the prediction performance. A second difference to previous models is the use of a support vector re- gression model instead of simple linear or logarithmic models. In a thorough evaluation, we show that these changes significantly improve the prediction performance, especially at temperatures below 60◦C. As a by-product, our approach allows the creation of a temperature-independent model, which can predict DNA retention times not only for a fixed temperature, but for all temperatures within the temperature range of the training data. Finally, we present OpenMS { a framework for computational mass spectrometry. OpenMS provides data structures and algorithms for the rapid development of mass spectrometry data analysis software. Rapid software prototyping is especially important in this area of research because both instrumentation and experimental procedures are quickly evolving. Thus, new analysis tools have to be developed frequently. OpenMS facilitates software development for mass spectrometry by providing a rich functionality ranging from support for many file formats, over customizable data structures and data visualization, to sophisticated algorithms for all major data analysis steps. The peptide feature quantitation algorithm presented in the first part of this thesis is one of many algorithms provided by OpenMS. We demonstrate the benefits of using OpenMS by the development of TOPP { The OpenMS Proteomics Pipeline. TOPP is a collection of command line tools which each perform one atomic data analysis step|typically one of the OpenMS data analysis algo- iv rithms. The individual TOPP tools are used as building blocks for customized analysis pipelines. This kind of flexibility and a graphical user interface for the visual creation of analysis pipelines make TOPP a versatile instrument for LC-MS data analysis. Kurzzusammenfassung Die Kopplung von Massenspektrometrie (MS) und Fl¨ussigchromatographie (LC) gewinnt immer mehr Bedeutung als analytische Technik in der biomedizinischen Forschung. Vor allem in der Hochdurchsatzproteomik und -metabolomik ist Massenspektrometrie weit verbreitet, weil sie sowohl qualitative als auch quantitative Information ¨uber Analyten liefert. Komplexe Stoffmischungen werden normalerweise mit Fl¨ussigchromatographie aufgetrennt bevor sie mittels Massenspektrometrie analysiert werden. Danach werden alle relevanten Informationen mithilfe spezieller Computerprogramme extrahiert, da die produzierten Datenmengen sehr groß sind. Diese Arbeit hat das Ziel die computer- gest¨utzteAnalyse von LC-MS Daten zu verbessern. Wir stellen zwei neue Methoden zur Datenanalyse und eine Softwarebibliothek zur Entwicklung von Analyseprogrammen vor. Der erste Teil dieser Arbeit besch¨aftigtsich mit einem Algorithmus zur Quantifizierung von Peptidsignalen (sogenannten Peptid-Features) in LC-MS Daten. Die exakte Quan- tifizierung aller Peptid-Features ist ein wichtiger Verarbeitungsschritt der meisten LC- MS Analyse-Pipelines. Unser Algorithmus detektiert und quantifiziert Peptide-Features in Peakdaten durch ein mehrstufiges Verfahren: Zuerst werden potenzielle Signalmit- telpunkte gesucht anhand der f¨urPeptidsignale typischen Eigenschaften. In einem zweiten Schritt werden die gefundenen Mittelpunkte zu Signalregionen vergr¨oßert,die im dritten Schritt mit einem theoretischen zweidimensionalen Modell verglichen wer- den. Signalregionen die eine hohe Ubereinstimmung¨ zwischen Messdaten und Modell aufweisen werden dann in eine Kandidatenliste von potenziellen Peptid-Features einge- tragen. Im letzten Schritt werden Widerspr¨uche in der Kandidatenliste gesucht und diese behoben. In einer Vergleichsstudie auf komplexen Daten mit vielen ¨uberlappenden Signalen konnten wir zeigen, dass unser Algorithmus mehreren modernen Algorithmen ¨uberlegen ist. Im zweiten Teil der Arbeit stellen wir einen neues maschinelles Lernverfahren zur Vorhersage von DNA Retentionszeiten in der Umkehrphasen-Chromatographie vor. Die Retentionszeit von DNA ist f¨urviele biologische Anwendungen von Interesse, zum Beispiel f¨urdie Qualit¨atskontrolle der DNA-Synthese und der DNA-Amplifikation. Die meisten existierenden Verfahren benutzen nur die Basenzusammensetzung der DNA um die Re- tentionszeit zu modellieren. Unser Modell beruht auch auf der Basenzusammensetzung, bezieht aber Sekund¨arstrukturinformationein, um die Vorhersageleistung zu verbessern. Ein weiterer Unterschied zu bisherigen Methoden ist die Verwendung von Support Vector Regression anstelle einfacher linearer und logarithmischer Modelle. In einer Vergleichs- studie zeigen wir, dass diese Neuerungen die Vorhersageleistung signifikant erh¨ohen, vor allem bei Temperaturen unter 60◦C. Außerdem erlaubt unsere Methode die Erstellung temperaturunabh¨angiger Modelle, die Retentionszeiten nicht nur f¨ureine feste Temper- atur, sondern f¨urden gesamten von den Trainingsdaten abgedeckt Temperaturbereich vorhersagen k¨onnen. Schließlich stellen wir OpenMS, eine Bibliothek zur Entwicklung von Software f¨ur die Massenspektrometrie, vor. OpenMS bietet alle erforderliche Datenstrukturen und viele Algorithmen zur schnellen Entwicklung von Analysesoftware. Die schnelle Entwick- lung von Softwareprototypen ist gerade in der Massenspektrometrie besonders wichtig, da sowohl die Instrumente als auch die experimentellen Protokolle sehr schnell weiter- v entwickelt werden. Daher m¨ussenregelm¨aßigneue Softwarel¨osungenzur Analyse der Daten entwickelt werden. OpenMS stellt eine umfangreiche Infrastruktur zur Verf¨ugung und vereinfacht so die Entwicklung dieser Analysesoftware. Die Funktionalit¨atvon OpenMS reicht von der Unterst¨utzungf¨urweit verbreitete Dateiformate, ¨uber anpass- bare Datenstrukturen and Datenvisualisierung, bis hin zu modernen Analysealgorithmen f¨uralle Hauptanalyseschritte. Die Vorteile die sich aus der Benutzung von OpenMS ergeben zeigen wir anhand der Entwicklung von TOPP { The OpenMS Proteomics Pipeline. TOPP ist eine
Details
-
File Typepdf
-
Upload Time-
-
Content LanguagesEnglish
-
Upload UserAnonymous/Not logged-in
-
File Pages149 Page
-
File Size-