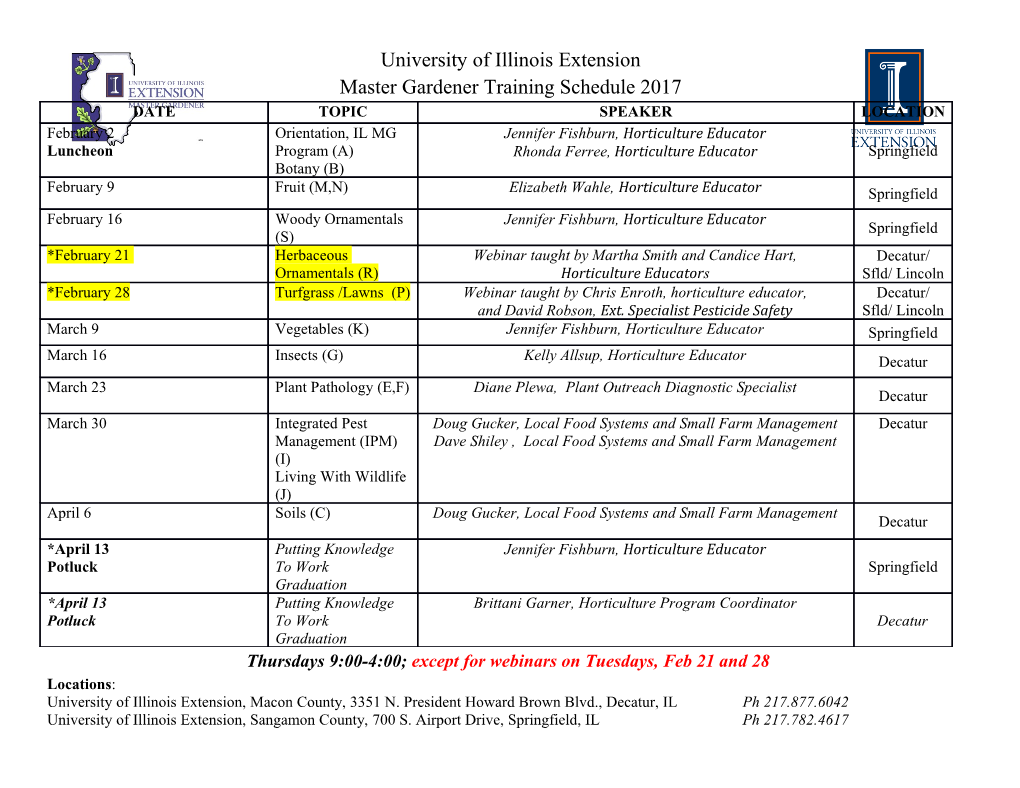
Suspect and Non-Target Analysis of polar organic compounds in biota using LC-HRMS Pablo Gago-Ferrero Contact: [email protected] Introduction Emerging Pollutants (EPs) Pharmaceuticals Personal care products Flame retardants Food additives Disinfection by-products Pesticides + Metabolites & Transformation Products (TPs) aquatic environment & Biota 2 Introduction Challenges in the analysis of organic contaminants in biota Sample preparation (lipid content, trace level, sample size) Thousands of organic contaminants with very different physicochemical properties Investigation of new (unknown) contaminants potentially dangerous for the ecosystems (and human health) Metabolites 3 Target screening Target screening • Known EP Well-established analytical (quantitative) • Reference standards methods for many priority contaminants available Good limits of detection • Unequivocal identification High accuracy Reliable quantification 4 Why Suspect / non-target? Target screening is biased due to preselection of substances Most organic constitutes of environmental samples are not identified! Potential chemical stressors may be omitted Most of the labs analyse the same substances Reference standards are necessary for all compounds 5 Suspect & non-target: Where to put our efforts? Thousands of chromatographic peaks in one sample Impossible & Pointless to identify all of them Smart use Suspect & Non target strategies Define Research question &Prioritization Suspect screening Classical micropollutants for which their presence in biota has been widely assessed and target methodologies are easily available Very long list of compounds without a clear purpose Metabolites of previously detected parent compounds Regulatory data base & market data Compounds potentially present due to close industrial activity Non-Target screening: Prioritization strategies Intensity-based prioritization Long time series prioritization (prioritized features whose intensities varied substantially over the time course of the sampling campaign) Geographical series prioritization Effect-directed analysis (EDA), for identifying predominant toxicants in complex environmental mixtures combining effect testing and fractionation In source fragmentation flagging Metabolites Case study I: Metabolites of venlafaxine in Biota Objective L.E. Santos • Suspect screening analysis for the assessment of the metabolization of venlafaxine by fish (Argyrosomus regius) • Fish exposure at 20 µg L-1 of VLF via water for 28 days Sample analysis in a HPLC-LTQ-Orbitrap Velos in Data Dependant •Sample treatment using ultrasound assisted extraction Acquisition mode (DDA) with fragmentation of the most intense ions Case study I: Metabolites of venlafaxine in Biota Software data processing using Compound Discoverer Identification of tentative VLF metabolites via compound 2.0 connected to Mass Frontier 7.0 software. exact mass and MS/MS ion fragments. • Selection of 5 (phase I) and 10 (phase II) possible chemical transformations. Suspect list (Compound Prediction) • Max. number of combined transformations: 3 { • Nº compounds predicted: 1527 Methods: Suspect screening performance Confirmation with corresponding reference standard Modified from Gago-Ferrero et al. 2015; Env. Sci. Tech 49(20) 1433 11 Case study I: Metabolites of venlafaxine in Biota Proposed metabolic pathway of venlafaxine in fish tissues • 10 VLF metabolites were tentatively identified • 2 VLF Phase II metabolites were identified in the fish liver * * * ** • Oxidation, demethylation and conjugation are the main reactions * involved • All VLF metabolites were identified in liver (*), except MET275 ** ** • 7 VLF metabolites were identified in brain (*) * * * * * * Confirmed Case study II: Ecometabolomics in fluvial biofilm Objectives A. Serra-Compte • Identify biomarkers of drought stress and pharmaceutical exposure Biofilm Sensitive to river changes • Identify metabolic pathways affected by stress Rapid interaction with dissolved • Relate metabolome changes with changes in the biofilm structural substances parameters Short life cycle & Bioaccumulation capacity Pharmaceutical exposure Drought Ecometabolomics Serra-Compte et al. 2018; Sci. Tot. Environ 618: 1382-1388 Case study II: Ecometabolomics in fluvial biofilm Biofilm exposure experiment in Experimental Streams Facility Conc Compound (ng/L) - Pharmaceutical exposure (P) Ibuprofen 404 - Dry period (D) (7 days) Diclofenac 366 Carbamazepine 124 - Dry period + pharm exposure (D+P) Sulfamethoxazole 699 - Control (C) Erithromycin 169 Metoprolol 1845 Atenolol 117 Gemfibrozil 140 Hydrochlorothiazide 1135 Analytical Workflow LC-LTQ Orbitrap Velos Acquisition: 100-700 m/z, Data Treatment: Extraction: PLE SIEVE software: deconvolution and allingment Biofilm Extracts Clean-up: SPE Case study II: Ecometabolomics in fluvial biofilm Metabolomics workflow First injection: LC-HRMS Orbitrap analysis Full scan mode •Chemometrics analysis •Background subtraction •Prioritization (databases: Human •Component detection •Identification of features that Metabolome DataBase (HMDB), contributed to the separation between chemspider, Plant metabolomics) • Peak alignment groups 67 potential metabolites 1978 features ( + and – ESI) 664 features ( + and – ESI) Second injection: LC-HRMS Orbitrap analysis MSMS (data dependent) • • Tentative identification •Confirmation Metabolic routes altered based on the metabolites identified 9 Metabolites identified 6 confirmed with standard •(KEGG databases) Case study II: Ecometabolomics in fluvial biofilm Biofilm exposure experiment in Experimental Streams Facility 42 days exposure experiment - Pharmaceutical exposure (P) - Dry period (D) (7 days) - Dry period + pharm exposure (D+P) - Control (C) Principal component analysis (PCA) of significant metabolites of biofilm exposed to the treatments Serra-Compte et al. 2018; Sci. Tot. Environ 618: 1382-1388 Case study II: Ecometabolomics in fluvial biofilm Potential chemical markers of stress Tentative Compoud Family Drought PhACs Drought + PhACs biomarkers Saturated Behenic acid ↑ ↓ – fatty acid Saturated Lignoceric acid ↑ ↓ – fatty acid Saturated Palmitic acid – ↓ – fatty acid Unsaturated Stearidonic acid ↑ – ↑ Potential biomarker of fatty acid drought stress Unsaturated Alpha linolenic acid ↑ – - fatty acid Unsaturated Palmitoleic acid ↓ – ↓ fatty acid LPA (0:0/16:0) Glycerophospholipid – ↓ ↓ Potential biomarker of pharm 16-Oxohexadecanoic Oxo fatty acid ↑ – ↑ stress Azelaic Acid Carboxylic acid ↑ ↓ ↑ Serra-Compte et al. 2018; Sci. Tot. Environ 618: 1382-1388 Acknowledgements Spanish Ministry of Economy and Competitiveness: SCARCE (CSD2009-00065) and PLAS-MED (CTM2017-89701-C3-2-R) GLOBAQUA-xxx ECsafeSEAFOOD FP7/2007-2013 NANOTRANSFER (ERA SIINN PCIN-2015-182-C02-02) Ramon y Cajal program (RYC-2014-16707) Economy and Knowledge Department of the Catalan Government (Consolidated Research Group ICRA- ENV 2017 SGR 1124).
Details
-
File Typepdf
-
Upload Time-
-
Content LanguagesEnglish
-
Upload UserAnonymous/Not logged-in
-
File Pages18 Page
-
File Size-