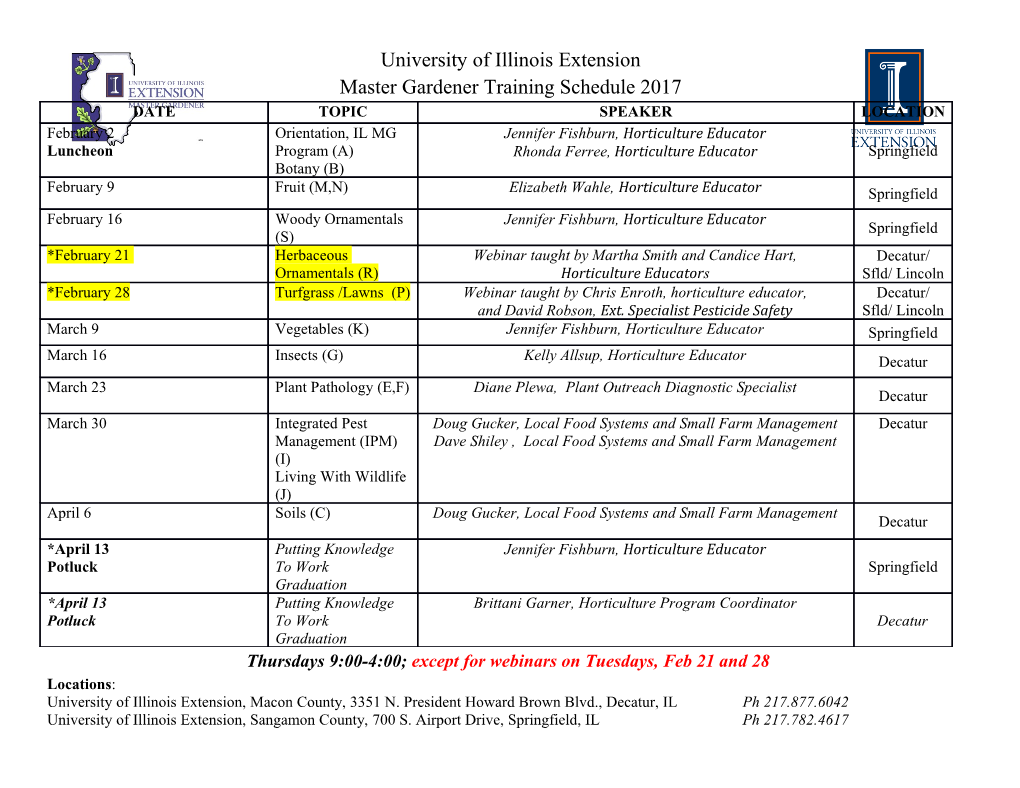
Random variables Integration Expectation 18.175: Lecture 3 Integration Scott Sheffield MIT 18.175 Lecture 3 Random variables Integration Expectation Outline Random variables Integration Expectation 18.175 Lecture 3 Random variables Integration Expectation Outline Random variables Integration Expectation 18.175 Lecture 3 I σ-algebra is collection of subsets closed under complementation and countable unions. Call (Ω; F) a measure space. I Measure is function µ : F! R satisfying µ(A) ≥ µ(;) = 0 P for all A 2 F and countable additivity: µ([i Ai ) = i µ(Ai ) for disjoint Ai . I Measure µ is probability measure if µ(Ω) = 1. I The Borel σ-algebra B on a topological space is the smallest σ-algebra containing all open sets. Random variables Integration Expectation Recall definitions I Probability space is triple (Ω; F; P) where Ω is sample space, F is set of events (the σ-algebra) and P : F! [0; 1] is the probability function. 18.175 Lecture 3 I Measure is function µ : F! R satisfying µ(A) ≥ µ(;) = 0 P for all A 2 F and countable additivity: µ([i Ai ) = i µ(Ai ) for disjoint Ai . I Measure µ is probability measure if µ(Ω) = 1. I The Borel σ-algebra B on a topological space is the smallest σ-algebra containing all open sets. Random variables Integration Expectation Recall definitions I Probability space is triple (Ω; F; P) where Ω is sample space, F is set of events (the σ-algebra) and P : F! [0; 1] is the probability function. I σ-algebra is collection of subsets closed under complementation and countable unions. Call (Ω; F) a measure space. 18.175 Lecture 3 I Measure µ is probability measure if µ(Ω) = 1. I The Borel σ-algebra B on a topological space is the smallest σ-algebra containing all open sets. Random variables Integration Expectation Recall definitions I Probability space is triple (Ω; F; P) where Ω is sample space, F is set of events (the σ-algebra) and P : F! [0; 1] is the probability function. I σ-algebra is collection of subsets closed under complementation and countable unions. Call (Ω; F) a measure space. I Measure is function µ : F! R satisfying µ(A) ≥ µ(;) = 0 P for all A 2 F and countable additivity: µ([i Ai ) = i µ(Ai ) for disjoint Ai . 18.175 Lecture 3 I The Borel σ-algebra B on a topological space is the smallest σ-algebra containing all open sets. Random variables Integration Expectation Recall definitions I Probability space is triple (Ω; F; P) where Ω is sample space, F is set of events (the σ-algebra) and P : F! [0; 1] is the probability function. I σ-algebra is collection of subsets closed under complementation and countable unions. Call (Ω; F) a measure space. I Measure is function µ : F! R satisfying µ(A) ≥ µ(;) = 0 P for all A 2 F and countable additivity: µ([i Ai ) = i µ(Ai ) for disjoint Ai . I Measure µ is probability measure if µ(Ω) = 1. 18.175 Lecture 3 Random variables Integration Expectation Recall definitions I Probability space is triple (Ω; F; P) where Ω is sample space, F is set of events (the σ-algebra) and P : F! [0; 1] is the probability function. I σ-algebra is collection of subsets closed under complementation and countable unions. Call (Ω; F) a measure space. I Measure is function µ : F! R satisfying µ(A) ≥ µ(;) = 0 P for all A 2 F and countable additivity: µ([i Ai ) = i µ(Ai ) for disjoint Ai . I Measure µ is probability measure if µ(Ω) = 1. I The Borel σ-algebra B on a topological space is the smallest σ-algebra containing all open sets. 18.175 Lecture 3 I Question: to prove X is measurable, is it enough to show that the pre-image of every open set is in F? −1 I Theorem: If X (A) 2 F for all A 2 A and A generates S, then X is a measurable map from (Ω; F) to (S; S). I Can talk about σ-algebra generated by random variable(s): smallest σ-algebra that makes a random variable (or a collection of random variables) measurable. I Example of random variable: indicator function of a set. Or sum of finitely many indicator functions of sets. I Let F (x) = FX (x) = P(X ≤ x) be distribution function for 0 X . Write f = fX = FX for density function of X . Random variables Integration Expectation Defining random variables I Random variable is a measurable function from (Ω; F) to (R; B). That is, a function X :Ω ! R such that the preimage of every set in B is in F. Say X is F-measurable. 18.175 Lecture 3 −1 I Theorem: If X (A) 2 F for all A 2 A and A generates S, then X is a measurable map from (Ω; F) to (S; S). I Can talk about σ-algebra generated by random variable(s): smallest σ-algebra that makes a random variable (or a collection of random variables) measurable. I Example of random variable: indicator function of a set. Or sum of finitely many indicator functions of sets. I Let F (x) = FX (x) = P(X ≤ x) be distribution function for 0 X . Write f = fX = FX for density function of X . Random variables Integration Expectation Defining random variables I Random variable is a measurable function from (Ω; F) to (R; B). That is, a function X :Ω ! R such that the preimage of every set in B is in F. Say X is F-measurable. I Question: to prove X is measurable, is it enough to show that the pre-image of every open set is in F? 18.175 Lecture 3 I Can talk about σ-algebra generated by random variable(s): smallest σ-algebra that makes a random variable (or a collection of random variables) measurable. I Example of random variable: indicator function of a set. Or sum of finitely many indicator functions of sets. I Let F (x) = FX (x) = P(X ≤ x) be distribution function for 0 X . Write f = fX = FX for density function of X . Random variables Integration Expectation Defining random variables I Random variable is a measurable function from (Ω; F) to (R; B). That is, a function X :Ω ! R such that the preimage of every set in B is in F. Say X is F-measurable. I Question: to prove X is measurable, is it enough to show that the pre-image of every open set is in F? −1 I Theorem: If X (A) 2 F for all A 2 A and A generates S, then X is a measurable map from (Ω; F) to (S; S). 18.175 Lecture 3 I Example of random variable: indicator function of a set. Or sum of finitely many indicator functions of sets. I Let F (x) = FX (x) = P(X ≤ x) be distribution function for 0 X . Write f = fX = FX for density function of X . Random variables Integration Expectation Defining random variables I Random variable is a measurable function from (Ω; F) to (R; B). That is, a function X :Ω ! R such that the preimage of every set in B is in F. Say X is F-measurable. I Question: to prove X is measurable, is it enough to show that the pre-image of every open set is in F? −1 I Theorem: If X (A) 2 F for all A 2 A and A generates S, then X is a measurable map from (Ω; F) to (S; S). I Can talk about σ-algebra generated by random variable(s): smallest σ-algebra that makes a random variable (or a collection of random variables) measurable. 18.175 Lecture 3 I Let F (x) = FX (x) = P(X ≤ x) be distribution function for 0 X . Write f = fX = FX for density function of X . Random variables Integration Expectation Defining random variables I Random variable is a measurable function from (Ω; F) to (R; B). That is, a function X :Ω ! R such that the preimage of every set in B is in F. Say X is F-measurable. I Question: to prove X is measurable, is it enough to show that the pre-image of every open set is in F? −1 I Theorem: If X (A) 2 F for all A 2 A and A generates S, then X is a measurable map from (Ω; F) to (S; S). I Can talk about σ-algebra generated by random variable(s): smallest σ-algebra that makes a random variable (or a collection of random variables) measurable. I Example of random variable: indicator function of a set. Or sum of finitely many indicator functions of sets. 18.175 Lecture 3 Random variables Integration Expectation Defining random variables I Random variable is a measurable function from (Ω; F) to (R; B). That is, a function X :Ω ! R such that the preimage of every set in B is in F. Say X is F-measurable. I Question: to prove X is measurable, is it enough to show that the pre-image of every open set is in F? −1 I Theorem: If X (A) 2 F for all A 2 A and A generates S, then X is a measurable map from (Ω; F) to (S; S). I Can talk about σ-algebra generated by random variable(s): smallest σ-algebra that makes a random variable (or a collection of random variables) measurable. I Example of random variable: indicator function of a set.
Details
-
File Typepdf
-
Upload Time-
-
Content LanguagesEnglish
-
Upload UserAnonymous/Not logged-in
-
File Pages63 Page
-
File Size-