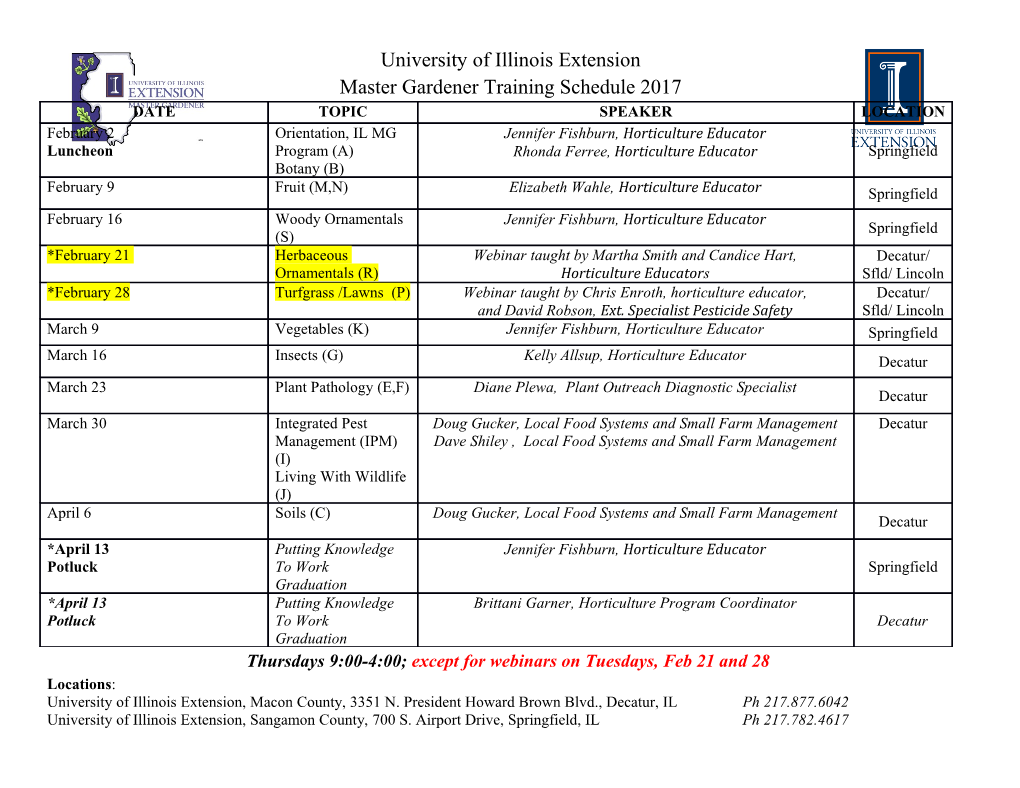
PRMS-Based Physical Modelling for Flood Risk Mapping in the Ruwer and Kyll Basin (Germany) Local Context In the eighties and nineties of this century disastrous floods have regularly occurred in the drainage basin of the river Mosel and its tributaries causing multimillion EURO damages in terms of ecology as well as economy. Due to the almost complete absence of crusting soils and the quite low proportions of impervious areas surface runoff can’t be blamed alone. Instead, complex interactions bet- ween surface runoff, subsurface flow and even groundwater flow seem to be responsible for flood generation in this region. In the Floodgen study, the Remote Sensing Department of the University of Trier has examined two mesoscale catchment areas – the basins of Ruwer (239 km²) and Kyll (843 km²), other relevant physical data (pedological, geo- two rivers joining the Mosel river near Trier. Hydro- logical, topographical and hydrometerological infor- logical complexity required physically-based mo- mation) to be processed within a Geographic Infor- delling integrating high resolution satellite data and mation System (GIS). Data Processing The Hydrological Tool: The Precipitation – Runoff Modeling System (PRMS) PRMS – a physically-based and distributed- image contaminations by atmospheric conditions parameter model developed by the US Geological and relief-induced illumination effects has been im- Survey – accounts for all flood-relevant flow com- plemented to increase land use classification preci- ponents (surface runoff, subsurface flow and sions (cf. flowchart). After pre-processing the dataset groundwater flow). For its application in the Flood- has been interpreted by a hybrid classification using gen study, it was adjusted and optimized for the regional context. Satellite PRMS pursues the concept of sub-dividing the Data basin’s area in hydrologically homogeneous HRUs (“Hydrologic Response Units”). For calculating flow Atmospheric correc- components and water balance terms PRMS con- tion 5S -code siders each HRU to consist of water storages connected in series. Their successive reaction will direct diffuse DEM determine the HRU’s and – by calculating the sum illumination illumination Frsky of all HRUs – the total basin’s hydrological DEM = pixelwise cos γ visible proportion of response. Estimation of iso- and hemispherical sky anisotropic irradiance Cosine Hay & Mc Kay 1985 GIS - Analysis Correction For the definition of appropriate HRUs a GIS-based anisotropic isotropic analysis integrating all physical data available for the irradiance irradiance drainage basins is mandatory. First of all, land use characteristics have been de- Cosine Weighting of the isotropic Correction diffuse light rived by making use of a muli-temporal set of Landsat-5 TM data. Here, a radiometric pre-proces- sing chain including different submodules to eliminate Satellite Data corrected for atmospheric & topographiceffects UNIVERSITÄT TRIER Modified from: Hill, J., Mehl, W.,Radeloff , V. (1995) Remote Sensing Department the maximum likelihood algorithm. The mul- titude of scenes integrated in the approach enabled a reliable separation of summer crops, winter crops, root crops, vineyards, grassland, different forest types, clearcuts, water and settlement (cf. illustrated subset covering the western part of the “Keller Mul- de” around Schillingen in the Ruwer basin). Additionally, soil properties (soil texture and profile depth) and digitized geological maps were integrated in the GIS. Digital elevation data were used to process slope and aspect. For HRU-definition GIS-integrated infor- mation were partly classified and clipped by an overlay analysis. Results of PRMS modelling Ruwer - Kasel gauge 25000 Qsim (l/s) All important water balance terms were quantified 20000 for each HRU (local scale) and for the total basin Qobs (l/s) areas (regional scale).For validation the simulated 15000 daily discharges (Qsim) were compared to the actual Q [l/s] Q 10000 gauge-measured amounts (Qobs). Very good corre- lations have been obtained for both basins 5000 throughout the calibration year 1989 (r=0.96; cf. illustrated hydrographs), and even in a several 0 years covering validation period (1988-1993) co- Nov Dec Jan Feb Mar Apr May Jun Jul Aug Sep Oct efficients didn’t fall short of 0.9. Ruwer - Kasel gauge For explaining flood generation the different flow 8 components have to be thoroughly analysed. 7 surface runoff According to the illustrated PRMS-simulation results 6 subsurface flow the following conclusions can be drawn: groundwater flow 5 - Subsurface flow can be clearly identified as the total discharge 4 most critical flow component for generating [mm] For verification,3 the individual flow components as winter floods. simulated by PRMS have been compared to mea- 2 - Short-term summer peaks are caused by sur- sured chemographs of specific tracer materials face runoff, whereas it plays a minor role in winter. 1 - The groundwater flow (base flow) has nearly no 0 flood generating potentials. Nov Dec Jan Feb Mar Apr May Jun Jul Aug Sep Oct Based on these findings a flood risk mapping has been carried out by a HRU-wise quantification of the flood-critical quickflow components – sub- surface flow and surface runoff. Here, homo- geneous and highly abundant precipitation was used as model input for enabling a direct com- parison of all HRUs’ hydrological behaviour. Based on the model output, quickflow values were assigned to flood-risk classes and mapped accordingly. This type of risk classification is shown by the illustrated subset of the “Keller Mulde”: Flood-generating areas with a high quickflow are indicated in red colours. In general, critical land uses are settlements, vine- yards and arable land (winter or summer crops) over schists or tertiary loams, but also permanent grassland on steep slopes. For further information on FLOODGEN, consult www.ceo.org/ .
Details
-
File Typepdf
-
Upload Time-
-
Content LanguagesEnglish
-
Upload UserAnonymous/Not logged-in
-
File Pages2 Page
-
File Size-