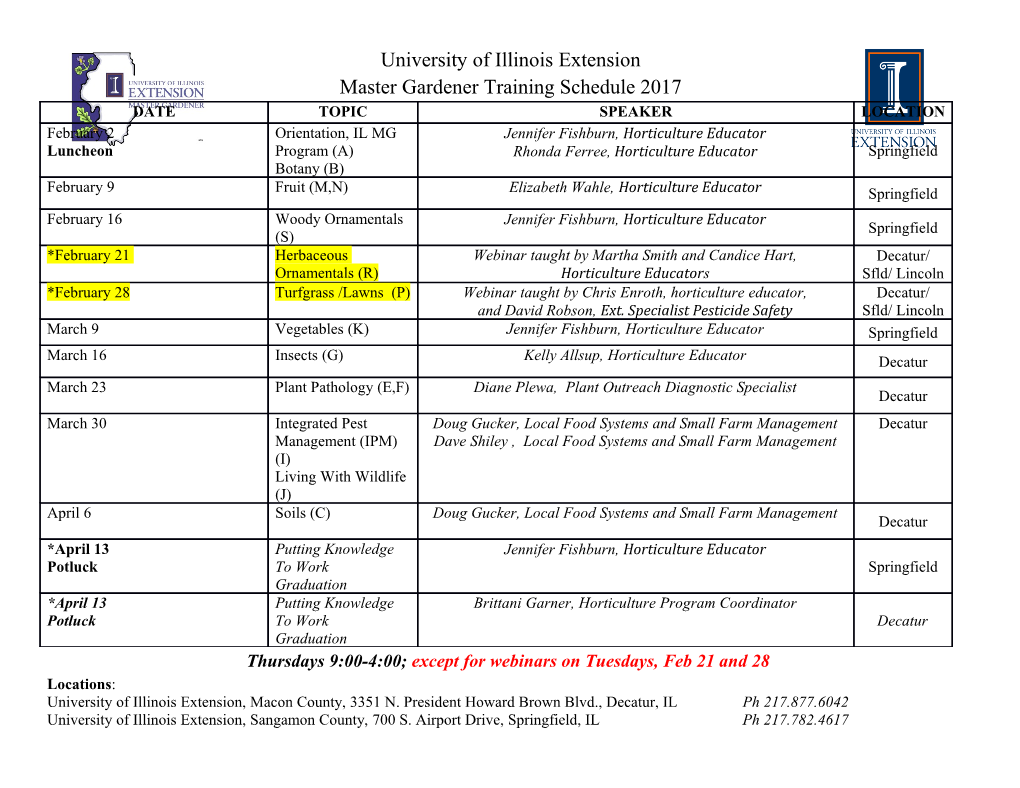
CAPACITY FACTOR RISK AT NUCLEAR POWER PLANTS Yangbo Du* and John E. Parsons** first draft November 2010 this revision January 2012 We develop a model of the dynamic structure of capacity factor risk. It incorporates the risk that the capacity factor may vary widely from year-to-year, and also the risk that the reactor may be permanently shutdown prior to the end of its anticipated useful life. We then fit the parameters of the model to the IAEA’s PRIS dataset of historical capacity factors on reactors across the globe from 1969 to 2010 (i.e., before the Fukushima disaster). The estimated capacity factor risk is greatest in the first year of operation and quickly declines until it is approximately constant through the life of the reactor. We also obtain a relatively low estimate for the mean capacity factor, approximately 73%. We discuss variations on these estimates and emphasize the importance of judgment in making these estimates. * Center for Energy and Environmental Policy Research, MIT, E19-411, 77 Massachusetts Ave., Cambridge, MA 02139 USA, E-mail: [email protected] ** Corresponding author: MIT Sloan School of Management, MIT Center for Energy and Environmental Policy Research, and the MIT Joint Program on the Science and Policy of Global Change, MIT, E19-411, 77 Massachusetts Ave., Cambridge, MA 02139 USA, E-mail: [email protected] 1. INTRODUCTION One of the critical risks facing an investor in a nuclear power plant is uncertainty about the plant’s realized capacity factor. Realized capacity factors show great variation. Although the typical investor’s cash flow model of a proposed plant shows a projected capacity factor of 85% or more, many reactors have problems achieving this target. Oftentimes the shortfall is quite large. According to the Power Reactor Information System (PRIS) database maintained by the International Atomic Energy Agency (IAEA), the realized capacity factor is less than 50% in more than 10% of all reactor years. In one of the countries with the largest nuclear power programs, Japan, the average capacity factor for the three years before the Fukushima disaster, 2008-2010, was only 63%. In the US, performance was extremely poor in the 1970s and 1980s. For example, in 1985 the overall capacity factor for nuclear power plants in the US was 58%. Individual reactor performance varied widely. Subsequently, capacity factors in the US have climbed markedly, so that the average is now slightly above 90%. How should capacity factor risk impact the valuation of a prospective new build power plant? Few economic analyses address this question explicitly. The standard discounted cash flow model simply applies a single risk-adjusted discount rate to the aggregate cash flow line, discounting successive year’s cash flows by the compounded discount rate. Although not widely appreciated, this simple model embodies a very restrictive implicit assumption about the dynamic structure of risk at the level of the aggregate cash flow: that is, the risk or variance of the cash flow grows linearly with time. This structure is consistent with the risk being well described as a geometric Brownian motion, but is not consistent with many other dynamic risk structures. Capacity Page 2 factor risk is unlikely to be well described by a geometric Brownian motion. Uncertainty on the capacity factor parameter will almost certainly not grow linearly with time. But what is the dynamic structure of capacity factor risk? Answering this question is a prerequisite to turning to more advanced valuation techniques, such as a real options model or similar tools.1 In this paper we provide a fully specified model of the dynamic structure of capacity factor risk. We then fit the parameters of the model to the IAEA’s PRIS dataset of historical capacity factors on reactors across the globe from 1969 to 2010 (i.e., before the Fukushima disaster). We find that capacity factor risk is greatest in the first year of operation and quickly declines until it is approximately constant. Whether risk is constant or increasing in later years depends significantly on the probability of a premature permanent shutdown of the reactor. Because these should be rare events, the small historical sample may not provide a reliable estimate, and estimates can vary significantly depending upon how the data is used. Our base case is parameterized with a conservatively low probability of a premature permanent shutdown which yields the approximately constant variance after the initial years of a reactor’s life. We show how the dynamic structure of risk may change as this estimate changes. In the course of fitting our model, we also obtain a relatively low estimate for the mean capacity factor, approximately 73%. This is very low relative to the 85% or higher figures commonly employed in investor cash flow models. We examine various subsets 1 For example, Rothwell (2006) values a nuclear new build using the real option technique. However, he continues to rely upon the Brownian motion assumption. Another example is Samis (2009), who uses a mean reverting process to model the electricity price risk. Page 3 of the data to account for possible factors that could bias our numbers to a low level. These do argue for an upward adjustment in the expected level of the capacity factor through the life of the plant, but the adjustments are small and the final estimate remains well short of the 85% mark, unless the sample chosen is restricted to selected countries over select years. There is a large literature analyzing the determinants of the capacity factor. Joskow and Rozanski (1979) estimate a significant learning curve for the operator, with the expected capacity factor increasing significantly in the first years of operation. They also document some learning by the manufacturer as successive plants of the same design are produced. They document some difference in the learning curve by reactor design, but essentially no difference across countries. Finally, they noted that the larger reactor designs had lower capacity factors. Easterling (1982) estimates that the learning effect on capacity factors is greatest during the first five years of operation. The variability of capacity factors is highest in the first year. He notes that different designs have different mean capacity factors, and that there are persistent differences in the individual unit capacity factors that could possibly represent any number of other factors. Krautmann and Solow (1988) find that the age of the unit, its vintage, the size of the unit, and the past year’s capacity factor are all significant determinants of the expected capacity factor. Rothwell (1990) refines the observation of the capacity factor by organizing the data according to the frequency for refueling, which need not be annual, the frequency used in most analyses. He also decomposes the capacity factor into the service factor—i.e., whether the unit is available or has been taken down for refueling or for repair—and the capacity utilization when operating. Finally, he segments the dataset by manufacturer. Page 4 The results for age are very mixed across manufacturers, and so he argues it should not be used to estimate the expected capacity factor. Similarly, the results for size seem to relate to specific designs and not to size generally.2 Krautmann and Solow (1992) show that improvements in the expected capacity factor with the age of the unit appear to have exhausted themselves in the period following the Three Mile Island accident, and that the units of at least one design were on the declining side of the age-performance curve. Lester and McCabe (1993) find a learning curve effect in the first three years of a units operation, and then document the differential learning curves for units operated at the same site, as well as the role of experience by design, by company and for the industry as a whole. Sturm (1993) identifies declining performance with age for countries in the former Soviet Union and Eastern Europe, especially attributable to the years immediately following the political transformations of the late 1980s and early 1990s. This is in contrast to the improving performance with age in the West at the same time, and even with identical reactor designs. Noting the significant improvements in the capacity factors among US nuclear power plants, Rothwell (2000) provides an updated estimate of the expected capacity factors by design type, manufacturer and size of the unit. Rothwell (2006) updates this for one cohort. Koomey and Hultman (2007) also note the significant improvement in the mean capacity factor at US units. 2 There is related work on factors that one might expect to enter as a determinant of the capacity factor. For example, Roberts and Burwell (1981) estimate the learning curve in licensee events reports and how this is impacted by placing new reactors at the same site as existing reactors. A lower number of events may lead to an increased capacity factor, although the authors did not report on capacity factors. David Maude-Griffin and Rothwell (1996) document how the hazard rate for an unplanned outage declined after the Three-Mile-Island reactor incident and the ensuing regulatory policy changes. Sturm (1994) also evaluates the time between forced outages, and finds significant country differences. Within country no differences by design generation or date of construction are identifiable. Page 5 Our contribution to this literature is our focus on the variability in the capacity factor and the risk structure through time. A portion of the previous literature touches on the variability in the capacity factor, including the random process of unplanned shutdowns and the decision to permanently shutdown a reactor. Rothwell (2007) incorporates a measure of the volatility in the capacity factor into his valuation model.
Details
-
File Typepdf
-
Upload Time-
-
Content LanguagesEnglish
-
Upload UserAnonymous/Not logged-in
-
File Pages115 Page
-
File Size-