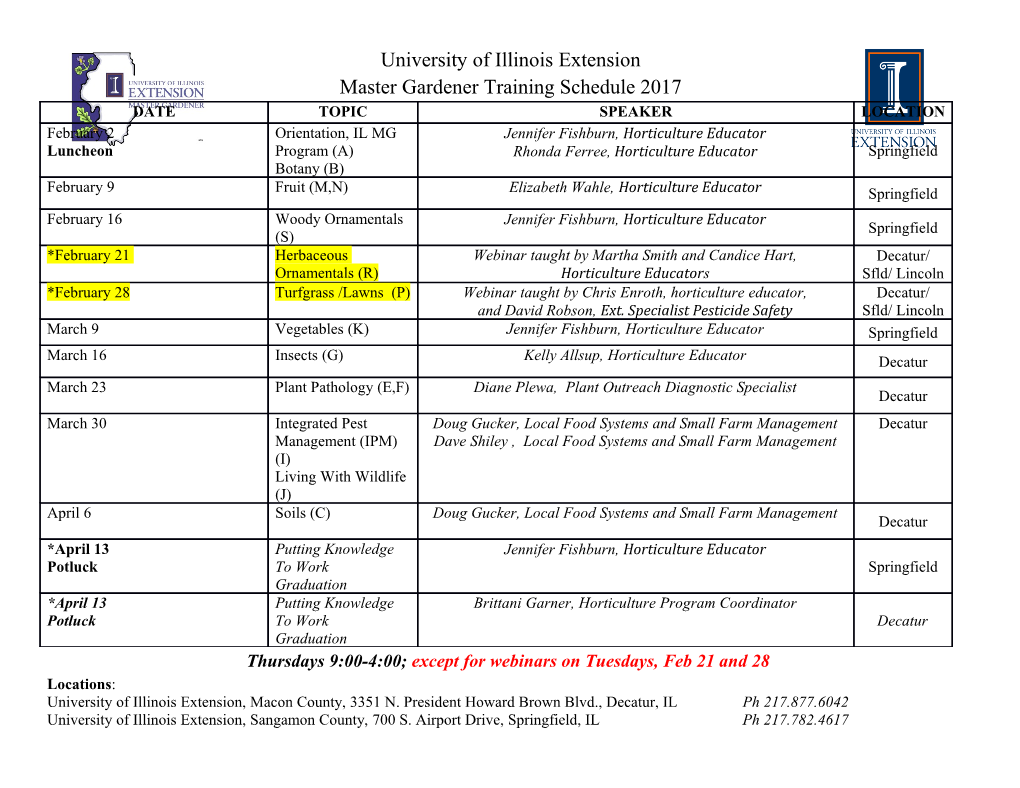
arXiv:1805.01385 Research on the Brain-inspired Cross-modal Neural Cognitive Computing Framework Yang Liu Received: 2018 / Accepted: / Published online: Abstract To address modeling problems of To address problems of cross-modal intelligence, the brain-inspired intelligence, this thesis is focused on neural cognitive computing model based on BIC researching in the semantic-oriented framework design methods was researched in this paper. The study is for multimedia and multimodal information. The organized as follows. Section 2 provides a research Multimedia Neural Cognitive Computing (MNCC) background, which deals with a literature review, model was designed based on the nervous mechanism including studies of BII, brain project, statistical learning, May 2018 and cognitive architecture. Furthermore, the cognitive computing, neuromorphic computing and deep semantic-oriented hierarchical Cross-modal Neural learning. Section 3 gives a definition of the study area, 31 Cognitive Computing (CNCC) framework was proposed structure and mechanism of the nervous system, and ] based on MNCC model, and formal description and function and architecture of the cognitive system. AI analysis for CNCC framework was given. It would Section 4 applies the formal description for design the effectively improve the performance of semantic model for semantic computing. Finally, Section 5 [cs. processing for multimedia and cross-modal information, concludes the chapter. 2 and has far-reaching significance for exploration and realization brain-inspired computing. 2. Literature Review Keywords 1 Deep learning, Cognitive computing, Brain-inspired computing, Cross-modal Neural 2.1. BII and brain project Cognitive Computing, Multimedia neural cognitive The research of artificial intelligence has developed in computing the ups and downs of success and failure. Many countries have made the significant investment in the 1. Introduction scientific research of artificial intelligence in recent years. 1805.01385 v In 1981, Japanese governments made the plans of the The brain-inspired computing (BIC) is the integration of fifth generation computer with artificial intelligence, but neurocognitive science and information technology. It the plan failed because of the man-machine conversation would realize state-of-the-art computing system which based on natural language, automatic program generation arXiv: has advanced in computing ability, efficiency and energy etc. in 1992. However, the research interest of scholars in consumption. It constructs neural cognitive computing artificial intelligence aroused by the success of corporate, model, storage and processing, and explores the new such as DeepBlue in chess, Watson[2] in knowledge generation of Brain-Inspired Intelligence (BII) in the question and answer, and AlphaGo[3] [4] of DeepMind. algorithm, chip and architecture. The research contents The methods and systems of BII are essential to of BIC include the brain-inspired algorithms, and achieve strong artificial intelligence. It depends on the brain-inspired hardware for learning and processing. For study of brain intelligence. In 2010, the US NIH the study of brain-inspired algorithm, which one is launched human connection project on macroscopic simulated from the macroscopical overall cognitive scales. The research object of human connection project function, the other is from the local microscopic is brain network. It includes two basic elements, which structures of neurons, synapses and networks. But there are the node and the connection. The node and is still a lack of effective research how to assemble connection are equally important, and can be defined as advanced function of the complex system from the local the neuron at microcosmic scale, the cortical column on network in mesoscopic. mesoscopic scale, and the brain area on macroscopic BIC has been an obvious success at present, but it is scale. The brain activity map project was launched by the far from reaching the general autonomous intelligence US government in 2013, which focus on functional level, and its model and algorithm lack of cross-modal connectivity research on mesoscopic scale. At the same cognitive and multimedia process ability. There is still a time, the EU initiated the human brain project based on long way to go to study the gap between nature blue brain project. In 2014, Japan started a intelligence and BII [1]. Until now, the study of brain-mapping project called brain mapping by brain-inspired model has not supported the uniform integrated neurotechnologies for disease studies. The cognitive function such as perception, attention, memory, China brain project, entitled "Brain Science and emotion, language and so on. Brain-Inspired Intelligence" was launched in 2016. The basic research of China brain project is the neural circuit mechanisms of brain cognitive functions. China brain Y. Liu () project is developing effective technology of BII, and Key Laboratory of Big Data Analysis and Processing of Henan approaches of brain disease diagnosis/intervention[5]. province, Intelligent Technology and Application Engineering Research Centre of Henan province, and College of Computer 2.2. Statistical learning and cognitive computing Science and Information Engineering, Henan University, Bayesian theory has an indispensable role in the Kaifeng 475004, P. R. China statistical learning. The Bayesian mechanism of the brain e-mail:[email protected] has been validated by a great deal of experimental result 2 of psychology and neurophysiology. Literature[6] SpiNNaker[17], Darwin [18], DianNao and presents a computational model under a Bayesian DaDianNao[19]. The much brain-inspired software criterion that captures the human's learning abilities for a system has been developed, such as SpikeNET, large class of simple concepts, which achieve NEURON, GENESIS and NEST. Compass of IBM human-level performance while outperforming recent company is a multi-threaded; massively parallel deep learning approaches on a challenging one-shot functional simulator and a parallel compiler that mapping classification task. According to Bayesian probability, a network of Long Distance Pathways (LDP) in the brain causal inference and statistical theory, it can simulate the to TrueNorth. Literature [20] present neuron models of perception and cognitive process of visual and aural, the brain as semantic pointer architecture unified which can construct a unified cognitive theoretical network with Nengo, which can simulate the human framework. tasks, such as image recognition, serial working memory, Probabilistic Graphical Model (PGM) based on graph reinforcement learning, counting, question answering, theory and probability statistics is a powerful tool for rapid variable creation, and fluid reasoning. modeling and statistical learning of complex stochastic Deep learning is also known as feature learning, which systems. The each node of PGM only connection with is a combination of big data and high-performance the limited number of other nodes, and its local structure computing. The models that are to be trained have a lot has the small world characteristic. In recent years, there of parameters in classic backpropagation algorithm, is more efficient model of PGM such as topic model, and which increased the risk of overfitting when not enough hierarchical Bayesian model based on Gaussian and training data exist. On the other hand, the method of Dirichlet processes. The topic model is a Bayesian random initialization of network parameters is used, and generative model, which has been widely applied in text the gradient descent algorithm is poor since the gradient retrieval and machine vision. Many models had been diffusion. In addition, it is easy failure because the introduced in the multimedia semantic processing, such algorithm traps into local optimum in non-convex as probabilistic latent semantic analysis, Latent Dirichlet optimization problems. The researchers such as Geoffrey Allocation (LDA), author topic model and hierarchical Hinton, Yoshua Bengio, Yann LeCun and Andrew Ng topic models[7]. The mostly general method is to extract believe that unsupervised learning can contribute to the local semantic features of the media by the bag of training of Deep Neural Network (DNN) [21]. They words model, and then Bayesian reasoning was improve the training problems of the DNN with some employed to intelligence analysis and statistical of the ticks such as unsupervised pre-training, dropout, topic of the multimedia content. In addition, multimedia large-scale labeled data, rectified linear unit activation knowledge graph[8] based on ontology or semantic function and GPU parallel acceleration. network has been used extensively in the information In the traditional model of classification and prediction, retrieval and content-based recommendation. we need to train the classifier according to the features of As a method, cognitive computing has existed for a prior knowledge or artificial extraction. The artificial long time, but it has been making a breakthrough in features would directly affect the results, so ability of recent times. Literature[9] seeks nothing less than to generalization and representation in classification and discover, demonstrate, and deliver the core algorithms of recognition is limited. Deep learning extracts feature and the macaque monkey brain. As a new generation of learning representations based on a set of algorithms that intelligent systems, cognitive computing mainly attempt to model
Details
-
File Typepdf
-
Upload Time-
-
Content LanguagesEnglish
-
Upload UserAnonymous/Not logged-in
-
File Pages12 Page
-
File Size-