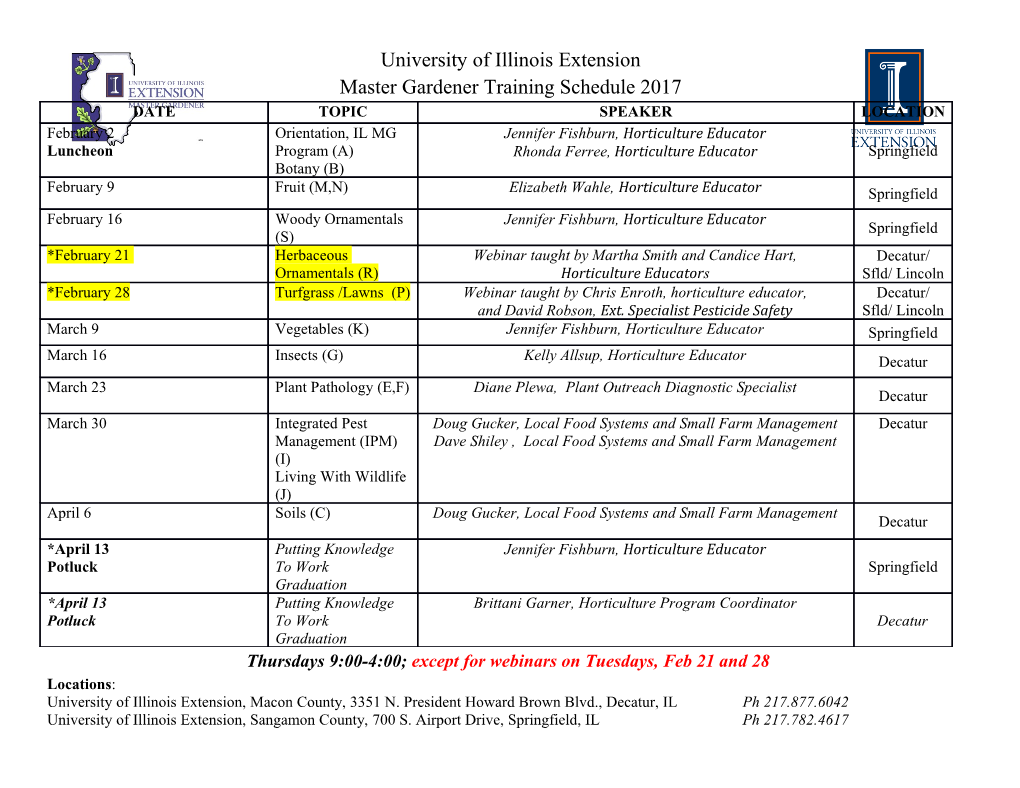
1 Von Mises-Fisher Elliptical Distribution Shengxi Li, Student Member, IEEE, Danilo Mandic, Fellow, IEEE Abstract—A large class of modern probabilistic learning recent applications [13], [14], [15], this type of skewed systems assumes symmetric distributions, however, real-world elliptical distributions results in a different stochastic rep- data tend to obey skewed distributions and are thus not always resentation from the (symmetric) elliptical distribution, which adequately modelled through symmetric distributions. To ad- dress this issue, elliptical distributions are increasingly used to is prohibitive to invariance analysis, sample generation and generalise symmetric distributions, and further improvements parameter estimation. A further extension employs a stochastic to skewed elliptical distributions have recently attracted much representation of elliptical distributions by assuming some attention. However, existing approaches are either hard to inner dependency [16]. However, due to the added dependency, estimate or have complicated and abstract representations. To the relationships between parameters become unclear and this end, we propose to employ the von-Mises-Fisher (vMF) distribution to obtain an explicit and simple probability repre- sometimes interchangeable, impeding the estimation. sentation of the skewed elliptical distribution. This is shown In this paper, we start from the stochastic representation of not only to allow us to deal with non-symmetric learning elliptical distributions, and propose a novel generalisation by systems, but also to provide a physically meaningful way of employing the von Mises-Fisher (vMF) distribution to explic- generalising skewed distributions. For rigour, our extension is itly specify the direction and skewness, whilst keeping the proved to share important and desirable properties with its symmetric counterpart. We also demonstrate that the proposed independence among the components in elliptical distributions. vMF distribution is both easy to generate and stable to estimate, Such generalisation is intuitive and maximally resembles the both theoretically and through examples. original (symmetric) elliptical distributions, which is beneficial Index Terms—Elliptical distribution, von Mises-Fisher distri- in three aspects: i) it admits a simple and closed-form density bution, skewed distribution function, so that all the elliptical distributions can be explicitly generalised as the proposed vMF elliptical distribution; ii) it shares many desirable properties with the original elliptical I. INTRODUCTION distribution, including the independence between the quadratic Probabilistic distributions are a common underpinning term (or the Mahalanobis distance) and the whitened variables, tool in modelling, understanding and predicting a wide the invariance property, and explicit moments; iii) it shares the variety of real-world signals. The normal distribution has robustness properties of the elliptical distributions, and can been a workhorse in probabilistic modelling, as it admits be estimated stably and efficiently, even by a naive numerical a simple representation and mathematical tractability, while gradient descent method. This opens a new avenue for the its application is justified through the central limit theorem. design and implementation of robust probabilistic learning However, issues such as the lack of robustness and flexibility systems, such as generative models in unsupervised learning when dealing with general signals remain a serious obstacle to and discriminative models in supervised learning systems. real-world applications. The family of elliptical distributions generalises normal distributions, and possesses many desired II. EXISTING GENERALISED ELLIPTICAL DISTRIBUTIONS properties such as simple generation, controllable robustness m and flexibility. Elliptical distributions include the normal, A random variable X e 2 R is said to satisfy an elliptical Cauchy, t, logistic, and Weibull distributions [1]. The well- distribution when it has the following stochastic representation behaved nature of elliptical distributions underpins powerful d modelling tools, such as unimodal [2], [3], mixture models X e = µ + RΛU; (1) arXiv:2103.07948v1 [stat.ML] 14 Mar 2021 [4], [5], Bayesian frameworks [6] and probabilistic graphical where R 2 R is a non-negative scalar random variable and models [7]. 0 U 2 Sm −1 is a random variable that is uniformly distributed Despite success, elliptical distributions inherit some of 0 0 on a unit sphere surface, i.e., Sm −1 := fx 2 Rm : xT x = 1g. the limitations of symmetric distributions, which limits their 0 Moreover, µ 2 Rm and Λ 2 Rm×m are two constant modelling power, as in many cases such as financial, biometric parameters that control the distribution centres and scatter. and audio scenarios, the data are not symmetric due to intrinsic It needs to be pointed out that the elliptical distribution coupling, systematic trend, outliers, or a small number of is symmetric about its centre, µ. This is due to the fact samples available. To address this issue, several skewed that R and U in (1) are independent random variables, thus versions of the elliptical distributions have been proposed, constituting a spherical distribution around 0 via RU. The with the majority [8], [9], [10], [11] following a similar way constant, Λ, transforms the sphere into an ellipse (still centred of generalising the normal distribution by adding a skewness around 0), while µ translates the centre of the elliptical weighting function [12]. Although attracting attentions in distribution. Shengxi Li and Danilo Mandic are with the Department of Electrical and When the cumulative density function (cdf) of R is Electronic of Imperial College London. absolutely continuous and Σ = ΛΛT is non-singular, we can 2 write the probability density function (pdf) of the elliptical elliptical distribution, the dispersion is uniquely dominated distribution as by Σ, while this no longer holds in generalised elliptical distributions [16], and could lead to multiple minima/maxima p (x) = det(Σ)−1=2 · c · g(x − µ)T Σ−1(x − µ); (2) X e m when modelling data in practice. Γ(m=2) m=2 where cm = =2π is a constant solely determined by T −1 the dimension, m, while g(t) (t = (x − µ) Σ (x − µ)) is III. GENERALISATION VIA VON MISES-FISHER called the density generator, which is related to the pdf of R DISTRIBUTION in (1) [1]. We denote X e by X e ∼E(µ; Σ; g). The skewness can be achieved by adding a weighting A. The vMF elliptical distribution term π(x − µ) in (2) [8], [9], [10], in a way similar to Being distributed on a unit sphere surface, the vMF is a the skewed normal distribution [12]. However, this type popular choice in directional statistics [23], [24], [25], [26]. of skewness does not necessarily start from a stochastic The vMF distribution is determined by two parameters: µv representation, which impedes clear interpretations of its inner for the main direction and τ for the concentration (denoted relationships, generations and moments. A further successful as vMF (µv; τ)). Therefore, it is natural and beneficial to variant employs conditional distributions of a symmetric replace U in (2) by the vMF distribution as a way of explicitly elliptical distribution [11], to give expressing the direction information. We thus propose a new T type of generalisation on the elliptical distributions in the d Y µ Σ β X se = Y j Y0 > 0; where ∼ E ; ; g form Y0 0 β 1 d (3) X = µ + RΛV; (5) where the parameter β controls the skewness of the distribu- where V denotes a random variable satisfying the vMF tion. The form of (3) represents a typical skewed elliptical distribution vMF (µ ; τ). In our definition, R is the same distribution, of which Y has also been extended to higher v 0 as that in (2), i.e., non-negative and independent of V. More dimensions [17], [18], [19]. Importantly, the form of (3) importantly, when τ ! 0, the vMF distribution approaches is invariant under quadratic forms [9], and is closed under the uniform distribution on a unit sphere U, and consequently marginalisation and affine transforms; we refer to [20] for (5) degenerates into the symmetric elliptical distribution. This more detail. However, the estimation of the above skewed generalisation maximally preserves the formats and desirable elliptical distributions can be ill-posed, especially regarding properties of the symmetric elliptical distribution, such as its shape (skewness) parameter. Although the singularity issue the independence and clear physical meaning of each part. in the information matrix of shape parameter can be relieved In other words, in our vMF elliptical distribution, µ closely by a centralised parametrisation trick [8], the estimation of relates to the data location, Λ controls the dispersion, R the shape parameter in this skewed version could still diverge, governs the tails and V the directions (skewness). As shall be which calls for other penalty techniques [21]. Note that the shown shortly, this is beneficial in both theoretical analysis moment estimation method employed to estimate skewed and practical estimator settings. normal distributions is also inadequate for skewed elliptical The pdf of X in (5) can be obtained in a closed-form as distributions [22]. −1=2 A further generalisation that explicitly comes with the −1=2 Σ (x − µ) pX (x) = det(Σ) · pV ( p ) · g(t); (6) stochastic representations was proposed by Frahm [16] t d ^ X ge = µ + RΛU; (4) where t represents the Mahalanobis distance i.e., t = (x − T −1 and has a form similar to that in (2). The difference lies in µ) Σ (x − µ) and pV (·) is the pdf of vMF distribution the scalar random variable R^ that does no longer require vMF (µv; τ). We provide the proof of (6) in Appendix- to be non-negative and can be even negative; R^ and U VI-A. An intuitive way of understanding our generalisation is are also dependent, which skews the distribution. This through the fact that vMF (µv; τ) resembles a Gaussian dis- 1 generalisation includes the skewed elliptical distribution in (3) tribution N (µv; =τI) constrained on a unit circle (especially as a special case, and is closed under affine transformation, for adequately large τ).
Details
-
File Typepdf
-
Upload Time-
-
Content LanguagesEnglish
-
Upload UserAnonymous/Not logged-in
-
File Pages6 Page
-
File Size-