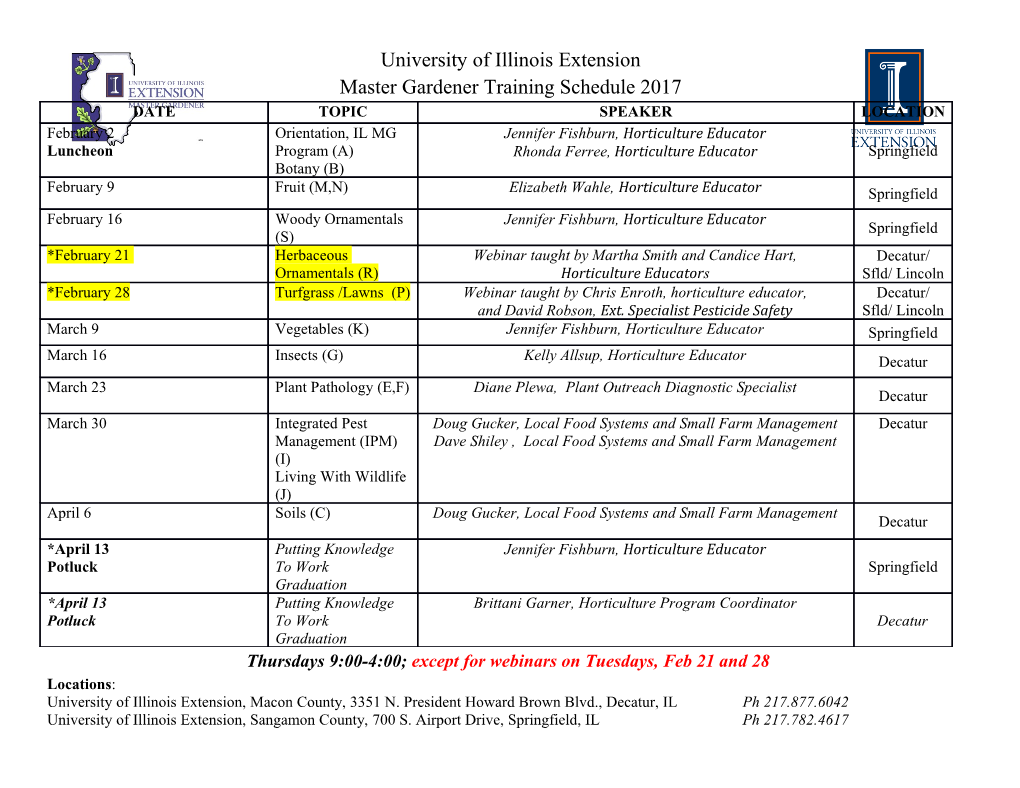
International Journal of Pure and Applied Mathematics Volume 120 No. 7 2018, 325-333 ISSN: 1314-3395 (on-line version) url: http://www.acadpubl.eu/hub/ Special Issue http://www.acadpubl.eu/hub/ A NEW APPROACH OF INTERVAL VALUED VAGUE SET IN PLANTS L. Mariapresenti*, I. Arockiarani** *[email protected] Department of mathematics, Nirmala college for women, Coimbatore Abstract: This paper proposes a new mathematical model which anchors on interval valued vague set theory. An interval valued interview chat with interval valued vague degree is framed. Then in- terval valued vague weighted arithmetic average operators (IVWAA) are defined to aggregate the vague information from the symptomes. Also a new distance measure is formulated for our diagnosis Keywords: Interview chart, aggregate operator, interval valued vague weighted arithmetic average, distance measure. 1. Introduction The fuzzy set theory has been utilized in many approaches to model the diagnostic process.[1, 2, 4, 8, 11, 12, 14]. Sanchez [9] invented a full developed relationships modeling theory of symptoms and diseases using fuzzy set. Atanassov[3] in 1986 defined the notion of intuitionistic fuzzy sets, which is the generalization of notion of Zadeh’s[13] fuzzy set. In 1993 Gau and Buehrer [6] initated the concept of vague set theory which was the generalization of fuzzy set with membership and non membership function. The vague set theory has been explored by many authors and has been applied in different disciplines. The task of a agricultural consultant is to diagnose the disease of the plant. In agriculture diagnosis can be regarded as a label given by the agricultural consultant to describe and synthesize the status of the diseased plant. As an approach to diagnose the disease of the plant, we apply this method to a single plant. We inspect the disease such as fungal disease, bacterial dis- ease and viral disease that affects the leaves of the plant. For this we develop an interview chart with interval vague degree based on the relation among the symptoms and disease. A disease in general have several symptoms. Therefore, it is necessary to aggregate the symptoms. To get the vague information from the symptoms, we 325 International Journal of Pure and Applied Mathematics Special Issue use the interval valued vague weighted arithmetic average (IVWAA) operator and we also propose a distance measure for the diagnosis of disease. The practical example is illustrated by a numerical ex- ample. 2. Preliminaries: Definition 2.1:[12] Let X be a non-empty set. A fuzzy set A drawn from X is defined as A = < x, µA(x) >: x X where µA : X [0, 1]is the membership function{ of the fuzzy∈ set A.} → Definition 2.2:[7] Let [I] be the set of all closed subintervals of the interval [0,1] and µ = [µL, µU ] [I], where muL and muU are the lower extreme and the upper extreme,∈ respectively. For a set X, an IVFS A is given by equation A = < x, µA(x) >: x X where the function µ : X [0, 1] defines the{ degree of membership∈ } of an A → element x to A, and µA(x) = [µAL(x), µAU (x)] is called an interval valued fuzzy number. Definition 2.3:[6] A vague set A in the universe of discourse U is characterized by two membership functions given by: (i) A true membership function tA : U [0, 1] and (ii) A false membership function f : U→ [0, 1] A → where tA is a lower bound on the grade of membership of x derived from the ”evidence for x”, fA is a lower bound on the negation of x derived from the ”evidence against x”, and tA + fA 1. Thus the grade of membership of µ in the vague set A is bounded≤ by a subinterval [tA, 1 fA] of [0,1].This indicates that if the grade of membership of x is− µ(x) then, t (x) µ(x) 1 f (x). A ≤ ≤ − A Definition 2.4:[5] Let A and B be vague sets of the form A = < x, tA, 1 fA]) >: x X and B = < x, tB, 1 fB]) >: x X Then{ − ∈ } { − ∈ } (i) A B if and only if tA(x) tB(x) and 1 fA(x) 1 fB(x) for all⊆x X. ≤ − ≤ − (ii) A = B∈ if and only if A B and B A ⊆ ⊆ 326 International Journal of Pure and Applied Mathematics Special Issue c (iii) A = < x, fA(x), 1 tA(x) > x X (iv) A B{= < x, min(−t (x), t (x))∈, min}(1 f (x), 1 f (x)) > x X { A B − A − B ∈ } (v) A B = < x, max(tA(x), tB(x)), max(1 fA(x), 1 fB(x)) > x X T { − − ∈ } For the sake of simplicity, we shall use the notation A =< x, [tA(x), 1 f (x)]S> instead of A = < x, t , 1 f ]) >: x X . − A { A − A ∈ } Definition 2.5:[10] An interval valued vague sets AV over a uni- verse of discourse X is defined as an object of the form AV = < x , [T V (x ),F V (x )] >, x X where T V : X e D[0, 1] and i A i A i i ∈ A → FAV : X D[0, 1] are called ” truth membership function”e and e e e ”false membership→ function” respectively and where D[0,1] is the set ofe all intervals within [0,1], or in other word an interval valued vague set can be represented by AV =< [(x ), [µ , µ ], [ν , ν ]] >: x X i 1 2 1 2 i ∈ where 0 µ1 µ2 1 and 0 ν1 ν2 1. For each interval ≤ ≤ V ≤ ≤ ≤ ≤ valued vague set A , π V e(x ) = 1 µ V (x ) ν V (x ) and are 1A i − 1A i − 1A i V called degree of hesitancy of xi in A respectively. e f f f 3. The proposed approach: e In this section, we have introduced the proposed approach for diagnostic test for plant disease. The approach is divided into four stage: Let S = S1,S2,Sm , D = ( D1,D2,Dn and P = P1,P2,Pq denote the sets{ of symptoms,} disease{ and plants,} respectively.{ The } two vague relations Q and R are defined as Q = < (p, s), t (p, s), 1 f (p, s) > /(p, s) P S { Q − Q ∈ × } R = < (s, d), t (s, d), 1 f (s, d) > /(s, d) S D { R − R ∈ × } where t (p, s) and 1 f (p, s) indicates the degree for plant’s Q − Q symptoms and tR(s, d) and 1 fR(s, d) are the relationship be- tween symptoms and disease.− Note that Q is defined on the set P S and R on the set S XD. The composition T of R and Q(×T = R Q) for diagnosis× of disease describes the state of plant in terms of◦ disease as a vague relation from P to D given by the truth membership and false membership, 327 International Journal of Pure and Applied Mathematics Special Issue t (p, d) = max min [t (p, s), t (s, d)] T { Q R } 1 fT (p, d) = max min [1 fQ(p, s), 1 fR(s, d)] for all p P and− d D. { − − } ∈ ∈ Stage 1: Collect the diseased plant degree and confirmability degrees from the symptoms of the diseased plant. Confirmablity degrees are presented in the interview chart and the diseased plant degrees are assigned by an agricultural consultant. Stage 2:Calculate the IVWAA of the diseased plants degrees and confirmability degrees respectively, using an aggregate opera- tor. Stage 3:Calculate the distance using the IVWAA calculated in stage 2. Stage 4:Determine the disease of the plant’s based on the dis- tance. Interview chart: The procedure of diagnosis involves interview- ing the agricultural consultant and testing the affected plants. The screening method is done by survey forms which help us in diagnosis of plant disease. Interview chart is the leading part. In this study, we developed an improved interview chart (i.e.,) an interval valued version of interviewing chart based on the knowledge of agricultural consultant. The interview charts have the exact number in [0,1] as the t /1 f degrees. A − A Aggregate operator:In this article we have four symptoms of fun- gal disease, five symptoms of bacterial disease and four symptoms of viral disease. It is in general that some symptoms appear simul- taneously and compositely from the plant. In this case we need to aggregate the interval valued vague information corresponding to the degrees for plant’s symptoms and confirmability degrees. We use the interval valued vague weighted arithmetic average(IVWAA) operator to aggregate vague information from the symptoms. It is defined as follows. Definition 3.1:(IVWAA operator) Let A be the collection of in- terval valued vague values. Then a IVWAA operator is defined as, n n IV W AA(A) = ([1 (1 t (x ))wi , 1 (1 t (x ))wi ], − i=1 − AL i − i=1 − AU i Q Q 328 International Journal of Pure and Applied Mathematics Special Issue n wi n wi [1 i=1(fAL(xi)) , 1 i=1(fAU (xi)) ]) −T − Where w = (w1, w2, ....., wn) be the weight vectors of A. In addi- n Q Q tion, wi > 0 and i=1 wi = 1 CDefinition 3.2:(DistanceP measure) For any two IVVS A = xi, [[tAL(xi), tAU (xi)] , [1 fAL(xi), 1 fAU (xi]] i = 1, 2, 3, ....n) and {h − − i } B = xi, [[tBL(xi), tBU (xi)] , [1 fBL(xi), 1 fBU (xi)]] i = 1, 2, 3, ....n) , the{h normalized hamming distance− considering− the hesitatei part is } defined as, l (A, B) =( 1 ) [ t (x ) t (x ) + t (x ) t (x ) h 4n | AL i − BL i | | AU i − BU i | + (1 fAL)(xi) (1 fBL)(xi) + (1 fAU )(xi) (1 fBU )(xi) | − P − − | | − − − | + (HAL)(xi) (HBL)(xi) + (HAU )(xi) (HBU )(xi) Where H| = 1 (t −+ f ) is the| hesitate| part.− | A − A A 4.
Details
-
File Typepdf
-
Upload Time-
-
Content LanguagesEnglish
-
Upload UserAnonymous/Not logged-in
-
File Pages10 Page
-
File Size-