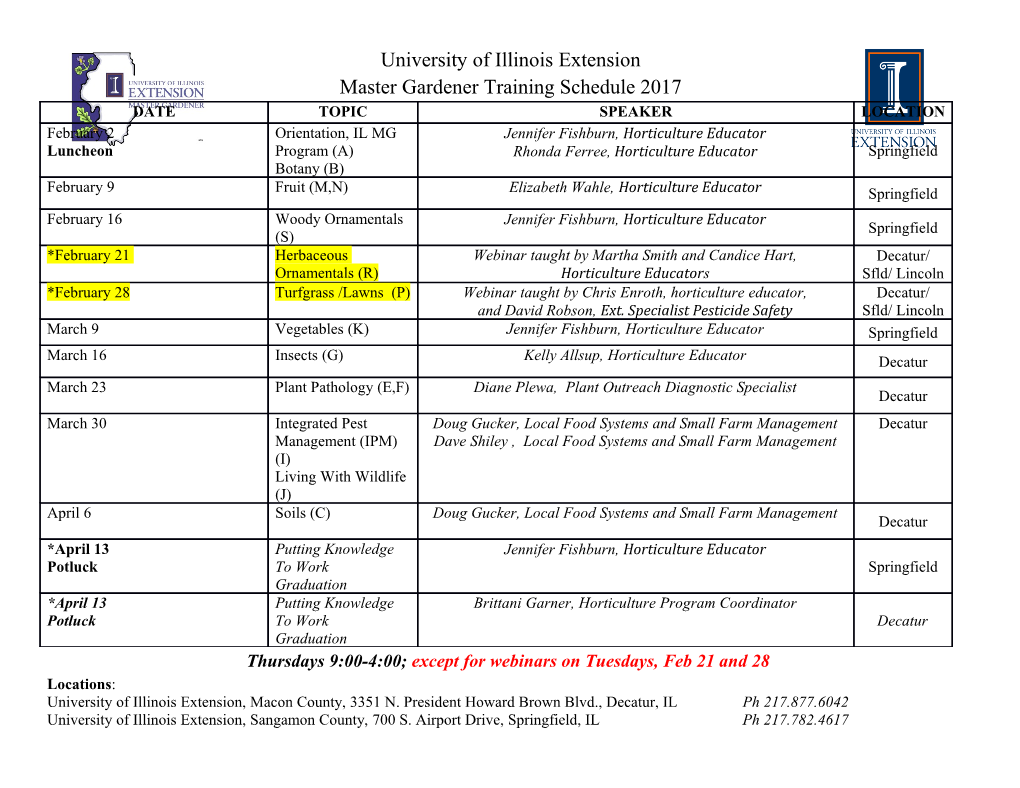
Stochastic Processes A stochastic process • X = X(t) { } is a time series of random variables. Stochastic Processes and Brownian Motion X(t) (or X ) is a random variable for each time t and • t is usually called the state of the process at time t. A realization of X is called a sample path. • A sample path defines an ordinary function of t. • c 2006 Prof. Yuh-Dauh Lyuu, National Taiwan University Page 396 c 2006 Prof. Yuh-Dauh Lyuu, National Taiwan University Page 398 Stochastic Processes (concluded) Of all the intellectual hurdles which the human mind If the times t form a countable set, X is called a • has confronted and has overcome in the last discrete-time stochastic process or a time series. fifteen hundred years, the one which seems to me In this case, subscripts rather than parentheses are to have been the most amazing in character and • usually employed, as in the most stupendous in the scope of its consequences is the one relating to X = X . { n } the problem of motion. If the times form a continuum, X is called a — Herbert Butterfield (1900–1979) • continuous-time stochastic process. c 2006 Prof. Yuh-Dauh Lyuu, National Taiwan University Page 397 c 2006 Prof. Yuh-Dauh Lyuu, National Taiwan University Page 399 Random Walks The binomial model is a random walk in disguise. • Random Walk with Drift Consider a particle on the integer line, 0, 1, 2,... • ± ± Xn = µ + Xn−1 + ξn. In each time step, it can make one move to the right • with probability p or one move to the left with ξn are independent and identically distributed with zero • probability 1 p. mean. − – This random walk is symmetric when p = 1/2. Drift µ is the expected change per period. • Connection with the BOPM: The particle’s position Note that this process is continuous in space. • • denotes the cumulative number of up moves minus that of down moves. c 2006 Prof. Yuh-Dauh Lyuu, National Taiwan University Page 400 c 2006 Prof. Yuh-Dauh Lyuu, National Taiwan University Page 402 Martingalesa X(t), t 0 is a martingale if E[ X(t) ] < for • { ≥ } | | ∞ Position t 0 and ≥ 4 E[ X(t) | X(u), 0 ≤ u ≤ s ]= X(s), s ≤ t. (39) 2 In the discrete-time setting, a martingale means • Time 20 40 60 80 E[ X X ,X ,... ,X ]= X . (40) n+1 | 1 2 n n -2 X can be interpreted as a gambler’s fortune after the • n -4 nth gamble. -6 Identity (40) then says the expected fortune after the • -8 (n + 1)th gamble equals the fortune after the nth gamble regardless of what may have occurred before. aThe origin of the name is somewhat obscure. c 2006 Prof. Yuh-Dauh Lyuu, National Taiwan University Page 401 c 2006 Prof. Yuh-Dauh Lyuu, National Taiwan University Page 403 Martingales (concluded) Still a Martingale? (continued) A martingale is therefore a notion of fair games. Well, no.a • • Apply the law of iterated conditional expectations to Consider this random walk with drift: • • both sides of Eq. (40) on p. 403 to yield Xi−1 + ξi, if i is even, Xi = E[ Xn ]= E[ X1 ] (41) X , otherwise. i−2 for all n. Above, ξ are random variables with zero mean. • n Similarly, E[ X(t)]= E[ X(0)] in the continuous-time a B89201033 • Contributed by Mr. Zhang, Ann-Sheng ( ) on April 13, case. 2005. c 2006 Prof. Yuh-Dauh Lyuu, National Taiwan University Page 404 c 2006 Prof. Yuh-Dauh Lyuu, National Taiwan University Page 406 Still a Martingale? (concluded) It is not hard to see that Still a Martingale? • Xi−1, if i is even, Suppose we replace Eq. (40) on p. 403 with E[ Xi Xi−1 ]= • | X , otherwise. i−1 E[ Xn+1 Xn ]= Xn. | Hence it is a martingale by the “new” definition. • It also says past history cannot affect the future. • But • But is it equivalent to the original definition?a • Xi−1, if i is even, a Contributed by Mr. Hsieh, Chicheng (M9007304) on April 13, 2005. E[ Xi ... ,Xi−2,Xi−1 ]= | X , otherwise. i−2 Hence it is not a martingale by the original definition. • c 2006 Prof. Yuh-Dauh Lyuu, National Taiwan University Page 405 c 2006 Prof. Yuh-Dauh Lyuu, National Taiwan University Page 407 Example Consider the stochastic process Probability Measure (continued) • n A stochastic process X(t), t 0 is a martingale with Z X , n 1 , • { ≥ } { n ≡ i ≥ } respect to information sets It if, for all t 0, i=1 { } ≥ X E[ X(t) ] < and | | ∞ where Xi are independent random variables with zero E[ X(u) I ]= X(t) mean. | t This process is a martingale because for all u > t. • E[ Z Z ,Z ,... ,Z ] The discrete-time version: For all n> 0, n+1 | 1 2 n • = E[ Zn + Xn+1 Z1,Z2,... ,Zn ] E[ X I ]= X , | n+1 | n n = E[ Zn Z1,Z2,... ,Zn ]+ E[ Xn+1 Z1,Z2,... ,Zn ] | | given the information sets In . { } = Zn + E[ Xn+1 ]= Zn. c 2006 Prof. Yuh-Dauh Lyuu, National Taiwan University Page 408 c 2006 Prof. Yuh-Dauh Lyuu, National Taiwan University Page 410 Probability Measure A martingale is defined with respect to a probability • measure, under which the expectation is taken. Probability Measure (concluded) – A probability measure assigns probabilities to states The above implies E[ X I ]= X for any m> 0 • n+m | n n of the world. by Eq. (15) on p. 137. A martingale is also defined with respect to an – A typical In is the price information up to time n. • information set. – Then the above identity says the FVs of X will not – In the characterizations (39)–(40) on p. 403, the deviate systematically from today’s value given the information set contains the current and past values price history. of X by default. – But it needs not be so. c 2006 Prof. Yuh-Dauh Lyuu, National Taiwan University Page 409 c 2006 Prof. Yuh-Dauh Lyuu, National Taiwan University Page 411 Example Martingale Pricing Consider the stochastic process Zn nµ, n 1 . Recall that the price of a European option is the • { − ≥ } • – Z n X . expected discounted future payoff at expiration in a n ≡ i=1 i risk-neutral economy. – X1,X2P,... are independent random variables with mean µ. This principle can be generalized using the concept of • Now, martingale. • Recall the recursive valuation of European option via E[ Zn+1 (n + 1) µ X1,X2,...,Xn ] • − | = E[ Z X ,X ,... ,X ] (n + 1) µ C =[ pCu + (1 p) Cd ]/R. n+1 | 1 2 n − − = Z + µ (n + 1) µ n − – p is the risk-neutral probability. = Z nµ. n − – $1 grows to $R in a period. c 2006 Prof. Yuh-Dauh Lyuu, National Taiwan University Page 412 c 2006 Prof. Yuh-Dauh Lyuu, National Taiwan University Page 414 Martingale Pricing (continued) Example (concluded) Let C(i) denote the value of the option at time i. • Define Consider the discount process • • In X1,X2,... ,Xn . ≡ { } C(i)/Ri, i = 0, 1,... ,n . Then { } • Z nµ, n 1 Then, { n − ≥ } • is a martingale with respect to I . C(i + 1) pCu + (1 p) Cd C { n } E C(i)= C = − = . Ri+1 Ri+1 Ri c 2006 Prof. Yuh-Dauh Lyuu, National Taiwan University Page 413 c 2006 Prof. Yuh-Dauh Lyuu, National Taiwan University Page 415 Martingale Pricing (continued) It is easy to show that Martingale Pricing (continued) • C(k) C Equation (43) holds for all assets, not just options. E C(i)= C = , i k. (42) • Rk Ri ≤ When interest rates are stochastic, the equation becomes • This formulation assumes: a C(i) C(k) • = Eπ , i k. (44) 1. The model is Markovian in that the distribution of M(i) i M(k) ≤ the future is determined by the present (time i ) and – M(j) is the balance in the money market account at not the past. time j using the rollover strategy with an initial 2. The payoff depends only on the terminal price of the investment of $1. underlying asset (Asian options do not qualify). – So it is called the bank account process. aContributed by Mr. Wang, Liang-Kai (Ph.D. student, ECE, Univer- B90902081 It says the discount process is a martingale under π. sity of Wisconsin-Madison) and Mr. Hsiao, Huan-Wen ( ) on • May 3, 2006. c 2006 Prof. Yuh-Dauh Lyuu, National Taiwan University Page 416 c 2006 Prof. Yuh-Dauh Lyuu, National Taiwan University Page 418 Martingale Pricing (concluded) If interest rates are stochastic, then M(j) is a random • Martingale Pricing (continued) variable. In general, the discount process is a martingale in that • – M(0) = 1. C(k) C(i) – M(j) is known at time j 1. Eπ = , i k. (43) − i Rk Ri ≤ Identity (44) on p. 418 is the general formulation of π • – Ei is taken under the risk-neutral probability risk-neutral valuation. conditional on the price information up to time i. Theorem 14 A discrete-time model is arbitrage-free if and This risk-neutral probability is also called the EMM, or • only if there exists a probability measure such that the the equivalent martingale (probability) measure. discount process is a martingale. This probability measure is called the risk-neutral probability measure. c 2006 Prof. Yuh-Dauh Lyuu, National Taiwan University Page 417 c 2006 Prof. Yuh-Dauh Lyuu, National Taiwan University Page 419 Futures Price under the BOPM Futures prices form a martingale under the risk-neutral • Martingale Pricing and Numeraire (concluded) probability.
Details
-
File Typepdf
-
Upload Time-
-
Content LanguagesEnglish
-
Upload UserAnonymous/Not logged-in
-
File Pages20 Page
-
File Size-