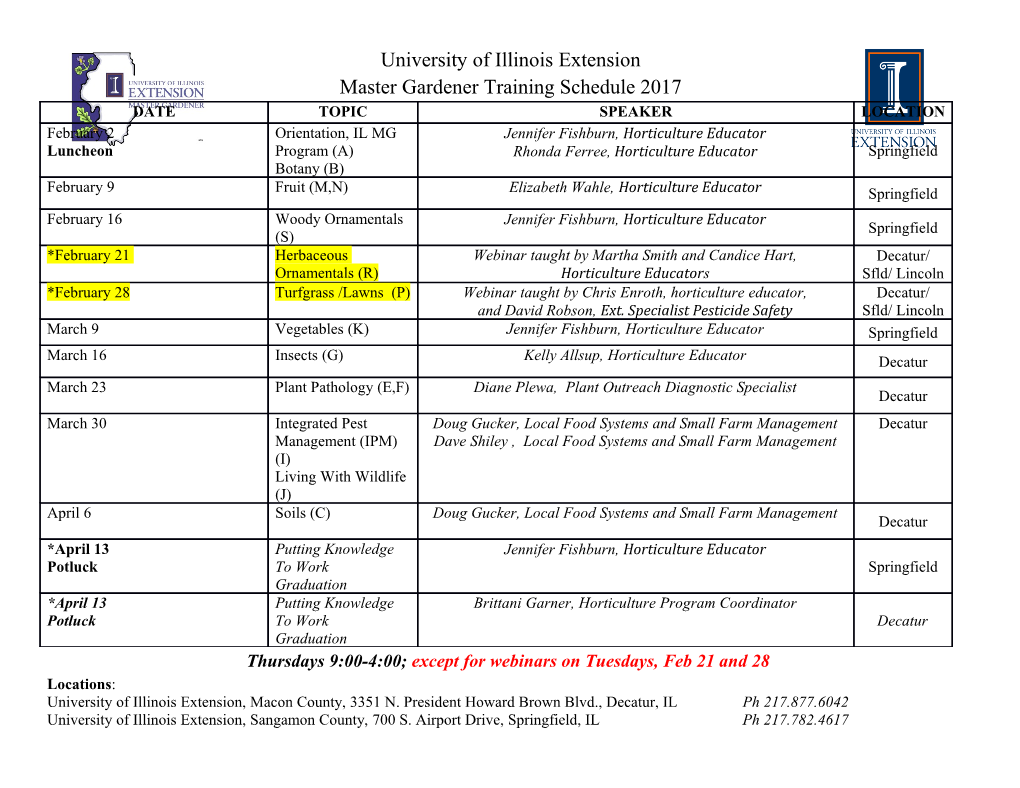
14:51 Friday 18th January, 2013 Chapter 2 The Truth about Linear Regression We need to say some more about how linear regression, and especially about how it really works and how it can fail. Linear regression is important because 1. it’s a fairly straightforward technique which often works reasonably well for prediction; 2. it’s a simple foundation for some more sophisticated techniques; 3. it’s a standard method so people use it to communicate; and 4. it’s a standard method so people have come to confuse it with prediction and even with causal inference as such. We need to go over (1)–(3), and provide prophylaxis against (4). A very good resource on regression is Berk (2004). It omits technical details, but is superb on the high-level picture, and especially on what must be assumed in order to do certain things with regression, and what cannot be done under any assumption. 2.1 Optimal Linear Prediction: Multiple Variables We have a response variable Y and a p-dimensional vector of predictor variables or features X~ . To simplify the book-keeping, we’ll take these to be centered — we can al- ways un-center them later. We would like to predict Y using X~ . We saw last time that the best predictor we could use, at least in a mean-squared sense, is the conditional expectation, r (~x)=E Y X~ = ~x (2.1) | î ó Instead of using the optimal predictor r (~x), let’s try to predict as well as possible while using only a linear function of ~x, say ~x β. This is not an assumption about the · 36 37 2.1. OPTIMAL LINEAR PREDICTION: MULTIPLE VARIABLES world, but rather a decision on our part; a choice, not a hypothesis. This decision can be good — ~x β could be a close approximation to r (~x) — even if the linear hypothesis is wrong. · One reason to think it’s not a crazy decision is that we may hope r is a smooth function. If it is, then we can Taylor expand it about our favorite point, say ~u: p @ r 2 r (~x)=r (~u)+ (xi ui )+O( ~x ~u ) (2.2) @ x ! − k − k i=1 i ~u X or, in the more compact vector-calculus notation, 2 r (~x)=r (~u)+(~x ~u) r (~u)+O( ~x ~u ) (2.3) − ·r k − k 2 If we only look at points ~x which are close to ~u, then the remainder terms O( ~x ~u ) are small, and a linear approximation is a good one1. k − k Of course there are lots of linear functions so we need to pick one, and we may as well do that by minimizing mean-squared error again: 2 MSE(β)=E Y X~ β (2.4) − · ïÄ ä ò Going through the optimization is parallel to the one-dimensional case (see last chap- ter), with the conclusion that the optimal β is 1 ~ β = v− Cov X ,Y (2.5) ~ î ó ~ where v is the covariance matrix of X , i.e., vij = Cov Xi ,Xj , and Cov X ,Y is the vector of covariances between the predictor variablesî and Yó, i.e. Cov îX~ ,Y ó i = Cov Xi ,Y . î ó Multiple regression would be a lot simpler if we could just do a simple regression ⇥ ⇤ for each predictor variable, and add them up; but really, this is what multiple regres- sion does, just in a disguised form. If the input variables are uncorrelated, v is diagonal 1 (vij = 0 unless i = j), and so is v− . Then doing multiple regression breaks up into a sum of separate simple regressions across each input variable. When the input vari- 1 ables are correlated and v is not diagonal, we can think of the multiplication by v− as de-correlating X~ — applying a linear transformation to come up with a new set of inputs which are uncorrelated with each other.2 Notice: β depends on the marginal distribution of X~ (through the covariance matrix v). If that shifts, the optimal coefficients β will shift, unless the real regression function is linear. 1 2 If you are not familiar with the big-O notation like O( ~x ~u ), now would be a good time to read Appendix B. k − k 2If Z~ is a random vector with covariance matrix I , then wZ~ is a random vector with covariance matrix wT w. Conversely, if we start with a random vector X~ with covariance matrix v, the latter has a “square 1/2 1/2 1/2 1/2 ~ root” v (i.e., v v = v), and v− X will be a random vector with covariance matrix I. When we ~ 1 ~ ~ 1/2 1/2 ~ write our predictions as X v− Cov X ,Y , we should think of this as X v− v− Cov X ,Y .We 1/2 use one power of v− to transformî theó input features into uncorrelatedÄ variablesäÄ beforeî takingóä their correlations with the response, and the other power to decorrelate X~ . 14:51 Friday 18th January, 2013 2.1. OPTIMAL LINEAR PREDICTION: MULTIPLE VARIABLES 38 2.1.1 Collinearity 1 ~ The formula β = v− Cov X ,Y makes no sense if v has no inverse. This will happen if, and only if, the predictorî ó variables are linearly dependent on each other — if one of the predictors is really a linear combination of the others. Then (as we learned in linear algebra) the covariance matrix is of less than “full rank” (i.e., “rank deficient”) and it doesn’t have an inverse. So much for the algebra; what does that mean statistically? Let’s take an easy case where one of the predictors is just a multiple of the others — say you’ve included people’s weight in pounds (X1) and mass in kilograms (X2), so X1 = 2.2X2. Then if we try to predict Y , we’d have Yˆ = β1X1 + β2X2 + β3X3 + ...+ βp Xp (2.6) p = 0X1 +(2.2β1 + β2)X2 + βi Xi (2.7) i=3 Xp =(β1 + β2/2.2)X1 + 0X2 + βi Xi (2.8) i=3 X p = 2200X1 +(1000 + β1 + β2)X2 + βi Xi (2.9) − i 3 X= In other words, because there’s a linear relationship between X1 and X2, we make the coefficient for X1 whatever we like, provided we make a corresponding adjustment to the coefficient for X2, and it has no effect at all on our prediction. So rather than having one optimal linear predictor, we have infinitely many of them. There are three ways of dealing with collinearity. One is to get a different data set where the predictor variables are no longer collinear. A second is to identify one of the collinear variables (it doesn’t matter which) and drop it from the data set. This can get complicated; principal components analysis (Chapter 17) can help here. Thirdly, since the issue is that there are infinitely many different coefficient vectors which all minimize the MSE, we could appeal to some extra principle, beyond prediction accuracy, to select just one of them, e.g., try to set as many of the coefficients to zero as possible (Appendix E.4.1). 2.1.2 The Prediction and Its Error Once we have coefficients β, we can use them to make predictions for the expected value of Y at arbitrary values of X~ , whether we’ve an observation there before or not. How good are these? If we have the optimal coefficients, then the prediction error will be uncorrelated with the predictor variables: ~ ~ ~ ~ 1 ~ ~ Cov Y X β,X = Cov Y,X Cov X (v− Cov X ,Y ),X (2.10) − · − · î ó î ~ ó 1î ~ î ó ó = Cov Y,X vv− Cov Y,X (2.11) − = 0î ó î ó (2.12) 14:51 Friday 18th January, 2013 39 2.1. OPTIMAL LINEAR PREDICTION: MULTIPLE VARIABLES Moreover, the expected prediction error, averaged over all X~ , will be zero (exercise). In general, however, the conditional expectation of the error is not zero, E Y X~ β X~ = ~x = 0 (2.13) − · | 6 î ó and the conditional variance is not constant in ~x, ~ ~ ~ ~ Var Y X β X = ~x1 = Var Y X β X = ~x2 (2.14) − · | 6 − · | î ó î ó 2.1.3 Estimating the Optimal Linear Predictor To actually estimate β from data, we need to make some probabilistic assumptions about where the data comes from. A quite weak but sufficient assumption is that ~ observations (Xi ,Yi ) are independent for different values of i, with unchanging co- variances. Then if we look at the sample covariances, they will, by the law of large numbers, converge on the true covariances: 1 xT y Cov X~ ,Y (2.15) n ! 1 î ó xT x v (2.16) n ! where as before x is the data-frame matrix with one row for each data point and one column for each feature, and similarly for y. So, by continuity, T 1 T β =(x x)− x y β (2.17) ! and we have a consistent estimator.b On the other hand, we could start with the residual sum of squares n 2 RSS(β) yi ~xi β (2.18) ⌘ i=1 − · X and try to minimize it. The minimizer is the same β we got by plugging in the sample covariances. No probabilistic assumption is needed to minimize the RSS, but it doesn’t let us say anything about the convergence of βb. For that, we do need some assumptions about X~ and Y coming from distributions with unchanging covariances.
Details
-
File Typepdf
-
Upload Time-
-
Content LanguagesEnglish
-
Upload UserAnonymous/Not logged-in
-
File Pages20 Page
-
File Size-