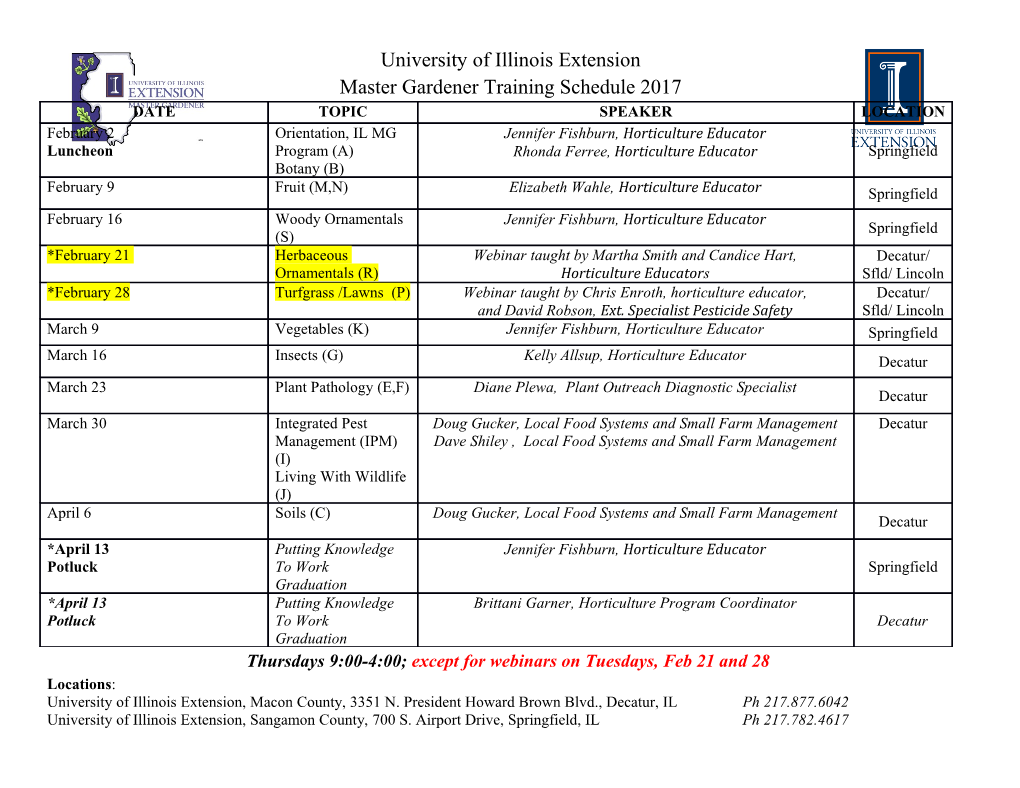
ST 762 Nonlinear Statistical Models for Univariate and Multivariate Response Quadratic versus Linear Estimating Equations GLS estimating equations 0 1 n fβj 0 2 2 −1 X σ gj 0 Yj − fj @ 2 2 1/σ A 4 4 2 2 2 = 0: 0 2σ gj 0 2σ gj (Yj − fj ) − σ gj j=1 νθj Estimating equations for β are linear in Yj . Estimating equations for β only require specification of the first two moments. GLS is optimal among all linear estimating equations. 1 / 26 Quadratic versus Linear Estimating Equations ST 762 Nonlinear Statistical Models for Univariate and Multivariate Response Gaussian ML estimating equations 0 2 2 1 n fβj 2σ gj νβj 2 2 −1 X σ g 0 Yj − fj j = 0: @ 2 2 1/σ A 4 4 (Y − f )2 − σ2g 2 0 2σ gj 0 2σ gj j j j j=1 νθj Estimating equations for β are quadratic in Yj . Estimating equations for β require specification of the third and fourth moments as well. Specifically, if we let Yj − f (xj ; β) j = ; σg (β; θ; xj ) then we need to know 3 ∗ 2 ∗ E j = ζj and var j = 2 + κj : 2 / 26 Quadratic versus Linear Estimating Equations ST 762 Nonlinear Statistical Models for Univariate and Multivariate Response Questions ∗ ∗ ^ If we know the true values ζj and κj , how much is β improved using the quadratic estimating equations versus using the linear estimating equations? If we use working values (for example ζj = κj = 0, corresponding to ∗ ∗ normality) that are not the true values (i.e., ζj and κj ), is there any improvement in using the quadratic estimating equations? If we use working variance functions that are not the true variance functions, is there any improvement in using the quadratic estimating equations? 3 / 26 Quadratic versus Linear Estimating Equations ST 762 Nonlinear Statistical Models for Univariate and Multivariate Response General form of quadratic estimating equations: 0 2 2 1 n fβ;j 2σ g νβ;j j σ2g 2 ζ σ3g 3 −1 X 1 j j j B C 3 3 4 4 @ 2 2 σ A ζj σ g (2 + κj ) σ g j=1 0 2σ gj j j νθ;j Yj − fj × 2 2 2 = 0: (Yj − fj ) − σ gj 4 / 26 Quadratic versus Linear Estimating Equations ST 762 Nonlinear Statistical Models for Univariate and Multivariate Response Large sample distribution for all parameters jointly: 0 ^ 1 p β − β0 L −1 −1 n @ σ^ − σ0 A −! N 0; A BA : ^ θ − θ0 Here n 1 X T −1 A = lim D0;j V D0;j ; n!1 n 0;j j=1 n 1 X T −1 −1 B = lim D0;j V var (s0;j j xj ) V D0;j ; n!1 n 0;j 0;j j=1 5 / 26 Quadratic versus Linear Estimating Equations ST 762 Nonlinear Statistical Models for Univariate and Multivariate Response Also 2 2 3 3 σ gj ζj σ gj Vj = 3 3 4 4 ; ζj σ gj (2 + κj ) σ gj 0 2 2 1T fβ;j 2σ gj νβ;j Dj = B 1 C @ 2 2 σ A 0 2σ gj νθ;j and V0;j and D0;j are evaluated at the true β0 and θ0. 6 / 26 Quadratic versus Linear Estimating Equations ST 762 Nonlinear Statistical Models for Univariate and Multivariate Response Also 2 2 ∗ 3 3 σ gj ζj σ gj var (s0;j j xj ) = ∗ 3 3 ∗ 4 4 ; ζj σ gj 2 + κj σ gj the true variance matrix. Note that if the working values for ζj and κj are the same as the true values, var (s0;j j xj ) = V0;j ; so B = A, and the large sample distribution simplifies to 0 ^ 1 p β − β0 L −1 n @ σ^ − σ0 A −! N 0; A : ^ θ − θ0 7 / 26 Quadratic versus Linear Estimating Equations ST 762 Nonlinear Statistical Models for Univariate and Multivariate Response 1=2 ^ To deduce the limiting distribution of n (β − β0), it would be necessary to carry out the indicated matrix inversion and multiplications and extract the upper left p × p submatrix of the result. It is possible to show that, just as GLS is optimal among linear estimating equations, β^ (as well asσ ^ and θ^) are optimal among quadratic estimating equations, provided the working values for ζj and κj are the true values. Next we consider a special case to gain better ideas of comparison between linear and quadratic estimating equations. 8 / 26 Quadratic versus Linear Estimating Equations ST 762 Nonlinear Statistical Models for Univariate and Multivariate Response If we take 3 2 E j = 0 and var j = 2 + κ for all j; while in truth 3 ∗ 2 ∗ E j = ζ and var j = 2 + κ for all j then we have 1=2 ^ L 2 −1 −1 n β − β0 −! N 0; σ0Γ ∆Γ : 9 / 26 Quadratic versus Linear Estimating Equations ST 762 Nonlinear Statistical Models for Univariate and Multivariate Response Here Γ = lim Γn n!1 4σ2 = lim n−1 XT WX + 0 RT PR n!1 2 + κ 4σ2 = Σ−1 + 0 Σ WLS 2 + κ β and ∆ = lim ∆n n!1 4σ2(2 + κ∗) 2σ ξ∗ = lim XT WX + 0 RT PR + 0 XT W1=2PR + RT PW1=2X n!1 (2 + κ2) 2 + κ 4σ2(2 + κ∗) 2σ ζ∗ = Σ−1 + 0 Σ + 0 T + TT WLS (2 + κ)2 β 2 + κ β β 10 / 26 Quadratic versus Linear Estimating Equations ST 762 Nonlinear Statistical Models for Univariate and Multivariate Response and 0 T 1 0 T 1 νβ01 τθ01 B . C B . C R = @ . A Q = @ . A νT τ T β0n n×p θ0n n×(q+1) and P = I − Q(QT Q)−1QT . 11 / 26 Quadratic versus Linear Estimating Equations ST 762 Nonlinear Statistical Models for Univariate and Multivariate Response Recall that, if the first two moments are correctly specified, then p ^ L 2 n βGLS − β0 −! N 0; σ0ΣWLS : ^ First we note that the properties of βGLS do not depend on those of ^ ^ σ^ and θ, whereas the properties of βML do. Next we compare β^ from the linear and quadratic equations in various scenarios under this special case. 12 / 26 Quadratic versus Linear Estimating Equations ST 762 Nonlinear Statistical Models for Univariate and Multivariate Response When the data are really normal That is, we choose ζ = 0 and κ = 0 while ζ∗ = 0 and κ∗ = 0. Then −1 2 −1 −1 Γ = ΣWLS + 2σ0Σβ = ΣML and ∆ = ΣML: So p ^ L 2 n βML − β0 −! N 0; σ0ΣML : We can show that ΣGLS − ΣML is nonnegative definite, as −1 2 −1 ΣML = (ΣWLS + 2σ0Σβ) −2 −1 −1 = ΣWLS − ΣWLS ΣWLS + σ0 =2Σβ ΣWLS: 13 / 26 Quadratic versus Linear Estimating Equations ST 762 Nonlinear Statistical Models for Univariate and Multivariate Response ^ ^ That is, βML is more efficient compared to βGLS when the data truly are normal and we use the normal theory ML estimating equations for β. The source of improvement is from Σβ, which arises from taking advantage of the additional information β available in the variance function g(·). 14 / 26 Quadratic versus Linear Estimating Equations ST 762 Nonlinear Statistical Models for Univariate and Multivariate Response When the data are only symmetrically distributed That is, we choose ζ = 0 and κ = 0 while ζ∗ = 0 and κ∗ > 0. Then −1 2 −1 2 Γ = ΣWLS + 2σ0Σβ and ∆ = ΣWLS + (2 + κ)σ0Σβ: p ^ L 2 −1 n βML − β0 −! N 0; σ0ΣQ ; with ΣQ = Γ ∆Γ ∗ We can show that ΣGLS − ΣQ is nonnegative definite, if κ ≤ 2. ^ That is, the optimality of βML no longer applies uniformly, when the data are only symmetric and we use the normal theory ML estimating equations for β. 15 / 26 Quadratic versus Linear Estimating Equations ST 762 Nonlinear Statistical Models for Univariate and Multivariate Response When the data are symmetrically distributed ...and we correctly specify both ζ and κ. That is, we choose ζ = ζ∗ = 0 and κ = κ∗. Then 4σ2 4σ2 Γ = Σ−1 + 0 Σ and ∆ = Σ−1 + 0 Σ WLS 2 + κ∗ β WLS 2 + κ∗ β and p ^ L 2 n βML − β0 −! N 0; σ0ΣC ; with 4σ2 −1 Σ = Σ−1 + 0 Σ C WLS 2 + κ∗ β 16 / 26 Quadratic versus Linear Estimating Equations ST 762 Nonlinear Statistical Models for Univariate and Multivariate Response We can show that ΣGLS − ΣC is nonnegative definite. ^ ^ That is, βML is more efficient compared to βGLS when we know the data are symmetric and we are able to specify correctly a value for the excess kurtosis. 17 / 26 Quadratic versus Linear Estimating Equations ST 762 Nonlinear Statistical Models for Univariate and Multivariate Response When the variance function g(·) does not depend on β In this case, Σβ = 0 and Tβ = 0. Then −1 Γ = ∆ = ΣWLS and p ^ L 2 n βML − β0 −! N 0; σ0ΣWLS : That is, there is nothing to be gained by using a quadratic estimating equation over a linear one, because there is no additional information on β to be gained from g(·). 18 / 26 Quadratic versus Linear Estimating Equations ST 762 Nonlinear Statistical Models for Univariate and Multivariate Response In general The large sample properties of the quadratic estimator depend on the assumed and true third and fourth moments of the data. Those of the GLS estimator do not, and are unchanged regardless of the nature of the true third and fourth moments. If the third and fourth moments are correctly specified, the linear estimator is inefficient relative to the quadratic estimator for β. If these are not correctly specified, it is no longer clear that one estimator dominates the other in terms of efficiency.
Details
-
File Typepdf
-
Upload Time-
-
Content LanguagesEnglish
-
Upload UserAnonymous/Not logged-in
-
File Pages26 Page
-
File Size-