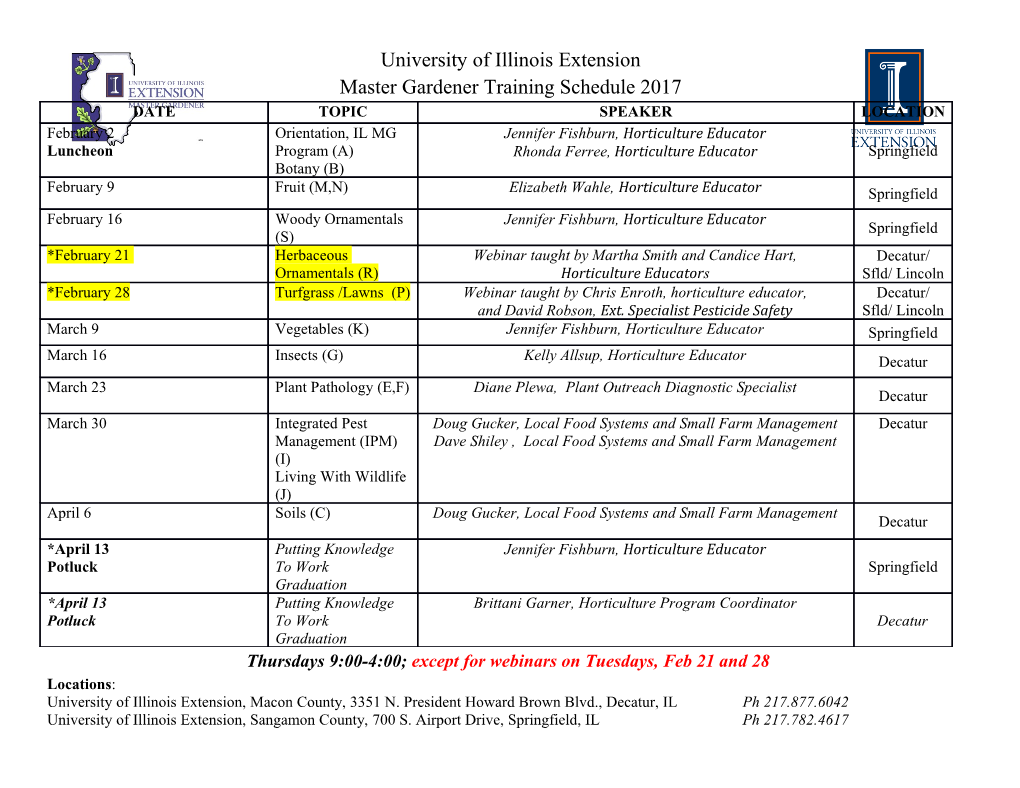
Stochastic Volatility Modeling: From Rough Heston Model to Affine Volterra Equatio Zixuan Wang, Yuanchun Ye Mentor: Shuoqing Deng Abstract In the famous Heston model, where the variance satisfies a CIR process, the characteristic function of the log-price can be calculated explicitly by the Riccati equation. When replacing the Brownian motion by the fractional Brownian motion, one now faces the Rough Heston model and difficulties arise because of the lack of Markovian structure. In this paper, we will first study the mechanism of calculating the characteristic function of the Rough Heston model using the convergence of a suitable class of Hawkes processes. Then, we will study the optimal consumption and stochastic control problems in the Heston framework and Affine Volterra framework by some specific examples. Contents 1 Introduction 1 2 Local Volatility2 3 Heston model4 3.1 Heston Dynamics....................................4 3.2 The Heston PDE....................................4 3.3 The Call Price.....................................5 4 Rough Heston Model6 4.1 Relationship between Hawkes process and Rough Heston model..........6 4.2 The rough limits of Hawkes processes........................7 5 Merton Problem8 6 Future Work 9 7 References 10 1 Introduction In financial mathematics, the Black-Scholes Equation is a well-known partial differential equa- tion (PDE) that governs the evolution of price of a European call or put under Black-Scholes 1 model. The assumptions under the model state that the price of assets follows a geometric Brownian motion with constant drift and volatility. The Black-Scholes PDE is @U 1 @2U @U + σ2S2 + rS = rU; (1.1) @t 2 @S2 @S where U is the price of the option as a function of stock price S and time t, r is the risk-free interest rate, and σ is the volatility of the stock. The derivation of this PDE can be obtained using pricing by replication, where we have assumed that the market is complete under Black-Scholes model. Here we are going to use 1 option and φ stocks to replicate cash X, which has the risk-free rate r. In continuous time, we have Xt = u(t; St) + φSt; (1.2) dXt = rXtdt: (1.3) Under Black-Scholes model, we also have dSt = rStdt + σStdWt. Applying Ito's formula to Xt, we have dxt 1 2 2 = utdt + uxdSt + σ St dt + φdSt 2 (1.4) 1 = (u + σ2S2)dt + (u + φ)(rS dt + σS dW ) t 2 t x t t t = rXtdt Now, we need to match the coefficients before dt and dWt: • Coefficient before dWt: ux + φ = 0; 1 2 2 • Coefficient before dt: ut + 2 σ St = rXt = r(u(t; St) + φSt). Solving for φ and plug back to the second equation, we obtain the famous Black-Scholes PDE in equation(1.1). However, due to the stock market crash in 1987, the classical Black-Scholes model began to lose its validity. Traders realized that extreme events could happen and markets have a significant skew. As a result, the possibility for extreme events needed to be factored into option pricing, and volatility smiles started occurring. It shows that implied volatility increases or decreases as options move more in-the-money (ITM) or out-of-the-money (OTM). In order to incorporate real-world situations like non-constant volatility into our volatility model, Dupire Local Volatility and Heston model was introduced. Moreover, rough Heston model will also be discussed in the following passage. 2 Local Volatility The concept of a local volatility was developed when Bruno Dupire, Emanuel Derman and Iraj Kani noted that there is a unique diffusion process consistent with the risk neutral densities derived from the market prices of European options. They have observed that volatility could be a function of stock price and time. Thus, the Dupire Local Volatility in its most general form is(assume there is no dividend): @C 1 @2C @C = σ2K2 − rK : (2.5) @T 2 @K2 @K 2 The underlying St follows the process dSt = rtStdt + σ(St; t)StdWt: (2.6) We need the following preliminaries: R T • Discount factor P (t; T ) = exp(− t rsds). • Fokker-Planck Equation. Denote f(St; t) the probability density function of the underlying price St at time t. Then f satisfies the following equation: @f @ @2 = − [µSf(S; t)] + σ2S2f(S; t): (2.7) @t @S @S2 The implication of applying Fokker-Planck Equation in our model is that there are lots of liquid options (vanilla options) in the market, and we can use the price of the options to derive the volatility function, which is consistent with the option price. • time-t price of European call with strike K, denoted by C = C(S; K): Z 1 + C = P (t; T )E[(ST − K) ] = P (t; T ) (ST − K)f(S; T )dS: (2.8) K We need the following derivatives of the call C(St; t), which can be easily derived using equa- tion(2.8): @C Z 1 = −P (t; T ) f(S; T )dS; (2.9) @K K @2C = P (t; T )f(K; T ); (2.10) @K2 @C Z 1 @ = −rT C + P (t; T ) (ST − K) [f(S; T )]dS: (2.11) @T K @T @f Substituting the Fokker-Planck equation for @t at t=T, we have: Z 1 2 @C @ @ 2 2 + rT C = P (t; T ) (ST − K)(− [µT Sf(S; T )] + 2 σ S f(S; T ) )dS: (2.12) @T K @S @S We need to evaluate two integrals from the above equation: Z 1 @ I1 = µT (ST − K) [Sf(S; T )] dS; (2.13) K @S Z 1 2 @ 2 2 I2 = (ST − K) 2 σ S f(S; T ) )dS: (2.14) K @S In order to solve these two integrals, we first need to utilize two identities, namely Z 1 C K @C ST f(S; T )dS = − ; (2.15) K P (t; T ) P (t; T ) @K 1 @2C f(K; T ) = : (2.16) P (t; T ) @K2 These two integrals can be easily evaluated by using these two identities and the integral by parts technique. Finally, we have obtained the solutions of the two integrals: −µ C µ K @C I = T + T ; (2.17) 1 P (t; T ) P (t; T ) @K 3 σ2K2 @2C I = : (2.18) 2 P (t; T ) @K2 Plugging them back to equation(2.12), we have achieved the Dupire Local Volatility PDE. Moreover, we have solved a function of local volatility. Assuming zero interest rate and zero dividend yield, we have: @C σ2(K; T ) = @T : (2.19) 1 2 @2C 2 K @K2 A limitation for local volatility is that it makes the assumption that the volatility must has something to do with the stock price and time. Research works find that the volatility is more likely to move in a random process. So Heston model was introduced, in which the volatility follows stochastic process. 3 Heston model 3.1 Heston Dynamics The Heston model assumes that St follows a Black-Sholes type stochastic process, while the stochastic variance vt follows a Cox, Ingersoll, Ross process. Thus p dSt = rStdt + vtStdW1;t (3.20) p dvt = κ(θ − vt)dt + σ vtdW2;t E[dW1;tdW2;t] = ρ dt 3.2 The Heston PDE Under the Heston model, the market is no longer complete as we assumed in the Black-Sholes model. To replicate an option, we need to construct a portfolio consisting of one option V = V (S; v; t), ∆ units of the stock S, and φ units of another option U = U(S; v; t). The value of the portfolio is: Π = V + ∆ S + φ U: Assuming the portfolio is self-financing, the change in the value of the portfolio is: dΠ = dV + ∆ dS + φ dU: (3.21) Apply It^o'sLemma to dV and dU, differentiating with respect to the variables t; S and v. Plug the results into (3.21), we get: n@V 1 @2 V @2 V 1 @2 V o dΠ = + vS2 + ρσ vS + σ2v dt+ @ t 2 @S2 @ v@ S 2 @ v2 n@U 1 @2 U @2 U 1 @2 U o φ + vS2 + ρσ vS + σ2v dt+ (3.22) @ t 2 @S2 @ v@ S 2 @ v2 n@V @U o n@V @U o + φ + ∆ dS + + φ dv: @S @S @ v @ v In order for the portfolio to be hedged against movements and volatility in the stock, the last two terms of equation (3.22) containing dS and dv must be zero. Thus the hedge parameters are: @V @ v φ = − @U ; @ v (3.23) @U @V ∆ = −φ − : @S @S 4 Plug the values in equation (3.23) into equation (3.22) gives us a form of dΠ without unknown parameters, which we write in a simplified form as dΠ = (A + φ B)dt. On the other hand, the portfolio must earn the risk free rate, r. Hence dΠ = rΠ dt. Combining the two equations of dΠ, we get: @V @U A − rV + rS @S B − rU + rS @S @V = @U ; (3.24) @ v @ v where @V 1 2 @2 V @2 V 1 2 @2 V • A = @ t + 2 vS @S2 + ρσ vS @ v@ S + 2 σ v @ v2 , @U 1 2 @2 U @2 U 1 2 @2 U • B = @ t + 2 vS @S2 + ρσ vS @ v@ S + 2 σ v @ v2 : By observation, both sides of equation (3.24) can be written as a function f(S; v; t) of S, v, and t. Following Heston assumption, specify the function as f(S; v; t) = −κ(θ − v) + λ(S; v; t), where λ(S; v; t) is the price of volatility risk.
Details
-
File Typepdf
-
Upload Time-
-
Content LanguagesEnglish
-
Upload UserAnonymous/Not logged-in
-
File Pages10 Page
-
File Size-