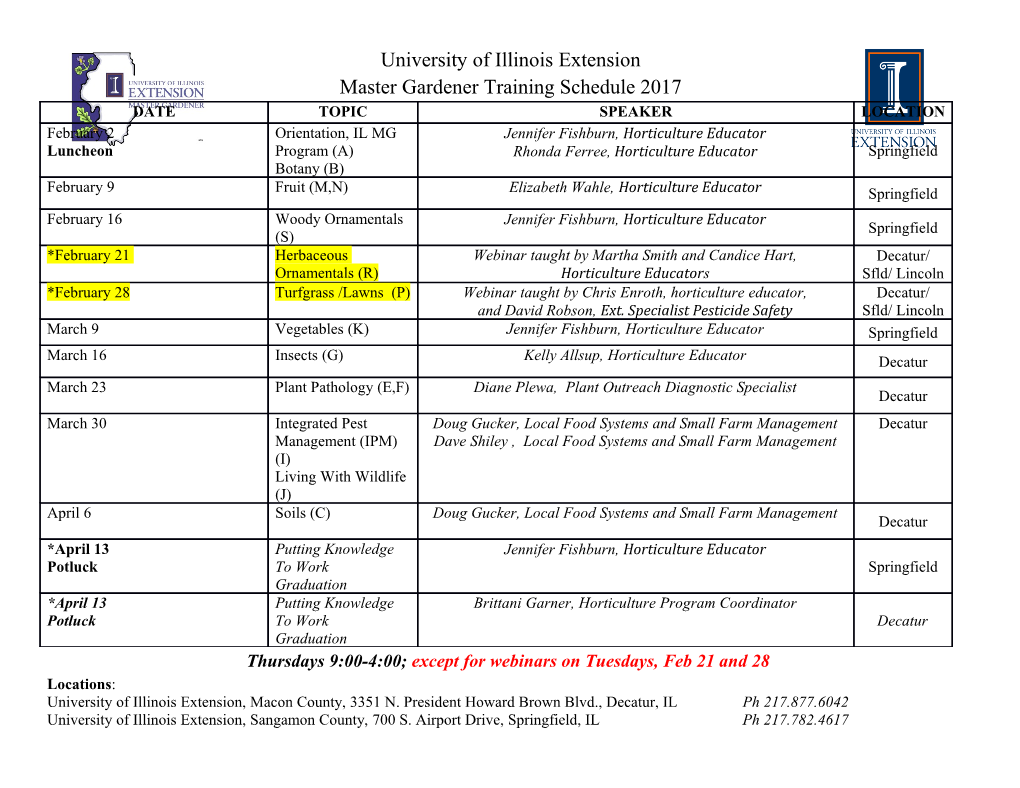
J. Virtamo 38.3143 Queueing Theory / Continuous Distributions 1 CONTINUOUS DISTRIBUTIONS Laplace transform (Laplace-Stieltjes transform) Definition The Laplace transform of a non-negative random variable X 0 with the probability density ≥ function f(x) is defined as st sX st f ∗(s)= ∞ e− f(t)dt = E[e− ] = ∞ e− dF (t) alsodenotedas (s) Z0 Z0 LX Mathematically it is the Laplace transform of the pdf function. • In dealing with continuous random variables the Laplace transform has the same role as • the generating function has in the case of discrete random variables. s – if X is a discrete integer-valued ( 0) r.v., then f ∗(s)= (e− ) ≥ G J. Virtamo 38.3143 Queueing Theory / Continuous Distributions 2 Laplace transform of a sum Let X and Y be independent random variables with L-transforms fX∗ (s) and fY∗ (s). s(X+Y ) fX∗ +Y (s) = E[e− ] sX sY = E[e− e− ] sX sY = E[e− ]E[e− ] (independence) = fX∗ (s)fY∗ (s) fX∗ +Y (s)= fX∗ (s)fY∗ (s) J. Virtamo 38.3143 Queueing Theory / Continuous Distributions 3 Calculating moments with the aid of Laplace transform By derivation one sees d sX sX f ∗0(s)= E[e− ]=E[ Xe− ] ds − Similarly, the nth derivative is n (n) d sX n sX f ∗ (s)= E[e− ] = E[( X) e− ] dsn − Evaluating these at s = 0 one gets E[X] = f ∗0(0) − 2 E[X ] =+f ∗00(0) . n n (n) E[X ] = ( 1) f ∗ (0) − J. Virtamo 38.3143 Queueing Theory / Continuous Distributions 4 Laplace transform of a random sum Consider the random sum Y = X + + X 1 ··· N where the Xi are i.i.d. with the common L-transform fX∗ (s) and N 0 is a integer-valued r.v. with the generating function (z). ≥ GN sY fY∗ (s) = E[e− ] sY = E[E e− N ] (outer expectation with respect to variations of N) | s(X1+ +XN ) = E[E e− ··· N ] (in the inner expectation N is fixed) | s(X1) s(XN ) = E[E[e− ] E[e− ]] (independence) ··· N = E[(fX∗ (s)) ] N = (f ∗ (s)) (by the definition E[z ]= (z)) GN X GN J. Virtamo 38.3143 Queueing Theory / Continuous Distributions 5 Laplace transform and the method of collective marks We give for the Laplace transform sX f ∗(s)=E[e− ], X 0, the following ≥ Interpretation: Think of X as representing the length of an interval. Let this interval be subject to a Poissonian marking process with intensity s. Then the Laplace transform f ∗(s) is the probability that there are no marks in the interval. P X has no marks = E[P X has no marks X ] (totalprobability) { } { | } = E[P the number of events in the interval X is 0 X ] { | } sX = E[e− ] = f ∗(s) intensiteettis n (sX) sX P there are n events in the interval X X = e− { | } n! sX ì í î P the number of events in the interval X is 0 X = e− X { | } J. Virtamo 38.3143 Queueing Theory / Continuous Distributions 6 Method of collective marks (continued) Example: Laplace transform of a random sum Y = X + + X , where 1 ··· N X X X , common L-transform f (s) 1 2 N ∗ ∼ ∼···∼ N is a r.v. with generating function N (z) G intensiteettis f ∗ (s) = P none of the subintervals of Y is marked Y { } ... = N ( f ∗ (s) ) X X X G X 1 2 N probability| {z } that a single subinterval has no marks probability| {z that none} of the subintervals is marked J. Virtamo 38.3143 Queueing Theory / Continuous Distributions 7 Uniform distribution X ∼ U(a, b) The pdf of X is constant in the interval (a,b): 1 a<x<b b a f(x)= − 0 elsewhere i.e. the value X is drawn randomly in the interval (a,b). f(x) F(x) 1 ab a b + a + b ∞ E[X] = Z xf(x)dx = −∞ 2 2 + a + b 2 (b a) ∞ V[X] = Z x f(x)dx = − −∞ − 2 12 J. Virtamo 38.3143 Queueing Theory / Continuous Distributions 8 Uniform distribution (continued) Let U ,...,U be independent uniformly distributed random variables, U U(0, 1). 1 n i ∼ The number of variables which are x (0 x 1)) is Bin(n,x) • ≤ ≤ ≤ ∼ – the event U x defines a Bernoulli trial where the probability of success is x { i ≤ } Let U ,...,U be the ordered sequence of the values. • (1) (n) Define further U(0) = 0 and U(n+1) = 1. It can be shown that all the intervals are identically distributed and P U U >x = (1 x)n i =1,...,n { (i+1) − (i) } − – for the first interval U U = U the result is obvious because U = min(U ,...,U ) (1)− (0) (1) (1) 1 n J. Virtamo 38.3143 Queueing Theory / Continuous Distributions 9 Exponential distribution X ∼ Exp(λ) (Note that sometimes the shown parameter is 1/λ, i.e. the mean of the distribution) X is a non-negative continuous random variable with the cdf λx F(x) 1 e x 0 1 − F (x)= − ≥ 0 x< 0 x and pdf f(x) λ λe λx x 0 − f(x)= ≥ 0 x< 0 x Example: interarrival time of calls; holding time of call J. Virtamo 38.3143 Queueing Theory / Continuous Distributions 10 Laplace transform and moments of exponential distribution The Laplace transform of a random variable with the distribution Exp(λ) is st λt λ f ∗(s)= ∞ e− λe− dt = Z0 · λ + s With the aid of this one can calculate the moments: λ 1 E[X] = f ∗0(0) = 2 = (λ+s) s=0 λ − 2 2λ 2 E[X ] = +f ∗00(0) = 3 = 2 (λ+s) s=0 λ 2 2 1 V[X] = E[X ] E[X] = 2 − λ 1 1 E[X]= V[X]= λ λ2 J. Virtamo 38.3143 Queueing Theory / Continuous Distributions 11 The memoryless property of exponential distribution Assume that X Exp(λ) represents e.g. the duration of a call. ∼ What is the probability that the call will last at least time x more given that it has already lasted the time t: P X > t + x,X >t P X > t + x X > t = { } { | } P X > t { } P X > t + x = { } P X > t λ({t+x) } e− λx = λt = e− = P X>x e− { } P X > t + x X > t = P X>x { | } { } exp(- λ t) exp(- λ (t-u)) The distribution of the remaining duration of the call • does not at all depend on the time the call has already lasted u t Has the same Exp(λ) distribution as the total duration • of the call. J. Virtamo 38.3143 Queueing Theory / Continuous Distributions 12 Example of the use of the memoryless property ´ A queueing system has two servers. The service times are ¨ assumed to be exponentially distributed (with the same pa- ´ rameter). Upon arrival of a customer ( ) both servers are occupied ( ) but there are no other waiting customers. × The question: what is the probability that the customer ( ) will be the last to depart from the system? ¨ The next event in the system is that either of the customers ( ) being served departs and the customer enters ( ) the × ´ freed server. By the memoryless property, from that point on the (remaining) service times of both cus- tomers ( ) and ( ) are identically (exponentially) distributed. × The situation is completely symmetric and consequently the probability that the customer ( ) is the last one to depart is 1/2. J. Virtamo 38.3143 Queueing Theory / Continuous Distributions 13 The ending probability of an exponentially distributed interval Assume that a call with Exp(λ) distributed duration has lasted the time t. What is the probability that it will end in an infinitesimal interval of length h? P X t + h X > t = P X h (memoryless) { ≤ | } { ≤ } λh = 1 e− − = 1 (1 λh + 1(λh)2 ) − − 2 −··· = λh + o(h) The ending probability per time unit = λ (constant!) J. Virtamo 38.3143 Queueing Theory / Continuous Distributions 14 The minimum and maximum of exponentially distributed random variables Let X X Exp(λ) (i.i.d.) 1 ∼···∼ n ∼ The tail distribution of the minimum is P min(X ,...,X ) >x = P X >x P X >x (independence) { 1 n } { 1 }··· { n } λx n nλx = (e− ) = e− The minimum obeys the distribution Exp(nλ). n parallel processes each of which ends with The ending intensity of the minimum = nλ intensity λ independent of the others The cdf of the maximum is X1 λx n X2 P max(X1,...,Xn) x = (1 e− ) { ≤ } − X3 The expectation can be deduced by inspecting the figure X4 X 1 1 1 5 í í í í í E[max(X ,...,X )] = + + + ì î ì î ì î ì î ì î 1 n nλ (n 1)λ ··· λ − ~Exp(nl ) ~Exp(l ) ~Exp((n-1)l ) ~Exp((n-2)l ) J. Virtamo 38.3143 Queueing Theory / Continuous Distributions 15 Erlang distribution X ∼ Erlang(n, λ) Also denoted Erlang-n(λ). X is the sum of n independent random variables with the distribution Exp(λ) X = X + + X X Exp(λ) (i.i.d.) 1 ··· n i ∼ The Laplace transform is λ n f ∗(s) = ( ) λ + s By inverse transform (or by recursively convoluting the density function) one obtains the pdf of the sum X n 1 (λx) − λx f(x) = λe− x 0 (n 1)! ≥ − J. Virtamo 38.3143 Queueing Theory / Continuous Distributions 16 Erlang distribution (continued): gamma distribution The formula for the pdf of the Erlang distribution can be generalized, from the integer param- eter n, to arbitrary real numbers by replacing the factorial (n 1)! by the gamma function − Γ(n): p 1 (λx) − λx f(x) = λe− Gamma(p, λ) distribution Γ(p) Gamma function Γ(p) is defined by By partial integration it is easy to see that when Γ(p)= ∞ e uup 1du Z0 − − p is an integer then, indeed, Γ(p) = (p 1)! − 0.5 n=1 The expectation and variance are n times those 0.4 of the Exp(λ) distribution: n=2 0.3 n=3 n=4 n n n=5 E[X]= V[X]= 0.2 λ λ2 0.1 0 2 4 6 8 10 J.
Details
-
File Typepdf
-
Upload Time-
-
Content LanguagesEnglish
-
Upload UserAnonymous/Not logged-in
-
File Pages19 Page
-
File Size-