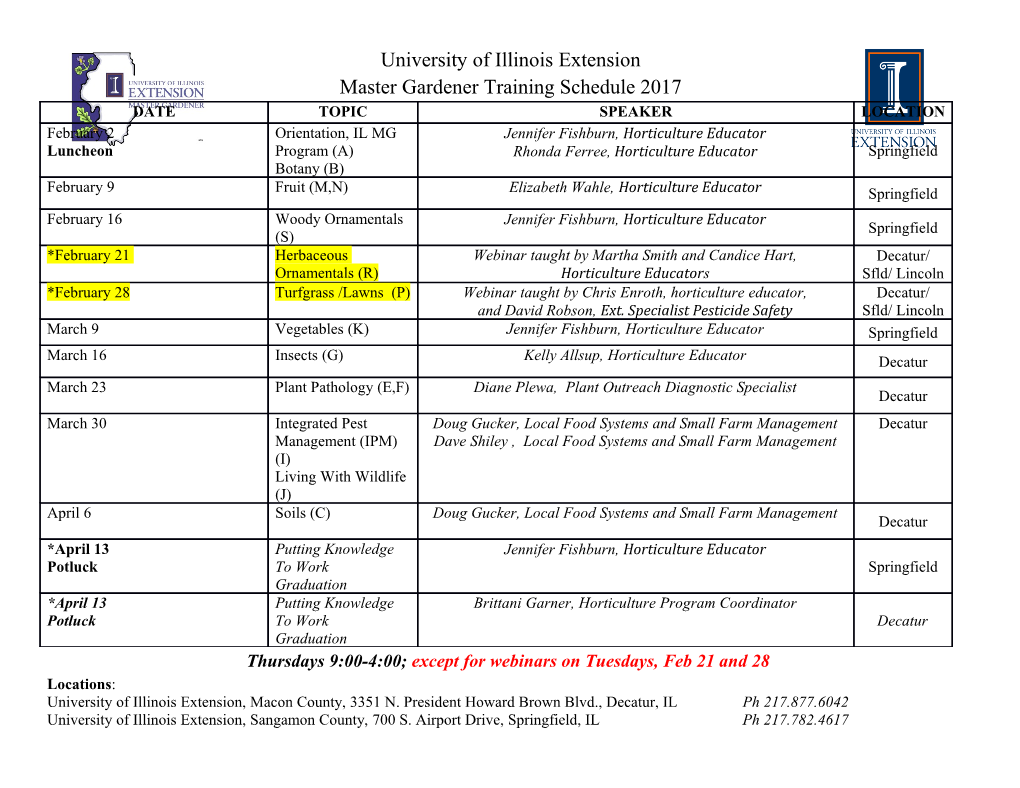
600.664: Randomized Algorithms Professor: Rao Kosaraju Johns Hopkins University Scribe: Your Name Randomized Algorithms Week 1: Probability Concepts Rao Kosaraju 1.1 Motivation for Randomized Algorithms In a randomized algorithm you can toss a fair coin as a step of computation. Alternatively a bit taking values of 0 and 1 with equal probabilities can be chosen in a single step. More generally, in a single step an element out of n elements can be chosen with equal probalities (uniformly at random). In such a setting, our goal can be to design an algorithm that minimizes run time. Randomized algorithms are often advantageous for several reasons. They may be faster than deterministic algorithms. They may also be simpler. In addition, there are problems that can be solved with randomization for which we cannot design efficient deterministic algorithms directly. In some of those situations, we can design attractive deterministic algoirthms by first designing randomized algorithms and then converting them into deter- ministic algorithms by applying standard derandomization techniques. Deterministic Algorithm: Worst case running time, i.e. the number of steps the algo- rithm takes on the worst input of length n. Randomized Algorithm: 1) Expected number of steps the algorithm takes on the worst input of length n. 2) w.h.p. bounds. As an example of a randomized algorithm, we now present the classic quicksort algorithm and derive its performance later on in the chapter. We are given a set S of n distinct elements and we want to sort them. Below is the randomized quicksort algorithm. Algorithm RandQuickSort(S = fa1; a2; ··· ; ang If jSj ≤ 1 then output S; else: Choose a pivot element ai uniformly at random (u.a.r.) from S Split the set S into two subsets S1 = fajjaj < aig and S2 = fajjaj > aig by comparing each aj with the chosen ai Recurse on sets S1 and S2 Output the sorted set S1 then ai and then sorted S2. end Algorithm For this the algorithm we will establish that the expected speed on any input of length is no more than ≥ 2n ln n . In addition, we will establish that the probability that the algorithm will take more than 1 600.664: Randomized Algorithms Professor: Rao Kosaraju Johns Hopkins University Scribe: Your Name 1 12 ln n steps is no more than n2 . Hence if n = 1000 and if we run the a algorithm for a million (10002) times, it will run for more than 12000 ln 1000 at most once. Before we can design and analyze randomized algorithms, however, it is important to review the basic concepts in probability theory. 1.2 Probability Spaces and Events When we conduct a random experiment several possible outcomes may occur. Definition 1 (Probability Space). A probability space consists of a universe Ω, a collection of subsets of Ω known as events, and a function, P , over the events that satisfies the following properties: 1. Ω is an event. 2. If E is an event then E¯ is an event. 3. If E1;E2;:::;Ek are events then [iEi is an event. (The union can be over a countable set of events.) 4. For each event E, P (E) ≥ 0. 5. P (Ω) = 1. P 6. If E1;E2;:::;Ek are disjoint events then P ([iEi) = i P (Ei). Note that if E1;E2 are events E1 \ E2 is also an event since E1 \ E2 = E1 \ E2 = E1 [ E2. Definition 2 (Conditional Probability). The conditional probability of event E1 given that event E2 has occurred, written P (E1jE2), is defined as P (E1 \ E2) P (E1jE2) = (1) P (E2) At times, we write P (E1 \ E2) as P (E1;E2). More generally, we write P (E1 \ E2 \···\ En) as P (E1;E2; ··· ;En) Observation: P(E1 \ E2 \···\ En) = P (E1jE2 \···\ En) P (E2jE3 \···\ En) ··· P (En−1jEn) P (En) : Definition 3 (Independence). Events E1 and E2 are independent if P (E1 \ E2) = P (E1) P (E2) This can also be stated as: P (E1jE2) = P (E1) or P (E2jE1) = P (E2) 2 600.664: Randomized Algorithms Professor: Rao Kosaraju Johns Hopkins University Scribe: Your Name Definition 4 (Pairwise independence). Events E1;E2;:::;En are pairwise independent if (8i; j; i 6= j)(Ei and Ej are independent ). Definition 5 (k-wise independence). Events E1;E2;:::;En are k-wise independent 2 ≤ k ≤ n, if for every 2 ≤ k1 ≤ k and distinct indices i1; : : : ; ik1 P E \ E \ ::: \ E = Πk1 P E ; i1 i2 ik1 j=1 ij Or equivalently P E jE \ ::: \ E = P (E ) : i1 i2 ik1 i1 1.3 Discrete Random Variables and Expectation Definition 6 (Discrete Random Variable). A discrete random variable is a function X : Ω ! a countable subset of R such that for each a in the subset fijX(i) = ag is an event. We write P (fijX (i) = ag) in the shorthand form P (X = a). The probability function P is known as the probability mass function. Definition 7 (Expectation). The expectation, E (X), of the random variable X is defined as X E (X) = aP (X = a) (2) a E(X) is usually denoted by µX or simply µ when X is understood. Definition 8 (Joint Mass Function). If X1;:::;Xn are random variables, then the joint mass function P (X1 = a1;:::;Xn = an) is defined as the probability of the event P ((X1 = a1) \ (X2 = a2) \ ::: \ (Xn = an)). The probability expression can also be written as pX1;X2;:::;Xn (a1; a2; : : : ; an). 1 We may find P (X1 = a1) as follows: X P(X1 = a1) = P(X1 = a1;X2 = a2;:::;Xn = an) (3) a2;:::;an Definition 9 (Independence of Random Variables). Random variables X1;:::;Xn are said to be independent if for every distinct i1; : : : ; im and for every a1; : : : ; am: m Y P (Xi1 = a1;Xi2 = a2;:::;Xim = am) = P Xij = aj j=1 1 Of course this generalizes to any Xi and ai, but we write it as X1 and a1 to simplify the notation. 3 600.664: Randomized Algorithms Professor: Rao Kosaraju Johns Hopkins University Scribe: Your Name Definition 10 (Pairwise Independence). Random variables X1;X2; :::; Xn are pairwise in- dependent if for every distinct i and j, Xi and Xj are independent. Definition 11 (k-wise Independence). Random variables X1;X2; :::; Xn are k-wise inde- pendent, 2 ≤ k ≤ n, if for every distinct i ; i ; :::; i , 2 ≤ k ≤ k, X ;X ; :::; X are 1 2 k1 1 i1 i2 ik1 independent. Example 1. 4 balls are thrown independently and u.a.r. into 5 bins. What is the probability that 2 balls fall into bin 1? We define our probability space to be Ω = f(i1; i2; i3; i4)jij 2 f1; 2; 3; 4; 5gg. The value ij specifies the bin into which ball j falls. Since each ball is thrown independently and u.a.r., 1 for every i1; i2; i3; i4, P (i1; i2; i3; i4) = 4 . Define a r.v. X :Ω ! f0; 1; 2; 3; 4g s.t. for every 5 ( P 1 if k = 1 i1; i2; i3; i4, X((i1; i2; i3; i4)) = f f(ij), in which f(k) = j 0 otherwise. Note that r.v. X stands for the number of balls that fall into bin 1. We are interested in 0 P(X = 2). For any choice of 2 j s s.t. ij = 1, the other positions can be chosen as any 0 0 value from f2; 3; 4; 5g. Hence the number of (i1; i2; i3; i4) s s.t. exactly 2 of the ijs are 1's 4 2 4 2 1 4 1 2 4 2 is 2 4 . Hence P (X = 2) is 2 4 54 which is given by 2 5 5 . Example 2. Let X be the number of balls that fall into bin 1. What is the value of E (X)? Using the same method as above, we have 4 4 P(X = 0) = 5 4 1 4 3 P(X = 1) = 1 5 5 4 1 2 4 2 P(X = 2) = 2 5 5 4 1 3 4 P(X = 3) = 3 5 5 1 4 P(X = 4) = 5 4 4 4 1 4 3 4 1 2 4 2 4 1 3 4 1 4 4 Then E (X) = 0 5 + 1 1 5 5 + 2 2 5 5 + 3 3 5 5 + 4 5 = 5 . This result can also be justified by appealing to our intuition: The expected number of balls that fall into any of the 5 bins should be the same. Since there a total of 4 balls, for any bin the expected number of balls should be one fifth of 4. 1.4 Functions of Random Variables Definition 12 (Function of a Random Variable). If X1;:::;Xk are random variables and f is a function, then f (X1;:::;Xk) is a random variable such that X P (f (X1;X2;:::;Xk) = a) = P (X1 = a1;:::Xk = ak) (4) a1; : : : ; ak s.t. f (a1; : : : ; ak) = a 4 600.664: Randomized Algorithms Professor: Rao Kosaraju Johns Hopkins University Scribe: Your Name Theorem 1. X E (f (X1;:::;Xk)) = f (a1; : : : ; ak) P (X1 = a1;:::;Xk = ak) a1;:::;ak X2 X 1 2 3 Example 3. Compute E(X + X ) for the joint mass function given by 1 1 2 1 .1 .1 .2 2 .3 .1 .2 We compute E(X1;X2) by two different methods: By applying the definition and by applying the above theorem. Direct Computation: P (X1 + X2 = 2) = P (X1 = 1;X2 = 1) = :1 P (X1 + X2 = 3) = P (X1 = 1;X2 = 2) + P (X1 = 2;X2 = 1) = :1 + :3 = :4 P (X1 + X2 = 4) = P (X1 = 1;X2 = 3) + P (X1 = 2;X2 = 2) = :2 + :1 = :3 P (X1 + X2 = 5) = P (X1 = 2;X2 = 3) = :2 Hence, E(X1 + X2) = 2(:1) + 3(:4) + 4(:3) + 5(:2) = 3:6.
Details
-
File Typepdf
-
Upload Time-
-
Content LanguagesEnglish
-
Upload UserAnonymous/Not logged-in
-
File Pages14 Page
-
File Size-