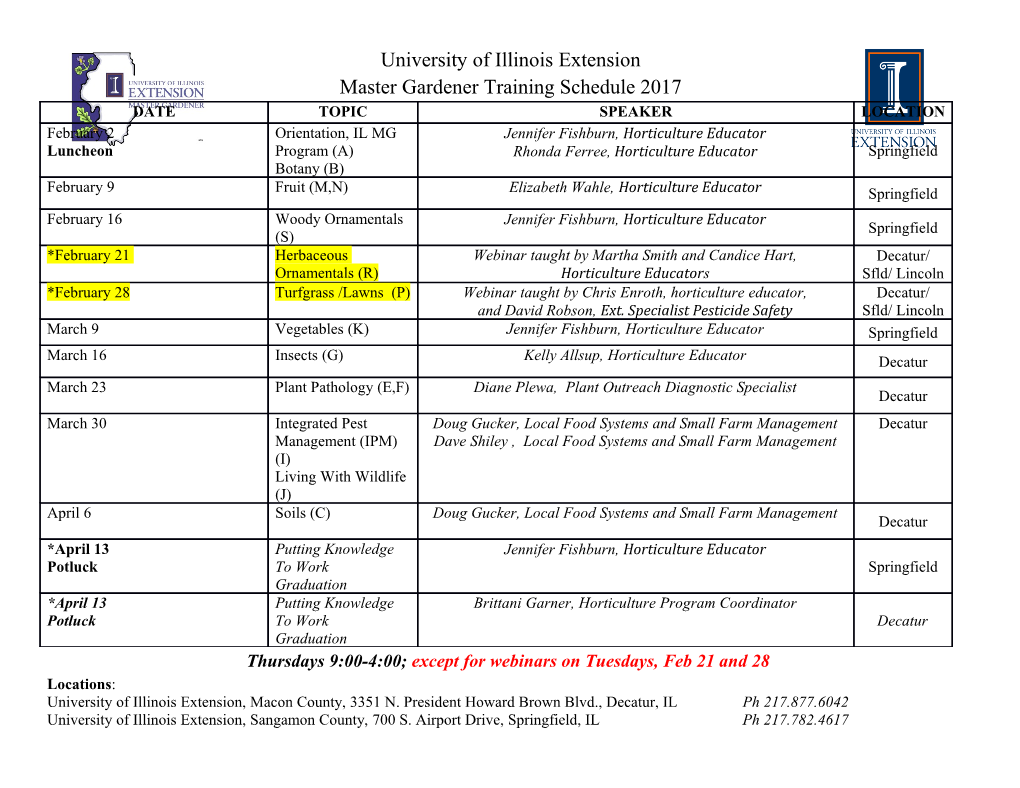
Preliminary Test of a Real-Time, Interactive Silent Speech Interface Based on Electromagnetic Articulograph Jun Wang Ashok Samal Jordan R. Green Dept. of Bioengineering Dept. of Computer Science & Dept. of Communication Sci- Callier Center for Communi- Engineering ences & Disorders cation Disorders University of Nebraska- MGH Institute of Health Pro- University of Texas at Dallas Lincoln fessions [email protected] [email protected] [email protected] treatment options for these individuals including Abstract (1) esophageal speech, which involves oscillation of the esophagus and is difficult to learn; (2) tra- A silent speech interface (SSI) maps articula- cheo-esophageal speech, in which a voice pros- tory movement data to speech output. Alt- thesis is placed in a tracheo-esophageal puncture; hough still in experimental stages, silent and (3) electrolarynx, an external device held on speech interfaces hold significant potential the neck during articulation, which produces a for facilitating oral communication in persons robotic voice quality (Liu and Ng, 2007). Per- after laryngectomy or with other severe voice impairments. Despite the recent efforts on si- haps the greatest disadvantage of these ap- lent speech recognition algorithm develop- proaches is that they produce abnormal sounding ment using offline data analysis, online test speech with a fundamental frequency that is low of SSIs have rarely been conducted. In this and limited in range. The abnormal voice quality paper, we present a preliminary, online test of output severely affects the social life of people a real-time, interactive SSI based on electro- after laryngectomy (Liu and Ng, 2007). In addi- magnetic motion tracking. The SSI played tion, the tracheo-esophageal option requires an back synthesized speech sounds in response additional surgery, which is not suitable for eve- to the user’s tongue and lip movements. ry patient (Bailey et al., 2006). Although re- Three English talkers participated in this test, search is being conducted on improving the where they mouthed (silently articulated) phrases using the device to complete a voice quality of esophageal or electrolarynx phrase-reading task. Among the three partici- speech (Doi et al., 2010; Toda et al., 2012), new pants, 96.67% to 100% of the mouthed assistive technologies based on non-audio infor- phrases were correctly recognized and corre- mation (e.g., visual or articulatory information) sponding synthesized sounds were played af- may be a good alternative approach for providing ter a short delay. Furthermore, one participant natural sounding speech output for persons after demonstrated the feasibility of using the SSI laryngectomy. for a short conversation. The experimental re- Visual speech recognition (or automatic lip sults demonstrated the feasibility and poten- reading) typically uses an optical camera to ob- tial of silent speech interfaces based on elec- tain lip and/or facial features during speech (in- tromagnetic articulograph for future clinical applications. cluding lip contour, color, opening, movement, etc.) and then classify these features to speech units (Meier et al., 2000; Oviatt, 2003). Howev- 1 Introduction er, due to the lack of information from tongue, Daily communication is often a struggle for per- the primary articulator, visual speech recognition sons who have undergone a laryngectomy, a sur- (i.e., using visual information only, without gical removal of the larynx due to the treatment tongue and audio information) may obtain a low of cancer (Bailey et al., 2006). In 2013, about accuracy (e.g., 30% - 40% for phoneme classifi- 12,260 new cases of laryngeal cancer were esti- cation, Livescu et al., 2007). Furthermore, Wang mated in the United States (American Cancer and colleagues (2013b) have showed any single Society, 2013). Currently, there are only limited tongue sensor (from tongue tip to tongue body 38 Proceedings of the 5th Workshop on Speech and Language Processing for Assistive Technologies (SLPAT), pages 38–45, Baltimore, Maryland USA, August 26 2014. c 2014 Association for Computational Linguistics Figure 1. Design of the real-time silent speech interface. back on the midsagittal line) encodes significant- based SSIs have been tested online with multiple ly more information in distinguishing phonemes subjects and encouraging results were obtained than do lips. However, visual speech recognition in a phrase reading task where the subjects were is well suited for applications with small- asked to silently articulate sixty phrases (Denby vocabulary (e.g., a lip-reading based command- et al., 2011). SSI based on electromagnetic sens- and-control system for home appliance) or using ing has been only tested using offline analysis visual information as an additional source for (using pre-recorded data) collected from single acoustic speech recognition, referred to as audio- subjects (Fagan et al., 2008; Hofe et al., 2013), visual speech recognition (Potamianos et al., although some work simulated online testing 2003), because such a system based on portable using prerecorded data (Wang et al., 2012a, camera is convenient in practical use. In contrast, 2012b, 2013c). Online tests of SSIs using elec- SSIs, with tongue information, have potential to tromagnetic articulograph with multiple subjects obtain a high level of silent speech recognition are needed to show the feasibility and potential accuracy (without audio information). Currently, of the SSIs for future clinical applications. two major obstacles for SSI development are In this paper, we report a preliminary, online lack of (a) fast and accurate recognition algo- test of a newly-developed, real-time, and interac- rithms and (b) portable tongue motion tracking tive SSI based on a commercial EMA. EMA devices for daily use. tracks articulatory motion by placing small sen- SSIs convert articulatory information into text sors on the surface of tongue and other articula- that drives a text-to-speech synthesizer. Although tors (e.g., lips and jaw). EMA is well suited for still in developmental stages (e.g., speaker- the early stage of SSI development because it (1) dependent recognition, small-vocabulary), SSIs is non-invasive, (2) has a high spatial resolution even have potential to provide speech output in motion tracking, (3) has a high sampling rate, based on prerecorded samples of the patient’s and (4) is affordable. In this experiment, partici- own voice (Denby et al., 2010; Green et al., pants used the real-time SSI to complete an 2011; Wang et al., 2009). Potential articulatory online phrase-reading task and one of them had a data acquisition methods for SSIs include ultra- short conversation with another person. The re- sound (Denby et al., 2011; Hueber et al., 2010), sults demonstrated the feasibility and potential of surface electromyography electrodes (Heaton et SSIs based on electromagnetic sensing for future al., 2011; Jorgensen and Dusan, 2010), and elec- clinical applications. tromagnetic articulograph (EMA) (Fagan et al., 2008; Wang et al., 2009, 2012a). 2 Design Despite the recent effort on silent speech in- 2.1 Major design terface research, online test of SSIs has rarely been studied. So far, most of the published work Figure 1 illustrates the three-component design on SSIs has focused on development of silent of the SSI: (a) real-time articulatory motion speech recognition algorithm through offline tracking using a commercial EMA, (b) online analysis (i.e., using prerecorded data) (Fagan et silent speech recognition (converting articulation al., 2008; Heaton et al., 2011; Hofe et al., 2013; information to text), and (c) text-to-speech syn- Hueber et al., 2010; Jorgenson et al., 2010; Wang thesis for speech output. et al., 2009a, 2012a, 2012b, 2013c). Ultrasound- The EMA system (Wave Speech Research 39 Figure 2. Demo of a participant using the silent speech interface. The left picture illustrates the coordinate system and sensor locations (sensor labels are described in text); in the right picture, a participant is using the silent speech interface to finish the online test. system, Northern Digital Inc., Waterloo, Canada) phrases into the system through the GUI. Adding was used to track the tongue and lip movement a new phrase in the vocabulary is done in two in real-time. The sampling rate of the Wave sys- steps. The user (the patient) first enters the tem was 100 Hz, which is adequate for this ap- phrase using a keyboard (keyboard input can also plication (Wang et al., 2012a, 2012b, 2013c). be done by an assistant or speech pathologist), The spatial accuracy of motion tracking using and then produces a few training samples for the Wave is 0.5 mm (Berry, 2011). phrase (a training sample is articulatory data la- The online recognition component recognized beled with a phrase). The system automatically functional phrases from articulatory movements re-trains the recognition model integrating the in real-time. The recognition component is mod- newly-added training samples. Users can delete ular such that alternative classifiers can easily invalid training samples using the GUI as well. replace and be integrated into the SSI. In this preliminary test, recognition was speaker- 2.3 Real-time data processing dependent, where training and testing data were The tongue and lip movement positional data from the same speakers. obtained from the Wave system were processed The third component played back either pre- in real-time prior to being used for recognition. recorded or synthesized sounds using a text-to- This included the calculation of head- speech synthesizer (Huang et al., 1997). independent positions of the tongue and lip sen- sors and low pass filtering for removing noise. 2.2 Other designs The movements of the 6 DOF head sensor A graphical user interface (GUI) is integrated were used to calculate the head-independent into the silent speech interface for ease of opera- movements of other sensors. The Wave system tion. Using the GUI, users can instantly re-train represents object orientation or rotation (denoted the recognition engine (classifier) when new by yaw, pitch, and roll in Euler angles) in qua- training samples are available.
Details
-
File Typepdf
-
Upload Time-
-
Content LanguagesEnglish
-
Upload UserAnonymous/Not logged-in
-
File Pages8 Page
-
File Size-