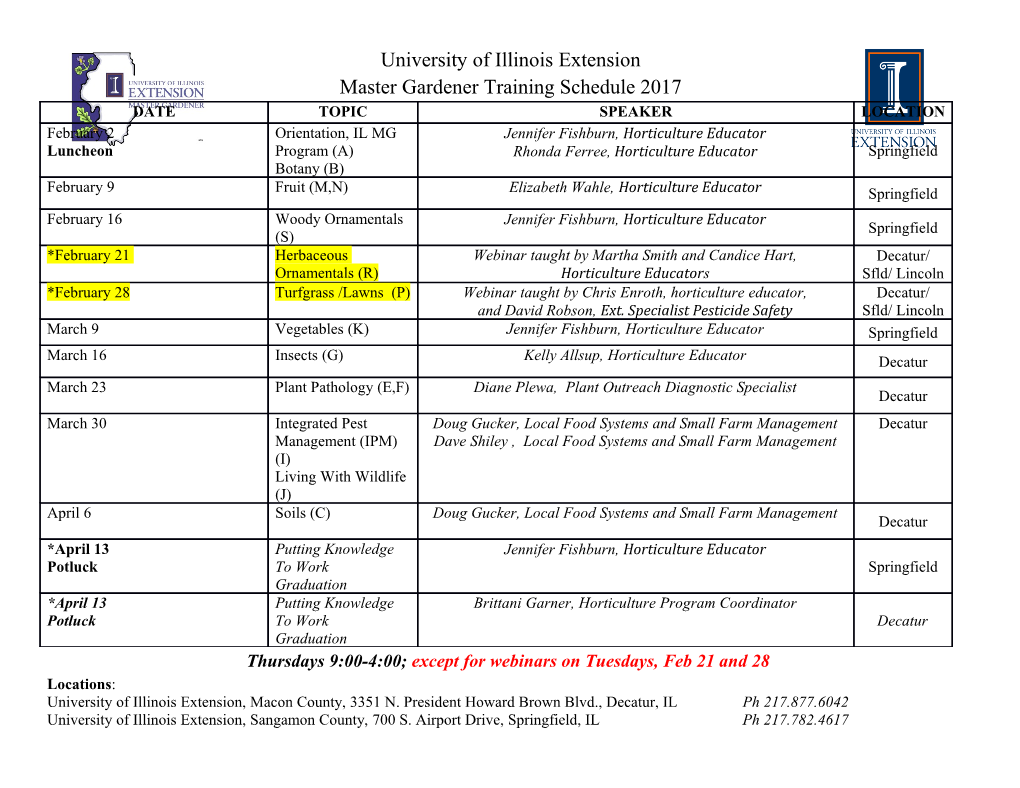
Math 22 – Linear Algebra and its applications - Lecture 22 - Instructor: Bjoern Muetzel GENERAL INFORMATION ▪ Office hours: Tu 1-3 pm, Th, Sun 2-4 pm in KH 229 Tutorial: Tu, Th, Sun 7-9 pm in KH 105 ▪ Homework 7: due Wednesday at 4 pm outside KH 008 ▪ Thursday: x-hour will be a lecture 6 Orthogonality and Least Squares 6.3 ORTHOGONAL PROJECTIONS Summary: 1.) We can find the orthogonal projection of a vector y in ℝ푛 onto a subspace W. This allows us to approximate the vector y with a vector 풚ෝ in W. 2.) We will see that, in a certain sense, this is the best approximation of a vector y with a vector in W. GEOMETRIC INTERPRETATION THE ORTHOGONAL DECOMPOSITION THEOREM ▪ Theorem 8: Let W be a subspace of ℝ푛. Then each 푦 in ℝ푛 can be written uniquely in the form 푦 = 풚ෝ + 풛 . (1) in W in 푊⊥ ▪ In fact, if 푢1, 푢2, … , 푢푝 is any orthogonal basis of W, then 풚∙풖ퟏ 풚∙풖풑 풚ෝ = 풖ퟏ + ⋯ + 풖풑 (2) or 풖ퟏ∙풖ퟏ 풖풑∙풖풑 풚ෝ = 푝푟표푗풖ퟏ 푦 + ⋯ + 푝푟표푗풖풑 푦 = projW (y) and 풛 = 푦 − 풚ෝ . Note: The vector 풚ෝ = projW (y) is called the orthogonal projection of y onto W. The total projection decomposes into line projections. Picture: Proof of Theorem 8: 1.) This construction is correct a) 푦ො in W: it can be written as a linear combination of basis vectors of W. b) z is in 푊⊥ ▪ We know that 풛 = 풚 − 풚ෝ. Since 푢1 is orthogonal to 푢2, … , 푢푝, it 푦∙푢1 푦∙푢푝 follows from the equation 푦ො = 푢1 + ⋯ + 푢푝 that 푢1∙푢1 푢푝∙푢푝 ▪ Thus z is orthogonal to 푢1. Similarly, z is orthogonal to each 푢푗 in the basis for W. Hence z is orthogonal to every vector in W. That is, z is in 푊⊥. THE ORTHOGONAL DECOMPOSITION THEOREM 2.) Uniqueness of the decomposition: 1 1 3 Example 1: Let 푢1 = 2 , 푢2 = 0 and 푦 = 3 . −1 1 3 1.) Show that 푢1, 푢2 is an orthogonal basis for 푊 = 푆푝푎푛 푢1, 푢2 . 2.) Write 푦 as the sum of a vector 푦ො in W and a vector 푧 in 푊⊥. 3 3.) Draw a picture of 푢1, 푢2, 푦 and 푦ො in ℝ . THE BEST APPROXIMATION THEOREM ▪ Theorem 9: Let W be a subspace of ℝ푛 and y be a vector in ℝ푛. Let 푦ො = 푝푟표푗푊(푦) be the orthogonal projection of y onto W. Then 풚ෝ is the closest point in W to y, i.e. 푦 − 푦ො < 푦 − 푣 for all v in W distinct from 푦ො. Hence 푦 − 푦ො = dist y, W . ▪ The vector 푦ො is called the best approximation to y by elements of W. Note: The distance from y to v, given by 풚 − 풗 , can be regarded as the “error” of using v in place of y. The theorem says that this error is minimized when 풗 = 풚ෝ. Proof of Theorem 9: PROPERTIES OF ORTHOGONAL PROJECTIONS 5 1 −1 ▪ Example 2: Let 푢1 = −2 , 푢2 = 2 and 푦 = −5 . 1 −1 10 ▪ Let 푊 = 푆푝푎푛 푢1, 푢2 . Show that 푢1, 푢2 is an orthogonal basis for 푊. Then find the distance 푦 − 푦ො = dist y, W from y to W. PROPERTIES OF ORTHOGONAL PROJECTIONS ▪ Theorem 10: If 푢1, 푢2, … , 푢푝 is an orthonormal basis for a subspace W of ℝ푛, then 푦ො = 푝푟표푗푊(푦) = 푦 ∙ 푢1 푢1 + 푦 ∙ 푢2 푢2 + ⋯ + 푦 ∙ 푢푝 푢푝 If U = 푢1, 푢2, … , 푢푝 , then 푇 푛 푝푟표푗푊(푦) = 푈푈 푦 for all y in ℝ . ▪ Proof: The first part follows immediately from Theorem 8. For the second part we rewrite the equation in matrix notation. .
Details
-
File Typepdf
-
Upload Time-
-
Content LanguagesEnglish
-
Upload UserAnonymous/Not logged-in
-
File Pages16 Page
-
File Size-