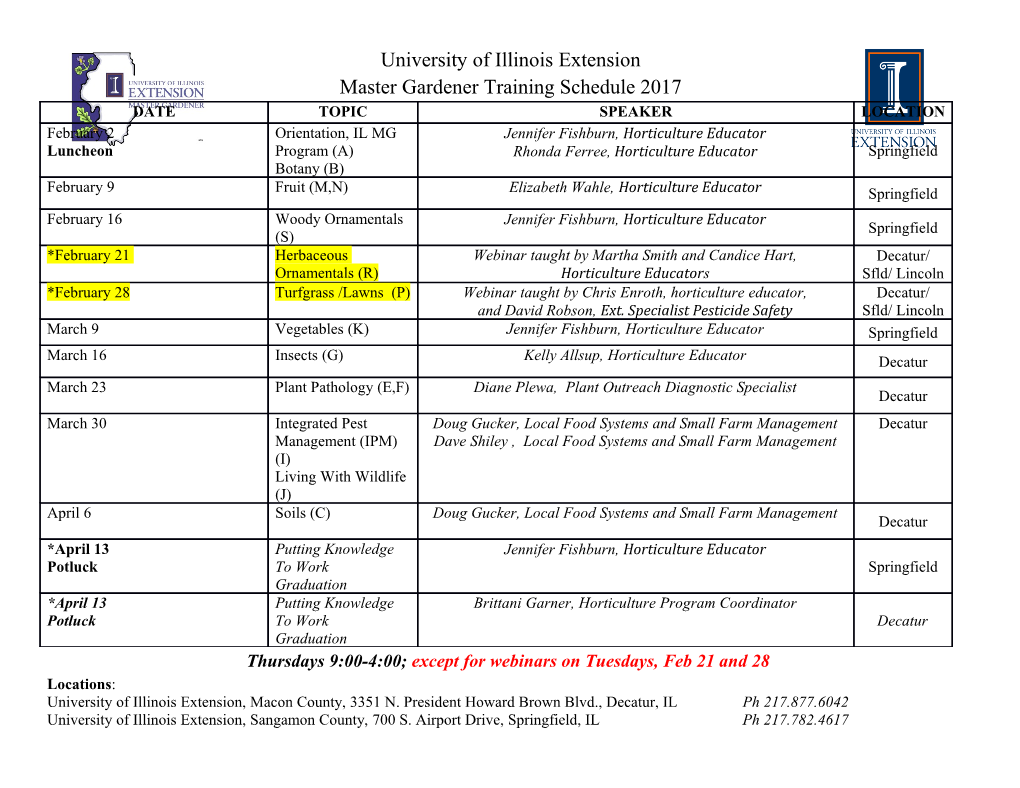
Notes Incorporating Shrub and Snag Specific LiDAR Data into GAP Wildlife Models Teresa J. Lorenz*, Kerri T. Vierling, Jody Vogeler, Jeffrey Lonneker, Jocelyn Aycrigg T.J. Lorenz, K.T. Vierling Department of Fish and Wildlife Sciences, College of Natural Resources, University of Idaho, Moscow, Idaho 83844 J. Vogeler Department of Fish and Wildlife Sciences, College of Natural Resources, University of Idaho, Moscow, Idaho 83844 Present address: Forest Ecosystems and Society, College of Forestry, Oregon State University, Corvallis, Oregon 97331 J. Lonneker, J. Aycrigg Gap Analysis Program, Idaho Cooperative Fish and Wildlife Research Unit, University of Idaho, Moscow, Idaho 83843 Abstract The U.S. Geological Survey’s Gap Analysis Program (hereafter, GAP) is a nationally based program that uses land cover, vertebrate distributions, and land ownership to identify locations where gaps in conservation coverage exist, and GAP products are commonly used by government agencies, nongovernmental organizations, and private citizens. The GAP land-cover designations are based on satellite-derived data, and although these data are widely available, these data do not capture the 3-dimensional vegetation architecture that may be important in describing vertebrate distributions. To date, no studies have examined how the inclusion of snag- or shrub-specific Light Detection and Ranging (LiDAR) data might influence GAP model performance. The objectives of this paper were 1) to assess the performance of the National GAP models and Northwest GAP models with independently collected field data, and 2) to assess whether the inclusion of 3-dimensional vegetation data from LiDAR improved the performance of National GAP and Northwest GAP models. We included only two parameters from the LiDAR data: presence or absence of shrubs and presence or absence of snags $25 cm diameter at breast height. We surveyed for birds at.150 points in a 20,000-ha coniferous forest in northern Idaho and used data for eight shrub- and cavity-nesting species for validation purposes. On a guild level, National GAP models performed only marginally better than Northwest GAP models in correct classification rate, and LiDAR data did not improve vertebrate distribution models. At the scale used in this study, GAP models had poor predictive power and this is important for managers interested in using GAP models for species distributions at scales similar to ours, such as a small park or preserve ,200 km2 in size. Additionally, because the inclusion of LiDAR data did not consistently affect the performance of GAP models, future studies might consider whether LiDAR data affect GAP model performance by examining 1) different spatial scales, 2) different LiDAR metrics, and/or 3) species-specific habitat relationships not currently available in GAP models. Keywords: birds; GAP; Idaho; LiDAR; shrub; snag; species modeling Received: September 27, 2013; Accepted: June 10, 2015; Published Online Early: June 2015; Published: December 2015 Citation: Lorenz TJ, Vierling KT, Vogeler J, Lonneker J, Aycrigg J. 2015. Incorporating shrub and snag specific LiDAR data into GAP wildlife models. Journal of Fish and Wildlife Management 6(2):437–447; e1944-687X. doi: http://dx.doi.org/10. 3996/092013-JFWM-064 Copyright: All material appearing in the Journal of Fish and Wildlife Management is in the public domain and may be reproduced or copied without permission unless specifically noted with the copyright symbol ß. Citation of the source, as given above, is requested. The findings and conclusions in this article are those of the author(s) and do not necessarily represent the views of the U.S. Fish and Wildlife Service. * Corresponding author: [email protected] Journal of Fish and Wildlife Management | www.fwspubs.org December 2015 | Volume 6 | Issue 2 | 437 Assessing GAP Model Performance with LiDAR T.J. Lorenz et al. Introduction examine the accuracy of GAP models, which is important to determine where improvements can be made in the The U.S. Geological Survey’s Gap Analysis Program GAP modeling process (Scott et al. 1996, 2002). (hereafter, GAP) is a nationally based program that uses Land cover is a common denominator in all GAP land cover, vertebrate distributions, and land ownership vertebrate models and is typically derived solely upon to identify locations where gaps in conservation cover- satellite-based data. Although land cover is undoubtedly age exist (e.g., Scott et al. 1993; http://gapanalysis.usgs. an important variable for vertebrates, the two-dimen- gov/). Primarily, GAP has modeled these conservation sional nature of satellite data may be insufficient to gaps for vertebrates using deductive modeling ap- capture important habitat variables for some species proaches, whereby literature and expert opinion are (Vierling et al. 2008). Because LiDAR is a remote-sensing both incorporated into the modeling process (e.g., tool that describes 3-dimensional vertical structure, the Aycrigg et al. 2010). Underlying assumptions of GAP incorporation of LiDAR data may improve our under- are that vertebrate associations with land cover are standing of species distributions at both fine and broad known, land cover is accurately mapped, and land cover spatial scales (Lefsky et al. 2002; Vierling et al. 2008; is mapped at a scale ecologically relevant to the species Bergen et al. 2009). Applications for wildlife habitat in question (Edwards et al. 1996; Boykin et al. 2010). modeling using LiDAR data have increased in recent Data are available from GAP at a national scale years, largely because LiDAR data can reflect ecologically (continental United States; hereafter, USGAP), regional relevant vegetation structure that may otherwise be scale (e.g., northwestern United States states, including difficult to gather through traditional field-based efforts Oregon, Washington, Montana, Idaho, and Wyoming; (e.g., Bradbury et al. 2005; Mu¨ller et al. 2009). hereafter, NWGAP), and for some individual U.S. states The value of LiDAR is apparent from the growing (e.g., Idaho); and each scale uses different numbers and number of research studies that have incorporated types of variables in their distribution models (Aycrigg et LiDAR-derived data in wildlife-habitat models (e.g., Goetz al. 2010, 2013). Modeling the distributions of multiple et al. 2007; Clawges et al. 2008; Vogeler et al. 2013). There species at a regional or national scale has several are multiple metrics that might be used to model challenges because these modeling efforts must balance distribution of a specific species, but as mentioned ecologically relevant variables with the grain and extent above, two metrics that are broadly applicable in a GAP of available spatial data (e.g., Elith and Leathwick 2009). framework are LiDAR-derived shrub and snag data. These Additionally, the amount of spatial data (.90 gigabytes) two metrics are likely to improve GAP vertebrate models required to model species at regional and national because they represent real structural components of the extents challenges current computational power. Conse- landscape used by wildlife (Martinuzzi et al. 2009). Our quently, most models use fewer than 10 different metrics objectives were to assess 1) the performance of USGAP for each vertebrate species, regardless of their scale. For and NWGAP models with independently collected field example, national models use a maximum of seven data, and 2) whether the inclusion of LiDAR-derived variables (land cover, hydrology [e.g., stream velocity, shrub and snag data improved the performance of salinity, etc.], human avoidance, elevation, forest edge, USGAP and NWGAP models. We used data from field woodland–shrubland, and minimum patch size [http:// surveys of four shrub-nesting and four cavity-nesting gapanalysis.usgs.gov/]), similar to regional programs bird species to assess the accuracy of distribution models such as Southwest GAP (slope, aspect, distance to water, created by 1) the national USGAP data set only, 2) the land cover, soil type, percent rock outcrop, and landform; regional NWGAP data set only, 3) LiDAR-derived data Boykin et al. 2010). Some regional programs use even only, and 4) combinations of LiDAR, USGAP, and NWGAP fewer metrics; for example, NWGAP only used a single data. variable (land cover) in its species models (Aycrigg et al. 2013). Study Area Validation of GAP distribution models is important because they are used by many different stakeholders We conducted this study on Moscow Mountain in and for multiple purposes (Scott et al. 1993; Edwards et northern Idaho (approx. 46u449N, 116u589W). Moscow al. 1996; Peterson et al. 2001; Collinge et al. 2005; North Mountain encompasses about 20,000 ha of mixed co- American Bird Conservation Initiative, U.S. Committee niferous forest. Vegetation in our study varied by aspect, 2011; Boykin et al. 2013). However, research studies on elevation, and management history. Dominant tree GAP model accuracy have had differing findings, mostly species included ponderosa pine Pinus ponderosa, Doug- dependent on scale. Edwards et al. (1996) found that the las-fir Pseudotsuga menziesii, grand fir Abies grandis, accuracy rate (percent correctly classified) of GAP western red cedar Thuja plicata, and western larch Larix distribution models for vertebrates was .69% for eight occidentalis (Martinuzzi et al. 2009; Vogeler et al. 2013; national parks in Utah. In Idaho, Peterson et al. (2001) Washington State University 2014). Common shrub noted accuracy rates of up to 85%, but also
Details
-
File Typepdf
-
Upload Time-
-
Content LanguagesEnglish
-
Upload UserAnonymous/Not logged-in
-
File Pages11 Page
-
File Size-