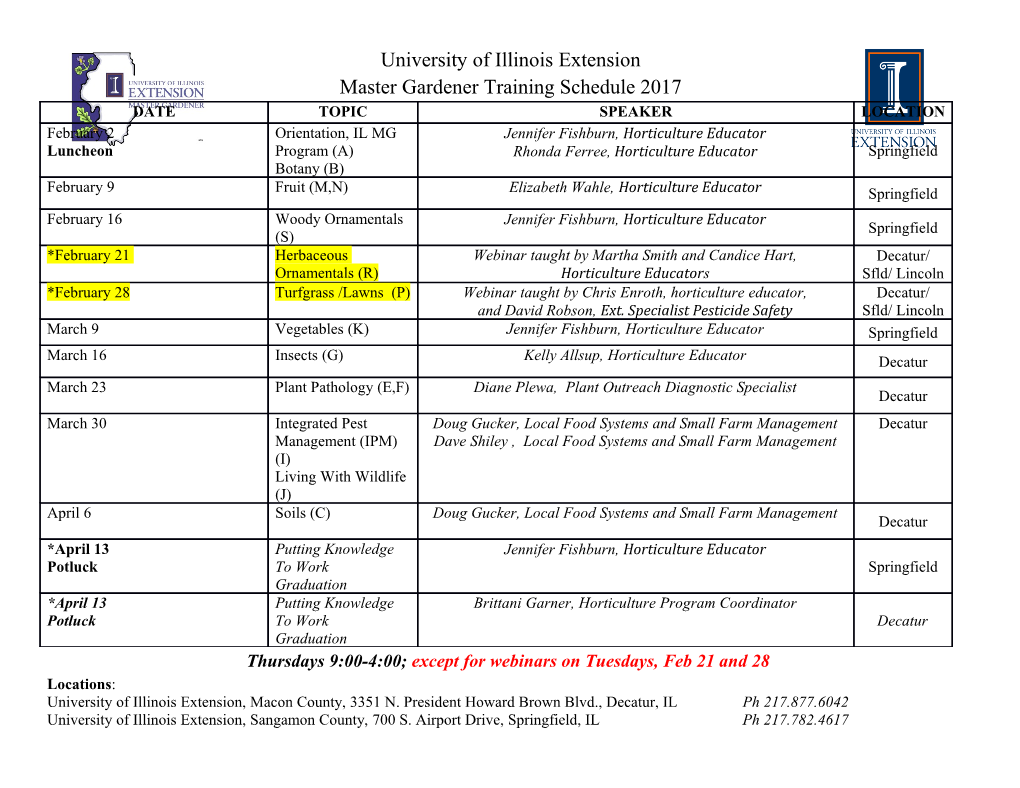
In statistics, a mixture model is a probabilistic model for representing the presence of sub-populations within an overall population, without requiring that an observed data-set should identify the sub-population to which an individual observation belongs. Formally a mixture model corresponds to the mixture distribution that represents the probability distribution of observations in the overall population. However, while problems associated with "mixture distributions" relate to deriving the properties of the overall population from those of the sub-populations, "mixture models" are used to make statistical inferences about the properties of the sub-populations given only observations on the pooled population, without sub-population-identity information. Some ways of implementing mixture models involve steps that do attribute postulated sub-population-identities to individual observations (or weights towards such sub-populations), in which case these can be regarded as a types of unsupervised learning or clustering procedures. However not all inference procedures involve such steps. Mixture models should not be confused with model for compositional data, i.e., data whose components are constrained to sum to a constant value (1, 100%, etc.). Structure of a mixture model General mixture model A typical finite-dimensional mixture model is a hierarchical model consisting of the following components: • N random variables corresponding to observations, each assumed to be distributed according to a mixture of K components, with each component belonging to the same parametric family of distributions but with different parameters • N corresponding random latent variables specifying the identity of the mixture component of each observation, each distributed according to a K-dimensional categorical distribution • A set of K mixture weights, each of which is a probability (a real number between 0 and 1), all of which sum to 1 • A set of K parameters, each specifying the parameter of the corresponding mixture component. In many cases, each "parameter" is actually a set of parameters. For example, observations distributed according to a mixture of one-dimensional Gaussian distributions will have a mean and variance for each component. Observations distributed according to a mixture of V-dimensional categorical distributions (e.g. when each observation is a word from a vocabulary of size V) will have a vector of V probabilities, collectively summing to 1. In addition, in a Bayesian setting, the mixture weights and parameters will themselves be random variables, and prior distributions will be placed over the variables. In such a case, the weights are typically viewed as a K-dimensional random vector drawn from a Dirichlet distribution (the conjugate prior of the categorical distribution), and the parameters will be distributed according to their respective conjugate priors. Mathematically, a basic parametric mixture model can be described as follows: Source URL: http://en.wikipedia.org/wiki/Mixture_model Saylor URL: http://www.saylor.org/courses/cs408 Attributed to [Wikipedia] Saylor.org Page 1 of 12 In a Bayesian setting, all parameters are associated with random variables, as follows: This characterization uses F and H to describe arbitrary distributions over observations and parameters, respectively. Typically H will be the conjugate prior of F. The two most common choices of F are Gaussian aka "normal" (for real-valued observations) and categorical (for discrete observations). Other common possibilities for the distribution of the mixture components are: • Binomial distribution, for the number of "positive occurrences" (e.g. successes, yes votes, etc.) given a fixed number of total occurrences • Multinomial distribution, similar to the binomial distribution, but for counts of multi-way occurrences (e.g. yes/no/maybe in a survey) • Negative binomial distribution, for binomial-type observations but where the quantity of interest is the number of failures before a given number of successes occurs • Poisson distribution, for the number of occurrences of an event in a given period of time, for an event that is characterized by a fixed rate of occurrence • Exponential distribution, for the time before the next event occurs, for an event that is characterized by a fixed rate of occurrence • Log-normal distribution, for positive real numbers that are assumed to grow exponentially, such as incomes or prices • Multivariate normal distribution (aka multivariate Gaussian distribution), for vectors of correlated outcomes that are individually Gaussian-distributed • A vector of Bernoulli-distributed values, corresponding e.g. to a black-and-white image, with each value representing a pixel; see the handwriting-recognition example below Specific examples A typical non-Bayesian Gaussian mixture model looks like this: A Bayesian version of a Gaussian mixture model is as follows: Source URL: http://en.wikipedia.org/wiki/Mixture_model Saylor URL: http://www.saylor.org/courses/cs408 Attributed to [Wikipedia] Saylor.org Page 2 of 12 A typical non-Bayesian mixture model with categorical observations looks like this: A typical Bayesian mixture model with categorical observations looks like this: Source URL: http://en.wikipedia.org/wiki/Mixture_model Saylor URL: http://www.saylor.org/courses/cs408 Attributed to [Wikipedia] Saylor.org Page 3 of 12 Examples A financial model Financial returns often behave differently in normal situations and during crisis times. A mixture model [1] for return data seems reasonable. Some model this as a jump-diffusion model, or as a mixture of two normal distributions. House prices Assume that we observe the prices of N different houses. Different types of houses in different neighborhoods will have vastly different prices, but the price of a particular type of house in a particular neighborhood (e.g. three-bedroom house in The normal distribution is plotted using different means and moderately-upscale neighborhood) will tend to cluster variances fairly closely around the mean. One possible model of such prices would be to assume that the prices are accurately described by a mixture model with K different components, each distributed as a normal distribution with unknown mean and variance, with each component specifying a particular combination of house type/neighborhood. Fitting this model to observed prices, e.g. using the expectation-maximization algorithm, would tend to cluster the prices according to house type/neighborhood and reveal the spread of prices in each type/neighborhood. (Note that for values such as prices or incomes that are guaranteed to be positive and which tend to grow exponentially, a log-normal distribution might actually be a better model than a normal distribution.) Topics in a document Assume that a document is composed of N different words from a total vocabulary of size V, where each word corresponds to one of K possible topics. The distribution of such words could be modeled as a mixture of K different V-dimensional categorical distributions. A model of this sort is commonly termed a topic model. Note that expectation maximization applied to such a model will typically fail to produce realistic results, due (among other things) to the excessive number of parameters. Some sorts of additional assumptions are typically necessary to get good results. Typically two sorts of additional components are added to the model: 1. A prior distribution is placed over the parameters describing the topic distributions, using a Dirichlet distribution with a concentration parameter that is set significantly below 1, so as to encourage sparse distributions (where only a small number of words have significantly non-zero probabilities). 2. Some sort of additional constraint is placed over the topic identities of words, to take advantage of natural clustering. • For example, a Markov chain could be placed on the topic identities (i.e. the latent variables specifying the mixture component of each observation), corresponding to the fact that nearby words belong to similar topics. (This results in a hidden Markov model, specifically one where a prior distribution is placed over state transitions that favors transitions that stay in the same state.) • Another possibility is the latent Dirichlet allocation model, which divides up the words into D different documents and assumes that in each document only a small number of topics occur with any frequency. Source URL: http://en.wikipedia.org/wiki/Mixture_model Saylor URL: http://www.saylor.org/courses/cs408 Attributed to [Wikipedia] Saylor.org Page 4 of 12 Handwriting recognition The following example is based on an example in Christopher M. Bishop, Pattern Recognition and Machine Learning. Imagine that we are given an NxN black-and-white image that is known to be a scan of a hand-written digit between 0 and 9, but we don't know which digit is written. We can create a mixture model with different components, where each component is a vector of size of Bernoulli distributions (one per pixel). Such a model can be trained with the expectation-maximization algorithm on an unlabeled set of hand-written digits, and will effectively cluster the images according to the digit being written. The same model could then be used to recognize the digit of another image simply by holding the parameters constant, computing the probability of the new image for each possible digit (a trivial calculation), and returning the digit that generated the highest probability. Direct and indirect
Details
-
File Typepdf
-
Upload Time-
-
Content LanguagesEnglish
-
Upload UserAnonymous/Not logged-in
-
File Pages12 Page
-
File Size-