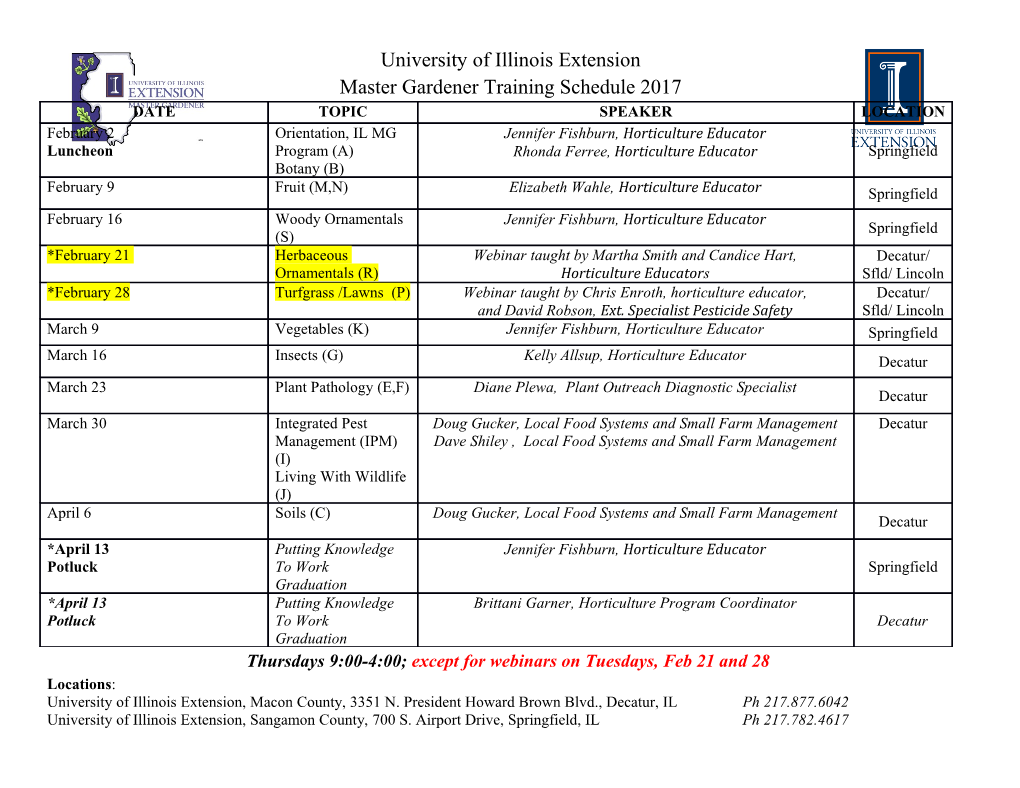
BUILDING AND UNDERSTANDING: REPRESSILATORS AND SYNCHRONY THREE CELLS IN A BUBBLE BATH Brenton Munson Clara Posner Jonathan Pekar October 23, 2016 UNIVERSITY OF CALIFORNIA SAN DIEGO DEPARTMENT OF BIOENGINNERING BENG 221 PROJECT i Contents ii CONTENTS 1 Introduction 1 1.1 Problem Statement . .2 2 Methods 2 2.1 Simplifying and Parameterization . .2 2.2 Numerical Method . .5 3 Results and Discussion6 References 14 4 Appendix 16 4.1 Code............................................. 16 4.1.1 Single Cell Repressilator Model . 16 4.1.2 Repressilator Model with Drug Sensing . 23 LISTOFFIGURES Figure 1 Repressilator System . .2 Figure 2 Repressilator with Drug Sensing . .4 Figure 3 Multicell Environment . .5 Figure 4 Starting a Repressilator . .7 Figure 5 Repressilator at Steady State . .8 Figure 6 Model Sensitivity to Transcription Rate . .8 Figure 7 Model Sensitivity to Translation Rate . .9 Figure 8 Model Sensitivity to Protein Degradation Rates . 10 Figure 9 Model Sensitivity to Hill Parameters . 11 Figure 10 Controlling a Repressilator with an External Stimulus . 11 Figure 11 Cell Synchronization . 12 Figure 12 Cell Synchronization . 12 INTRODUCTION 1 1 INTRODUCTION The demands placed upon modern medicine are rapidly driving scientists and engineers to inves- tigate the mechanisms of disease and biological function to ever-increasing complexity. Much of this frontier lies in modeling not only the outcome of individual genetic perturbations but also how the networks of genetic interactions coordinate cellular- and tissue-level phenotypes [1]. While progress has been made in describing living systems, these networks often become too complex and cumbersome to interrogate with the current tools of bioinformatics [2,3]. As such, the problem is often broken down into more discrete subunits, with which they can be modeled, perturbed, and hopefully understood on a more functional level [4]. In a classical engineering sense, these broken down sub-networks can be thought of as unit operations, and, once characterized, can be pieced together into higher order biological systems. Synthetic biology is an emerging field at the intersection of engineering and molecular and cellular biology, where it attempts to create and model both novel and natural systems. There are two approaches within synthetic biology to tackle such problems: top-down and bottom-up [5]. The former attempts to take a living system, such as yeast, and insert designed biological units, often in the form of DNA plasmids. By leveraging the existing cellular infrastructure of a host organism, scientists can rapidly develop novel systems without needing to create an entire de novo system. However, this hijacking is not without consequences. While the systems of synthetic biology are currently relatively small, they can still impart stress on the host organism and change functions in unforeseen ways. Therefore, synthetic biology constructs (as well as all biological constructs) must be understood in the context of signaling and genetic regulatory networks in order to better understand their roles and consequences. The bottom-up approach to synthetic biology can avoid much of the complications arising from using a host organism by taking non-living components and initializing the system ex-vivo [6]. How- ever, designing and constructing such systems is largely outside our current technological purview. While a bottom-up approach will lend itself well to a more controlled environment for understanding synthetic constructs, current reports and models mostly leverage a top-down approach. However, when modeling regulatory networks in living systems, it is still useful to start from the fundamen- tals. The central dogma of molecular biology describes how DNA is encoded variably into RNA, which is then translated into protein. The produced proteins are the machines of the cell, providing a vast array of functions and forms. Controlling this process is one of the fundamental roles of cellular function and is carried out by protein-based transcription factors. The first synthetic gene circuits implemented in biological hosts were the toggle switch and the repressilator. They have been used to study the dynamic behavior of transcription and translation, as well as to begin to develop theories and predictive capacities regarding synthetic networks [7,8]. The toggle switch consists of a bi-repressive system of two genes, where the protein product of each gene acts as a repressor of the other gene, while also acting as a self-promoter. The METHODS 2 Figure 1: Repressilator System system exhibits a switch-like behavior: one gene is constitutively expressed, while the other re- mains untranscribed. Bifurcation between bistable and monostable systems arises from varying repressor strengths and cooperativity. The repressilator takes the toggle switch one step further by introducing an additional repressing protein to the system, giving rise to oscillatory behavior. Here we investigate a mathematical model of the repressilator to better understand the dynamics and sensitivities of the system, model parameters, and propose a control mechanism by which oscillatory behavior can be temporally controlled. 1.1 Problem Statement Genetic maps are often constructed without a mathematical framework that would help predict function and consequences when changes occur. Here, we look at the relationship among three genes that repress each other and model their relationship mathematically. We use numerical methods to elucidate the dynamic relationships arising from their map structure. We then use these methods to look at how a drug might induce synchrony among cells. 2 METHODS 2.1 Simplifying and Parameterization We describe the repressilator system by a series of ordinary, nonlinear differential equations (equa- tions1 to3). The main processes that change the concentration of the mRNA for each gene are degradation and repressible transcription. We assume the degradation is the product of the an mRNA degradation rate constant (kdm) and the instantaneous mRNA concentration. The pro- duction of mRNA is the transcription rate α (currently assumed to be equal for all three genes), modulated by the concentration of the repressor. The Hill coefficient (n) accounts for the coopera- tivity of a protein in its repression of mRNA transcription. METHODS 3 da α = -k a + (1) dt dm 1 + Cn db α = -k b + (2) dt dm 1 + An dc α = -k c + (3) dt dm 1 + Bn The protein is created by translation, quantified by the product of the translation rate β and the mRNA concentration. We also assume the degradation is the product of the protein degradation rate constant (kdp) and the instantaneous protein concentration. dA = βA - k A (4) dt dp dB = βB - k B (5) dt dp dC = βC - k C (6) dt dp For our single cell simulation, we aim to create a repressilator system that will reach an oscilla- tory steady state involving its three genetic elements. To achieve this, we set the initial concentra- tions of all mRNA and protein to zero, except for the mRNA from gene A, which we set to 1M. We set the following parameter values: transcription rate (α) to 100, the Hill coefficient (n) to 2, the degradation constants of both mRNA and protein (kdm and kdp) to 1, and the translation rate (β) to 1. To examine the effect of the different parameters on the system and its oscillatory nature, we vary the transcription rate (α), translation rate (β), and the Hill coefficient (n). We can control the oscillatory nature of the repressilator system in one cell by introducing a drug D that induces the transcription of gene A at rate k. For this model, we assume the drug diffuses into the cell and the diffusion out of the cell is negligible. We assume that the cell is permeable to the drug inducer, so that an injection of concentrated drug into the extracellular media causes an equal concentration spike of drug concentration inside the cell. The drug addition only modifies the rate of change of mRNA concentration for gene A; the previous mRNA and protein equations remain the same (Fig.2). The drug is also assumed to degrade at a rate of kdd, so that it is not continually activating gene A. dA α = -k a + + kD (7) dt dm 1 + Cn dD = -k D (8) dt dd METHODS 4 Figure 2: Diagram of the Repressilator System in a Single Cell with a Drug Diffusing into the Cell and Inducing Gene A Our next modification to the system is to model three different cells all containing the repressila- tor system, but each cell is initialized with a non-zero mRNA concentration of a different gene (Fig. 3). We model a large spike of the drug inducer to the extracellular media surrounding all three cells by assuming that each cell uptakes the same amount of drug. This is to examine whether we can use an external agent to synchronize a group of asynchronous cells. The differential equations used to model each cell in the three-cell system (shown in equations 9 to 15) are the same as the equations for the single cell with the added drug. The “i” denotes the identity of the cell as labeled by a number. dAi α = -kdmai + n + kD (9) dt 1 + Ci dbi α = -kdmbi + n (10) dt 1 + Ai dci α = -kdmci + n (11) dt 1 + Bi dA i = βA - k A (12) dt i dp i dB i = βB - k B (13) dt i dp i dC i = βC - k C (14) dt i dp i dD = -k D (15) dt dd METHODS 5 Figure 3: Diagram of the Three Cells containing the Repressilator with a Drug Diffusing into the Cell and Inducing Gene A. Initially, all protein levels are set to zero. Cell 1 starts with a 1M mRNA for gene A, cell 2 starts with a 1M mRNA for gene B, and cell 3 starts with a 1M mRNA for gene C. The cells are allowed to reach steady-state oscillations before and after the drug is spiked in.
Details
-
File Typepdf
-
Upload Time-
-
Content LanguagesEnglish
-
Upload UserAnonymous/Not logged-in
-
File Pages28 Page
-
File Size-