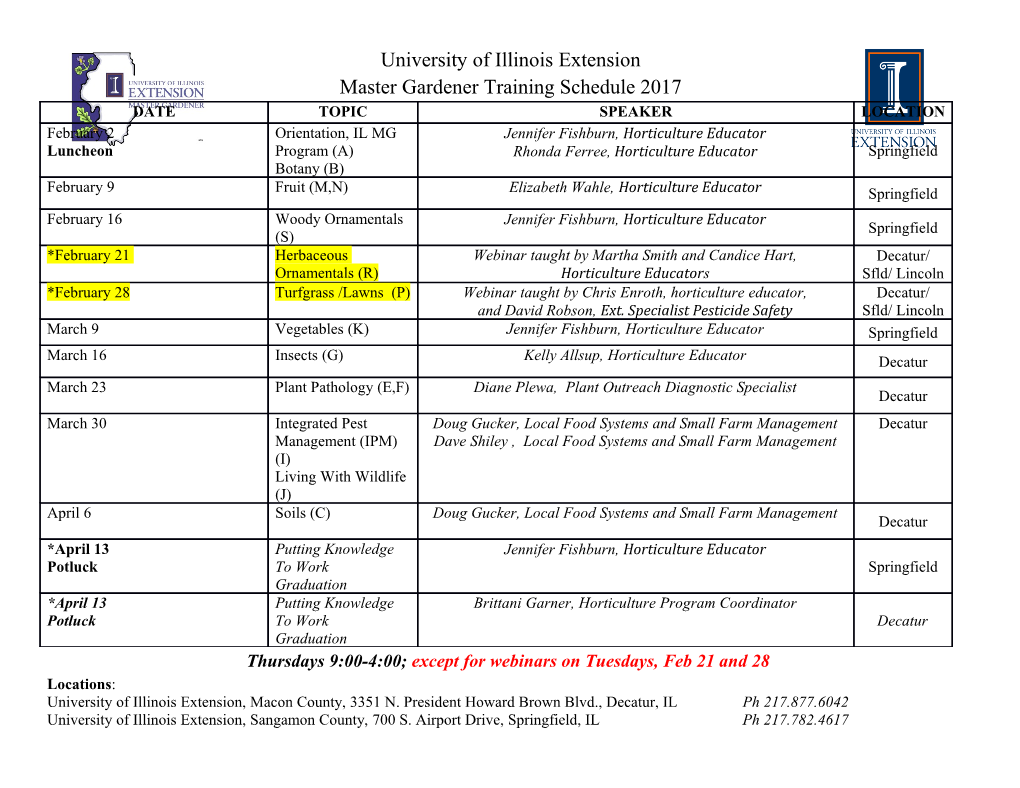
Google Cloud Dataflow A Unified Model for Batch and Streaming Data Processing Jelena Pjesivac-Grbovic STREAM 2015 Agenda 1 Data Shapes 2 Data Processing Tradeoffs 3 Google’s Data Processing Story 4 Google Cloud Dataflow Score points Form teams Geographically distributed Online and Offline mode User and Team statistics in real time Accounting / Reporting Abuse detection https://commons.wikimedia.org/wiki/File:Globe_centered_in_the_Atlantic_Ocean_(green_and_grey_globe_scheme).svg 1 Data Shapes Data... ...can be big... ...really, really big... Thursday Wednesday Tuesday ...maybe even infinitely big... 8:00 1:00 9:00 2:00 10:00 3:00 11:00 4:00 12:00 5:00 13:00 6:00 14:00 7:00 … with unknown delays. 8:00 8:00 8:00 8:00 9:00 10:00 11:00 12:00 13:00 14:00 2 Data Processing Tradeoffs Data Processing Tradeoffs 1 + 1 = 2 $$$ Completeness Latency Cost Requirements: Billing Pipeline Important Not Important Completeness Low Latency Low Cost Requirements: Live Cost Estimate Pipeline Important Not Important Completeness Low Latency Low Cost Requirements: Abuse Detection Pipeline Important Not Important Completeness Low Latency Low Cost Requirements: Abuse Detection Backfill Pipeline Important Not Important Completeness Low Latency Low Cost Google’s 3 Data Processing Story Data Processing @ Google Dataflow MapReduce FlumeJava Dremel Spanner GFS Big Table Pregel Colossus MillWheel 2002 2004 2006 2008 2010 2012 2014 2016 Data Processing @ Google Dataflow MapReduce FlumeJava Dremel Spanner GFS Big Table Pregel Colossus MillWheel 2002 2004 2006 2008 2010 2012 2014 2016 Data Processing @ Google Dataflow MapReduce FlumeJava Dremel Spanner GFS Big Table Pregel Colossus MillWheel 2002 2004 2006 2008 2010 2012 2014 2016 Batch Patterns: Transform, Filter, and Aggregate [11:00 - MR Tuesday 12:00)[12:00 - 13:00)[13:00 - 14:00)[14:00 - 15:00)[15:00 - 16:00)[16:00 - 17:00)[18:00 - 19:00)[19:00 - 20:00)[21:00 - 22:00)[22:00 - MR 23:00)[23:00 - 0: Create structured data 00) Thursday Wednesday Tuesday Generate time-based windows MR Create structured data, repeatedly Batch Patterns: Sessions Tuesday Wednesday Tuesday Wednesday Jose Lisa Ingo MapReduce Asha Cheryl Ari Data Processing @ Google Dataflow MapReduce FlumeJava Dremel Spanner GFS Big Table Pregel Colossus MillWheel 2002 2004 2006 2008 2010 2012 2014 2016 Streaming Patterns: Element-wise transformations Processing 8:00 9:00 10:00 11:00 12:00 13:00 14:00 Time Streaming Patterns: Aggregating Time Based Windows Processing 8:00 9:00 10:00 11:00 12:00 13:00 14:00 Time Streaming Patterns: Event Time Based Windows Input Processing Time 10:00 11:00 12:00 13:00 14:00 15:00 Output Event Time 10:00 11:00 12:00 13:00 14:00 15:00 Streaming Patterns: Session Windows Input Processing Time 10:00 11:00 12:00 13:00 14:00 15:00 Output Event Time 10:00 11:00 12:00 13:00 14:00 15:00 Event-Time Skew Watermarks describe event time Watermark progress. Skew "No timestamp earlier than the watermark will be seen" Often heuristic-based. Processing Time Too Slow? Results are delayed. Too Fast? Some data is late. Event Time Streaming or Batch? 1 + 1 = 2 $$$ Completeness Latency Cost Why not both? Data Processing @ Google Dataflow MapReduce FlumeJava Dremel Spanner GFS Big Table Pregel Colossus MillWheel 2002 2004 2006 2008 2010 2012 2014 2016 4 Google Cloud Dataflow What are you computing? How does the event time affect computation? How does the processing time affect latency? How do results get refined? What are you computing? • A Pipeline represents a graph of data processing transformations • PCollections flow through the pipeline • Optimized and executed as a unit for efficiency Dataflow Model: Data • A PCollection<T> is a collection of data of type T • Maybe be bounded or unbounded in size • Each element has an implicit timestamp • Initially created from backing data stores Dataflow Model: Transformations PTransforms transform PCollections into other PCollections. Element-Wise Aggregating Composite What Where When How Example: Computing Integer Sums // Collection of raw log lines PCollection<String> raw = ...; // Element-wise transformation into team/score pairs PCollection<KV<String, Integer>> input = raw.apply(ParDo.of(new ParseFn())) // Composite transformation containing an aggregation PCollection<KV<String, Integer>> output = input .apply(Sum.integersPerKey()); What Where When How Example: Computing Integer Sums What Where When How Example: Computing Integer Sums What Where When How Aggregating According to Event Time • Windowing divides data into event- time-based finite chunks. Key Key Key Key Key Key Key Key Key 1 2 3 1 2 3 1 2 3 • Required when 1 1 1 doing aggregations 2 2 2 3 3 4 3 over unbounded 4 5 4 Fixed Sliding Sessions data. What Where When How Example: Fixed 2-minute Windows PCollection<KV<String, Integer>> output = input .apply(Window.into(FixedWindows.of(Minutes(2)))) .apply(Sum.integersPerKey()); What Where When How Example: Fixed 2-minute Windows What Where When How When in Processing Time? • Triggers control Watermark when results are emitted. • Triggers are often relative to the Processing Time watermark. Event Time What Where When How Example: Triggering at the Watermark PCollection<KV<String, Integer>> output = input .apply(Window.into(FixedWindows.of(Minutes(2))) .trigger(AtWatermark()) .apply(Sum.integersPerKey()); What Where When How Example: Triggering at the Watermark What Where When How Example: Triggering for Speculative & Late Data PCollection<KV<String, Integer>> output = input .apply(Window.into(FixedWindows.of(Minutes(2))) .trigger(AtWatermark() .withEarlyFirings(AtPeriod(Minutes(1))) .withLateFirings(AtCount(1)))) .apply(Sum.integersPerKey()); What Where When How Example: Triggering for Speculative & Late Data What Where When How How are Results refined? • How should multiple outputs per window accumulate? • Appropriate choice depends on consumer. Firing Elements Discarding Accumulating Acc. & Retracting Speculative 3 3 3 3 Watermark 5, 1 6 9 9, -3 Late 2 2 11 11, -9 Total Observ 11 11 23 11 What Where When How Example: Add Newest, Remove Previous PCollection<KV<String, Integer>> output = input .apply(Window.into(Sessions.withGapDuration(Minutes(1))) .trigger(AtWatermark() .withEarlyFirings(AtPeriod(Minutes(1))) .withLateFirings(AtCount(1))) .accumulatingAndRetracting()) .apply(new Sum()); What Where When How Example: Add Newest, Remove Previous What Where When How Customizing What Where When How 1. Classic Batch 2. Batch with Fixed Windows 3. Streaming 4. Streaming with 5. Streaming with Speculative + Late Data Retractions What Where When How Google Cloud Dataflow A fully-managed cloud service and Cloud Dataflow programming model for batch and streaming big data processing. Open Source SDKs ● Used to construct a Dataflow pipeline. ○ Custom IO: both bounded and unbounded ● Available in Java. Python in the works. ● Pipelines can run… ○ On your development machine ○ On the Dataflow Service on Google Cloud Platform ○ On third party environments like Spark or Flink. Fully Managed Dataflow Service Runs the pipeline on Google Cloud Platform. Includes: ● Graph optimization: Modular code, efficient execution ● Smart Workers: ○ Full Lifecycle management, ○ Autoscaling (up and down) ○ Dynamic task rebalancing ● Easy Monitoring: Dataflow UI, Restful API and CLI, Integration with Cloud Logging, etc. Learn More! ● The Dataflow Model @VLDB 2015 http://www.vldb.org/pvldb/vol8/p1792-Akidau.pdf ● Dataflow SDK for Java https://github.com/GoogleCloudPlatform/DataflowJavaSDK ● Google Cloud Dataflow on Google Cloud Platform http://cloud.google.com/dataflow (Free Trial!) Thank you .
Details
-
File Typepdf
-
Upload Time-
-
Content LanguagesEnglish
-
Upload UserAnonymous/Not logged-in
-
File Pages55 Page
-
File Size-