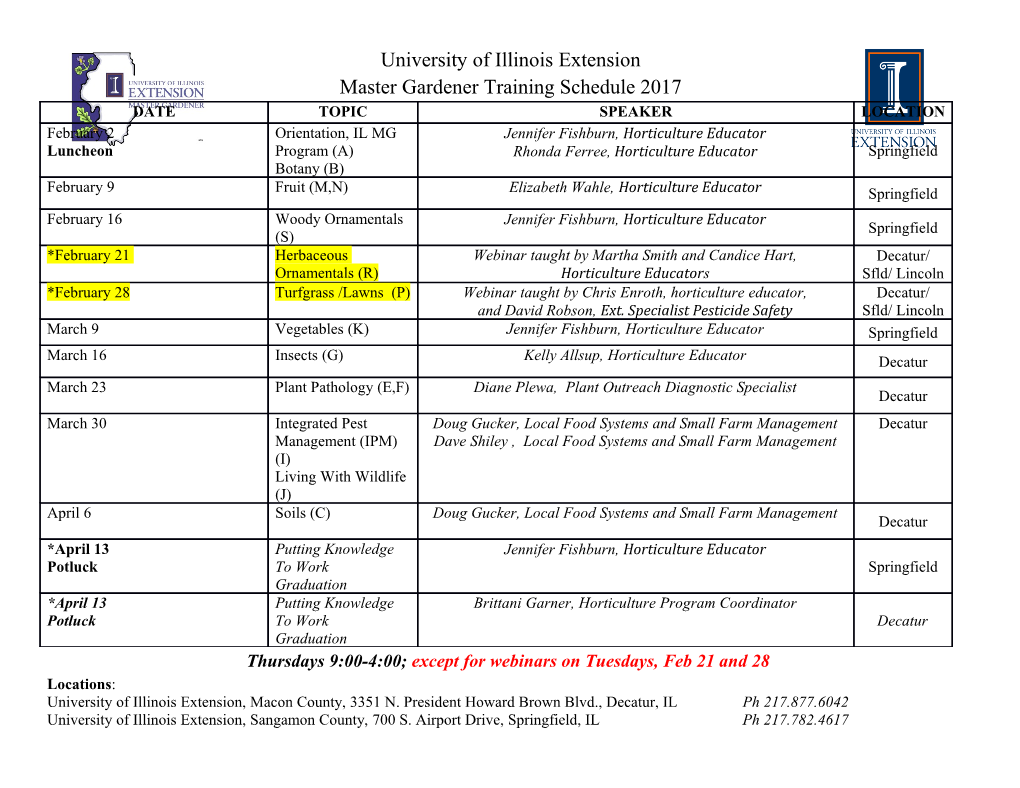
Induction • Inductive arguments (or simply induction) – Reasoning from particular cases to general conclusions. – In general, supported by statistical analysis or probability. • Causal inductive arguments – Special type of induction in which the premises suggest the conclusion that there is a causal relation between two properties or factors. Characteristics of Inductive Arguments 1. Premises and conclusions are all empirical propositions – Descriptions of matters of fact – Not definitions – Not value judgements. 2. Conclusion is not deductively entailed by the premises 3. Underlying reasoning is that the regularities described in the premises will persist throughout the range described in the conclusion 4. Conclusion is either: (a) that unexamined case will resemble the examined ones (b) the premises support an explanatory causal hypothesis Populations, Sample Size and Bias • Inductive conclusions generalize. We sometimes say that they extrapolate. This just means that inductive conclusions give more information than the information stated in the premises. – For example, “3,356 crows were observed to be black, so all crows are black.” • The conclusion makes a statement about some population or group based on a sample that is smaller that is smaller than the population or group. • For an inductive conclusion to be reasonable, the premises must be a representative sample of the whole population. – An unrepresentative sample is called a biased sample. – Biased sampling is a serious problem, and it often arises unintentionally because of a lack of understanding of the population being sampled. Representative Samples • Evaluating whether or not a sample is representative is difficult and is not straightforward. • It involves a variety of factors, including population size, population homogeneity, and the particular property being sampled. • To understand this more completely TAKE A STATISTICS COURSE before leaving university!!! • That said, three things to keep in mind: 1) Sampling works best when it is a random sample of the total population. Biased sampling leads to misrepresentative inductive generalization. 2) For a population in the millions, a random sample of about 1,000 can provide a perfectly representative picture of a populations. 3) In order to achieve a representative sample, small populations require the sample to be a greater proportion of the population than for larger populations. (Small populations tend to exhibit more variability.) Evaluating Inductive Arguments • By definition, the representativeness of a sample can never be proven in an inductive argument. – Representativeness needs to be evaluated using good sense and good judgment. – In statistics, confidence is the metric used to evaluate this question. – If you don’t know any statistics, then you need to make a good guess about whether the sample is representative of the population being sampled. • Key question: How likely is it that the target population resembles the sample population in the relevant respects? – Same question expressed succinctly: Is the sample population representative? Evaluating Inductive Generalizations Four steps: 1) Identify the sample and target populations as precisely as possible (relying on contextual cues if necessary). 2) Identify the size of the sample. 3) Reflect on the degree of variability of the trait or property which is extrapolated in the conclusion. 4) Reflect on the sampling process. • Have techniques been used to minimize the possibility of bias? • e.g., increasing sample size, stratified sampling, and so forth. Two General Problems with Inductive Arguments • Pseudoprecision – The attempt to make a conclusion seem to be justified by expressing premises with numerical exactness. – “Ignatieff is now 27.6 percentage points ahead of Harper.” • Questionable definition – “Studies prove that over 95% of communication is non-verbal, body language”. Four Inductive Fallacies 1) Hasty inductive generalization: a sample is grossly inadequate so that it is guaranteed to be unrepresentative, and hence, unacceptable. 2) Anecdotal arguments: a common, special case of a hasty inductive generalization, which involves basing an inductive generalization entirely on one (gripping or compelling) story. Again, unrepresentative, and hence, unacceptable. 3) Genetic fallacy: premises offer information about the origins of something, and a conclusion is inferred from this about the thing’s fundamental properties or value. A fallacy of relevance. 4) Fallacies of composition and division: Attributing to the wholes the properties of parts. A fallacy of relevance. Genetic Fallacy • “86% of people in the world believe that God exists, so God must exist.” – This example involves incorrect reasoning from a (presumably) correct fact about the number of people who believe in god to the existence of god. The number of people who believe in god tells us nothing about the putative properties of god. • “America will always be a maverick state. The majority of the Founding Fathers were simply rabble rousers.” • But becareful: In many cases, the origin of a claim is relevant to the evaluation of the acceptability of the claim. – Consider assessment of testimony of texts or experts. Fallacy of Composition and Division • Two sides of the same coin: – The fallacy of composition is committed by arguing that because something is true of members of a group, it is true of a group as a whole. – The fallacy of division is committed by arguing that if something is true of a group, then it is also true of the individuals belonging to the group. Example: Fallacy of Composition • Classic illustration: – “Atoms are not visible to the naked eye, and humans are made up of atoms, so humans are not visible to the naked eye.” • “A US battleship consumes more fuel than a 9.9hp motor boat, so all the battleships consume more fuel than 9.9hp motor boats.” Example: Fallacy of Division • Classic illustration: – “Humans are visible, and humans are made up of atoms, so atoms are visible.” • “Canadians use more electricity per capita than Americans. So, Joe Bloggs in St. John’s uses more power than American, Lee Pippa.” Causal Inductive Arguments • Causal inductive argument: – An inductive argument in which the conclusion is that there is a causal relation between two factors or properties. – E.g., Smoking causes cancer. Green-house-gas emissions from industry cause global climate change. • Causal inductive arguments are ubiquitous: – Arguments in science commonly suppose the identification of causes. • But, this kind of argument leads to a number of distinctive complications and problems. In particular, it is difficult to distinguish between: 1. Constant conjunction, i.e., correlation of factors 2. Necessary connection, i.e., a causal connection between factors Causes • We say things in the form of “C caused E” and “E was caused by C”. – As noted in the discussion of the logical conditional, when we say “If C then E” we tend to suppose (incorrectly) that we are talking about a causal relationship. • That said, the nature of causation, and related problem of verifying causal claims, are vexing questions to philosophers. • Saying that “C caused E” could be explained in, at least, one of three things: 1) Event: C caused E means at least that C happened and then E happened, but in addition that something about C engendered or brought into being E. 2) Counterfactual conditional: C caused E, if and only if, C had not occurred, then E would not have occurred. 3) Law of nature: C caused E, if and only if, it is a law of nature that Cs always cause Es. Five Different Interpretations of ‘C causes E’ 1. C is a necessary condition for E: without C, E would not occur. (The presence of oxygen is a necessary condition for human life.) 2. C is a sufficient condition for E: C is enough by itself to produce E. (Massive heart failure is a sufficient condition for death.) 3. C is a necessary and sufficient condition for E. (Having diabetes is necessary and sufficient for having a malfunctioning pancreas.) 4. C is an especially important causal factor, leading to E: Individually necessary part of a jointly sufficient causal chain. (The carelessly discarded cigarette butt caused the fire.) 5. C is a causal factor, contributing to the likelihood of E. (Smoking causes lung cancer.) Correlation and Cause • The premises of a causal induction typically establish, among a sample group, a correlation between two properties or factors. • The conclusion of a causal induction extrapolates beyond this data in two ways. 1) Like all inductive arguments, it extrapolates that the regularity observed between factors the sample will persist in the target population. 2) It adds the further conjecture that the regularity will persist because there is a causal connection or relation between the factors. • Why does (2) present a special problem? Example: Allergies and Asthma • In recent decades, certain kinds of allergies (e.g., peanut) and asthma have been drastically on the rise in Western nations, though not elsewhere in the world. • The evidence that they are significantly correlated is overwhelming. – If you have these kinds of allergies, then there is a statistically significant increase in the chance that you are prone to develop asthma. – Likewise, if you have asthma, then there is a statistically significant increase in the chance that you are prone to develop these kinds of allergies. • But, is there a causal relation between allergies and asthma? No amount of correlation will distinguish among these three
Details
-
File Typepdf
-
Upload Time-
-
Content LanguagesEnglish
-
Upload UserAnonymous/Not logged-in
-
File Pages31 Page
-
File Size-