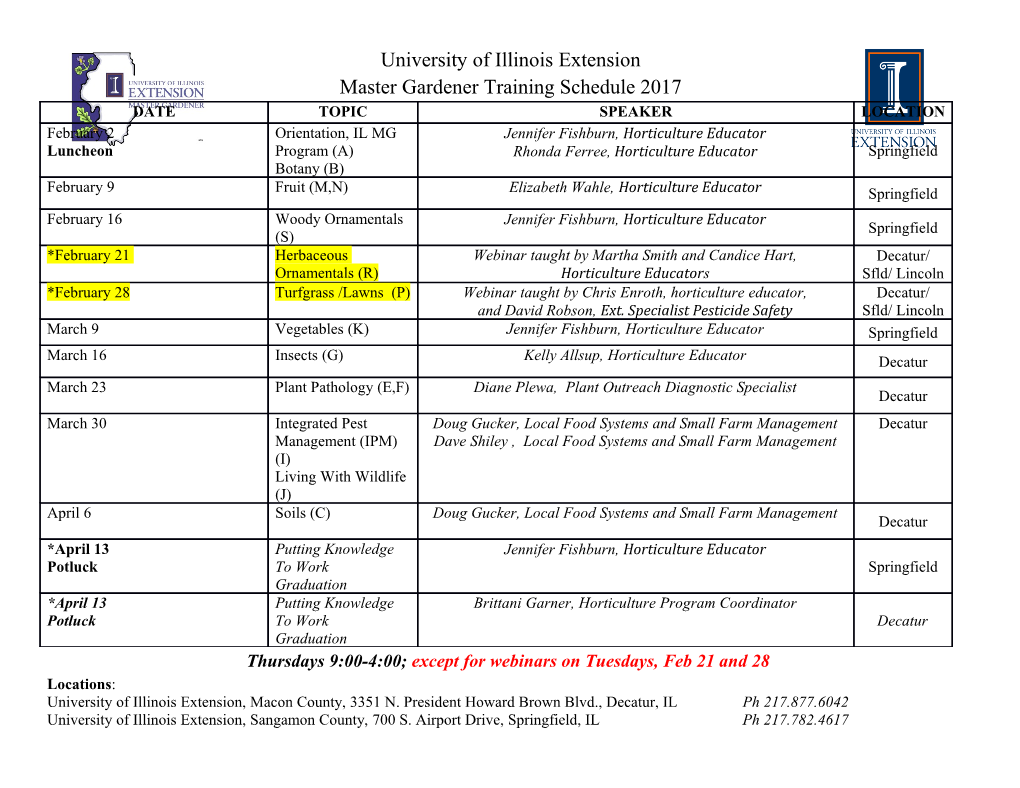
Productive High Performance Parallel Programming with Auto-tuned Domain-Specific Embedded Languages By Shoaib Ashraf Kamil A dissertation submitted in partial satisfaction of the requirements for the degree of Doctor of Philosophy in Computer Science in the Graduate Division of the University of California, Berkeley Committee in charge: Professor Armando Fox, Co-Chair Professor Katherine Yelick, Co-Chair Professor James Demmel Professor Berend Smit Fall 2012 Productive High Performance Parallel Programming with Auto-tuned Domain-Specific Embedded Languages Copyright c 2012 Shoaib Kamil. Abstract Productive High Performance Parallel Programming with Auto-tuned Domain-Specific Embedded Languages by Shoaib Ashraf Kamil Doctor of Philosophy in Computer Science University of California, Berkeley Professor Armando Fox, Co-Chair Professor Katherine Yelick, Co-Chair As the complexity of machines and architectures has increased, performance tuning has become more challenging, leading to the failure of general compilers to generate the best possible optimized code. Expert performance programmers can often hand-write code that outperforms compiler- optimized low-level code by an order of magnitude. At the same time, the complexity of programs has also increased, with modern programs built on a variety of abstraction layers to manage complexity, yet these layers hinder efforts at optimization. In fact, it is common to lose one or two additional orders of magnitude in performance when going from a low-level language such as Fortran or C to a high-level language like Python, Ruby, or Matlab. General purpose compilers are limited by the inability of program analysis to determine pro- grammer intent, as well as the lack of detailed performance models that always determine the best executable code for a given computation and architecture. The latter problem can be mitigated through auto-tuning, which generates many code variants for a particular problem and empirically determines which performs best on a given architecture. This thesis addresses the problem of how to write programs at a high level while obtaining the performance of code written by performance experts at the low level. To do so, we build domain- specific embedded languages that generate low-level parallel code from a high-level language, and then use auto-tuning to determine the best performing low-level code. Such DSELs avoid analysis by restricting the domain while ensuring programmers specify high-level intent, and by performing empirical auto-tuning instead of modeling machine parameters. As a result, programmers write in high-level languages with portions of their code using DSELs, yet obtain performance equivalent to the best hand-optimized low-level code, across many architectures. We present a methodology for building such auto-tuned DSELs, as well as a software in- frastructure and example DSELs using the infrastructure, including a DSEL for structured grid computations and two DSELs for graph algorithms. The structured grid DSEL obtains over 80% of peak performance for a variety of benchmark kernels across different architectures, while the graph algorithm DSELs mitigate all performance loss due to using a high-level language. Overall, the methodology, infrastructure, and example DSELs point to a promising new direction for obtaining high performance while programming in a high-level language. 1 For all who made this possible. i Contents List of Figures vii List of Tables x List of Symbols xi Acknowledgements xiii 1 Introduction 1 1.1 Thesis Contributions . .2 1.2 Thesis Outline . .3 2 Motivation and Background 6 2.1 Trends in Computing Hardware . .6 2.2 Trends in Software . .7 2.3 The Productivity-Performance Gap . .8 2.4 Auto-tuning and Auto-tuning Compilers . .9 2.5 Summary . 10 3 Related Work 11 3.1 Optimized Low-level Libraries and Auto-tuning . 11 3.2 Accelerating Python . 12 3.3 Domain-Specific Embedded Languages . 12 3.4 Just-in-Time Compilation & Specialization . 13 3.5 Accelerating Structured Grid Computations . 14 3.6 Accelerating Graph Algorithms . 14 3.7 Summary . 15 4 SEJITS: A Methodology for High Performance Domain-Specific Embedded Languages 16 4.1 Overview of SEJITS . 16 4.2 DSELs and APIs in Productivity Languages . 18 4.3 Code Generation . 21 4.4 Auto-tuning . 22 4.5 Best Practices for DSELs in SEJITS . 22 4.6 Language Requirements to Enable SEJITS . 23 4.7 Summary . 24 ii 5 Asp is SEJITS for Python 25 5.1 Overview of Asp . 25 5.2 Walkthrough: Building a DSEL Compiler Using Asp . 26 5.2.1 Defining the Semantic Model . 26 5.2.2 Transforming Python to Semantic Model Instances . 29 5.2.3 Generating Backend Code . 30 5.3 Expressing Semantic Models . 31 5.4 Code Generation . 32 5.4.1 Dealing with Types . 33 5.5 Just-In-Time Compilation of Asp Modules . 34 5.6 Debugging Support . 34 5.7 Auto-tuning Support . 35 5.8 Summary . 36 6 Experimental Setup 37 6.1 Hardware Platforms . 37 6.2 Software Environment . 38 6.2.1 Compilers & Runtimes . 38 6.2.2 Parallel Programming Models . 39 6.3 Performance Measurement Methodology . 39 6.3.1 Timing Methodology . 39 6.3.2 Roofline Model . 39 6.4 Summary . 40 7 Overview of Case Studies 42 8 Structured Grid Computations 44 8.1 Characteristics of Structured Grid Computations . 45 8.1.1 Applications . 45 8.1.2 Dimensionality . 45 8.1.3 Connectivity . 45 8.1.4 Topology . 47 8.2 Computational Characteristics . 48 8.2.1 Data Structures . 48 8.2.2 Interior Computation & Boundary Conditions . 49 8.2.3 Memory Traffic . 50 8.3 Optimizations . 50 8.3.1 Algorithmic Optimizations . 51 8.3.2 Cache and TLB Blocking . 52 8.3.3 Vectorization . 53 8.3.4 Locality Across Grid Sweeps . 54 8.3.5 Communication Avoiding Algorithms . 55 8.3.6 Parallelization . 55 8.3.7 Summary of Optimizations . 55 8.4 Modeling Performance of Structured Grid Algorithms . 56 iii 8.4.1 Serial Performance Models . 56 8.4.2 Roofline Model for Structured Grid . 57 8.5 Summary . 59 9 An Auto-tuner for Parallel Multicore Structured Grid Computations 61 9.1 Structured Grids Kernels & Architectures . 61 9.1.1 Benchmark Kernels . 64 9.1.2 Experimental Platforms . 65 9.2 Auto-tuning Framework . 65 9.2.1 Front-End Parsing . 65 9.2.2 Structured Grid Kernel Breadth . 67 9.3 Optimization & Code Generation . 67 9.3.1 Serial Optimizations . 68 9.3.2 Multicore-specific Optimizations and Code Generation . 69 9.3.3 CUDA-specific Optimizations and Code Generation . 70 9.4 Auto-Tuning Strategy Engine . 70 9.5 Performance Evaluation . 72 9.5.1 Auto-Parallelization Performance . 72 9.5.2 Performance Expectations . 72 9.5.3 Performance Portability . 76 9.5.4 Programmer Productivity Benefits . 77 9.5.5 Architectural Comparison . 77 9.6 Limitations . 77 9.7 Summary . 78 10 Sepya: An Embedded Domain-Specific Auto-tuning Compiler for Structured Grids 79 10.1 Analysis-Avoiding DSEL for Structured Grids . 80 10.1.1 Building Blocks of Structured Grid Calculations . 80 10.1.2 Language and Semantics . 81 10.1.3 Avoiding Analysis . 83 10.1.4 Language in Python Constructs . 83 10.2 Structure of the Sepya Compiler . 85 10.3 Implemented Code Generation Algorithms & Optimizations . 86 10.3.1 Auto-tuning . 87 10.3.2 Data Structure . 87 10.4 Evaluation . 88 10.4.1 Test kernels & Other DSL systems . ..
Details
-
File Typepdf
-
Upload Time-
-
Content LanguagesEnglish
-
Upload UserAnonymous/Not logged-in
-
File Pages181 Page
-
File Size-