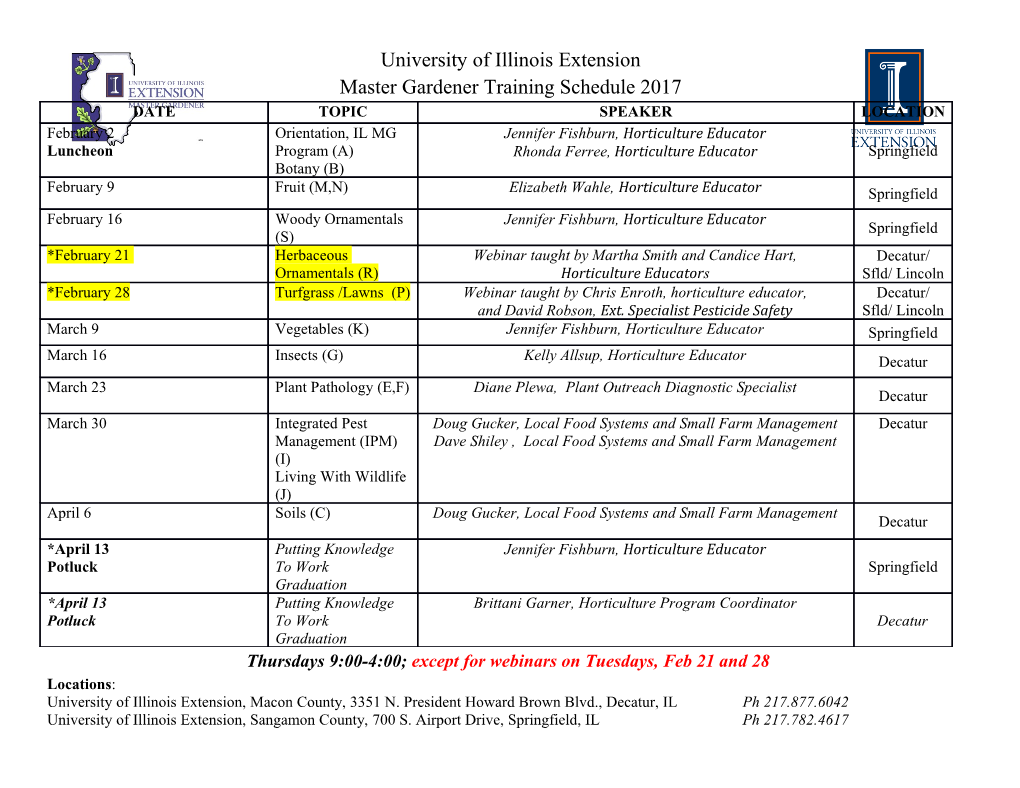
ST 370 Probability and Statistics for Engineers Discrete Random Variables A random variable is a numerical value associated with the outcome of an experiment. Discrete random variable When we can enumerate the possible values of the variable (such as 0, 1, 2, . ), the random variable is discrete. Example: acceptance sampling Suppose that a sample of size 10 is drawn from a shipment of 200 items, of which some number are non-compliant; X is the number of non-compliant items in the sample. The possible values of X are 0, 1, 2, . , 10, so X is a discrete random variable. 1 / 15 Discrete Random Variables ST 370 Probability and Statistics for Engineers Continuous random variable When the variable takes values in an entire interval, the random variable is continuous. Example: flash unit recharge time Suppose that a cell phone camera flash is chosen randomly from a production line; the time X that it takes to recharge is a positive real number; X is a continuous random variable. Presumably, there is some lower bound a > 0 that is the shortest possible recharge time, and similarly some upper bound b < 1 that is the longest possible recharge time; however, we usually do not know these values, and we would just say that the possible values of X are fx : 0 < x < 1g. 2 / 15 Discrete Random Variables ST 370 Probability and Statistics for Engineers Probability distribution The probability distribution of a random variable X is a description of the probabilities associated with the possible values of X . The representation of a probability distribution is different for discrete and continuous random variables. Probability mass function For a discrete random variable, the simplest representation is the probability mass function (pmf) fX (x) = P(X = x) where x is any possible value of X . 3 / 15 Discrete Random Variables Probability distribution ST 370 Probability and Statistics for Engineers Example: acceptance sampling Suppose one item is chosen at random from a shipment of 200 items, of which 5 are non-compliant. Let ( 1 if the item is non-compliant, X = 0 if the item is compliant. We could say that X is the number of non-compliant items seen. The probability mass function of X is ( 0:975 x = 0 fX (x) = 0:025 x = 1 A random variable like X that takes only the values 0 and 1 is called a Bernoulli random variable. 4 / 15 Discrete Random Variables Probability distribution ST 370 Probability and Statistics for Engineers Example: Dice Suppose you roll a fair die, and the number of spots showing is X . Then X is a discrete random variable with probability mass function 1 f (x) = ; x = 1; 2; 3; 4; 5; 6: X 6 Because the probability is the same for all the possible values of X , it is called the discrete uniform distribution. Properties of the probability mass function They are probabilities: fX (x) ≥ 0. P They cover all possibilities: x fX (x) = 1. 5 / 15 Discrete Random Variables Probability distribution ST 370 Probability and Statistics for Engineers Cumulative distribution function As an alternative to the probability mass function, the probability distribution of a random variable X can be defined by its cumulative distribution function (cdf) FX (x) = P(X ≤ x); −∞ < x < 1: In terms of the probability mass function: X FX (x) = fx (xi ); −∞ < x < 1: xi ≤x FX (·) has a jump at each possible value xi of X , and the jump equals the corresponding probability fX (xi ), so the probability mass function can be obtained from the cumulative distribution function. 6 / 15 Discrete Random Variables Cumulative distribution function ST 370 Probability and Statistics for Engineers Example: acceptance sampling 8 0 x < 0 <> FX (x) = 0:975 0 ≤ x < 1 :>1 x ≥ 1 curve(pbinom(x, 1, .025), from = -1, to = 2) 7 / 15 Discrete Random Variables Cumulative distribution function ST 370 Probability and Statistics for Engineers Example: dice 8 >0 x < 1 > >1=6 1 ≤ x < 2 <> FX (x) = 2=6 2 ≤ x < 3 >. >. > :1 x ≥ 6 curve(pmax(0, pmin(1, floor(x)/6)), from = 0, to = 7) 8 / 15 Discrete Random Variables Cumulative distribution function ST 370 Probability and Statistics for Engineers Mean and Variance Mean value The mean value, or expected value, of a discrete random variable with probability mass function fX (·) is X µX = E(X ) = xfX (x): x E(X ) is a weighted average of the possible values of X , each weighted by the corresponding probability. The expected value E(X ) is a typical value of the random variable X , in the same way that a sample meanx ¯ is a typical value of the sample x1; x2;:::; xn. 9 / 15 Discrete Random Variables Mean and Variance ST 370 Probability and Statistics for Engineers Example: acceptance sampling One item is chosen at random from a shipment of 200 items, of which 5 are non-compliant, and X is the number of non-compliant items seen: E(X ) = 0 × fX (0) + 1 × fX (1) = 0:025: For any Bernoulli random variable X , E(X ) = P(X = 1): 10 / 15 Discrete Random Variables Mean and Variance ST 370 Probability and Statistics for Engineers Example: Dice Suppose you roll a fair die, and the number of spots showing is X : E(X ) = 1 × fX (1) + 2 × fX (2) + ··· + 6 × fX (6) = (1 + 2 + 3 + 4 + 5 + 6)=6 = 3:5: Note In these examples and in many others, the \expected" value is not one of the possible values of the random variable; this is not the paradox that it is sometimes made out to be! 11 / 15 Discrete Random Variables Mean and Variance ST 370 Probability and Statistics for Engineers Variance Suppose that X is a random variable with expected value µX . Then 2 Y = (X − µX ) is another random variable, and its expected value is X E(Y ) = yfY (y) y X 2 = (x − µX ) fX (x): x 12 / 15 Discrete Random Variables Mean and Variance ST 370 Probability and Statistics for Engineers 2 The variance of X is E(Y ) = E[(X − µX ) ]: 2 2 σX = V (X ) = E (X − µX ) : The standard deviation of X is q 2 σX = σX : 13 / 15 Discrete Random Variables Mean and Variance ST 370 Probability and Statistics for Engineers Example: acceptance sampling For any Bernoulli random variable X , µX = P(X = 1) = p; say; so 2 2 2 σX = (0 − p) × P(X = 0) + (1 − p) × P(X = 1) = p2(1 − p) + (1 − p)2p = p(1 − p) and p σX = p(1 − p): 14 / 15 Discrete Random Variables Mean and Variance ST 370 Probability and Statistics for Engineers Example: Dice 2 2 x x − 3:5 (x − 3:5) fX (x)(x − 3:5) fX (x) 1 1 -2.5 6.25 6 1.0417 1 2 -1.5 2.25 6 0.3750 1 3 -0.5 0.25 6 0.0417 1 4 0.5 0.25 6 0.0417 1 5 1.5 2.25 6 0.3750 1 6 2.5 6.25 6 1.0417 Total: 2.9168 2 So σX = 2:917 and σX = 1:708. 15 / 15 Discrete Random Variables Mean and Variance.
Details
-
File Typepdf
-
Upload Time-
-
Content LanguagesEnglish
-
Upload UserAnonymous/Not logged-in
-
File Pages15 Page
-
File Size-