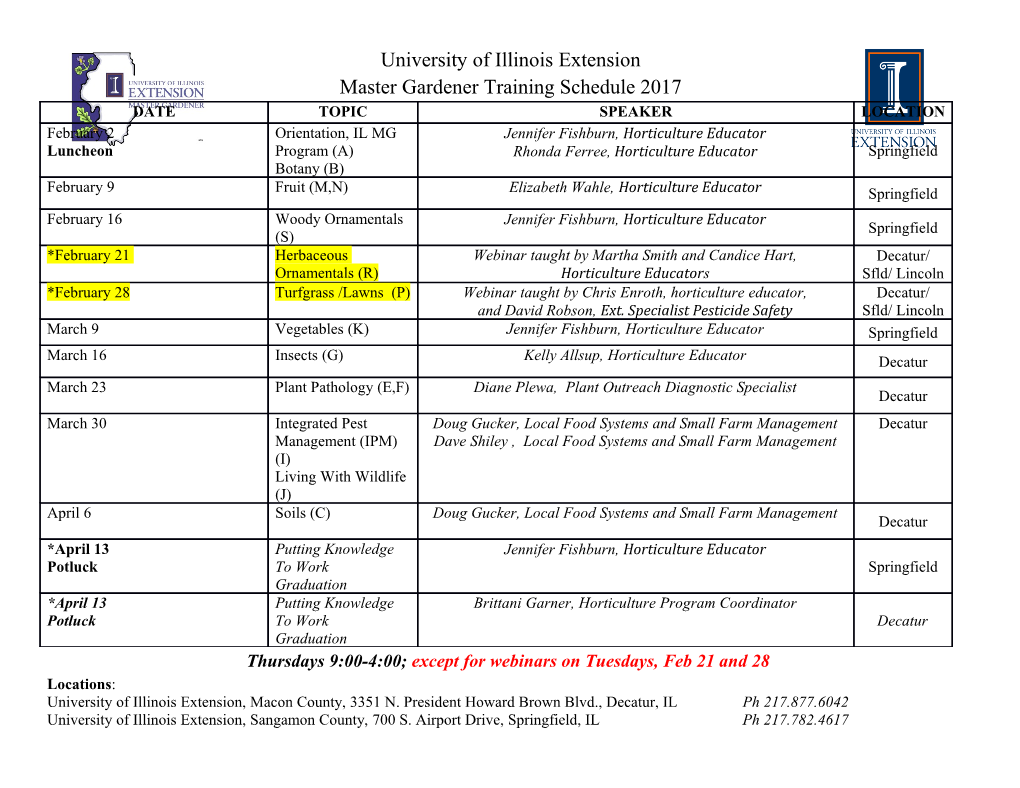
Spatial Transition Learning on Road Networks with Deep Probabilistic Models Xiucheng Liy, Gao Congy, Yun Chengz y Nanyang Technological Univeristy, z ETH Zurich fxli055@e., [email protected], [email protected] TABLE I Abstract—In this paper, we study the problem of predicting the EXAMPLE OF TRIPS ON ROAD NETWORK. most likely traveling route on the road network between two given locations by considering the real-time traffic. We present a deep Route Destination Frequency probabilistic model–DeepST–which unifies three key explanatory factors, the past traveled route, the impact of destination and r3 ! r2 ! r4 C 400 real-time traffic for the route decision. DeepST explains the r3 ! r2 ! r5 ! r10 C 100 generation of next route by conditioning on the representations r1 ! r2 ! r5 ! r6 A 100 of the three explanatory factors. To enable effectively sharing r1 ! r2 ! r7 ! r8 B 100 r ! r ! r ! r ! r B 100 the statistical strength, we propose to learn representations of 1 2 5 6 9 K-destination proxies with an adjoint generative model. To incorporate the impact of real-time traffic, we introduce a high C r<latexit sha1_base64="(null)">(null)</latexit> 10 r<latexit sha1_base64="(null)">(null)</latexit> dimensional latent variable as its representation whose posterior r<latexit sha1_base64="(null)">(null)</latexit> 4 6 A r<latexit sha1_base64="lQIphb3ZxX5RFxPPJ2+0wE0HOKM=">AAAB6nicbZBNS8NAEIYn9avWr6hHL4tF8FQSEfRY9OKxov2ANpTNdtMu3WzC7kQooT/BiwdFvPqLvPlv3LY5aOsLCw/vzLAzb5hKYdDzvp3S2vrG5lZ5u7Kzu7d/4B4etUySacabLJGJ7oTUcCkUb6JAyTup5jQOJW+H49tZvf3EtRGJesRJyoOYDpWIBKNorQfd9/tu1at5c5FV8AuoQqFG3/3qDRKWxVwhk9SYru+lGORUo2CSTyu9zPCUsjEd8q5FRWNugny+6pScWWdAokTbp5DM3d8TOY2NmcSh7YwpjsxybWb+V+tmGF0HuVBphlyxxUdRJgkmZHY3GQjNGcqJBcq0sLsSNqKaMrTpVGwI/vLJq9C6qPmW7y+r9ZsijjKcwCmcgw9XUIc7aEATGAzhGV7hzZHOi/PufCxaS04xcwx/5Hz+AAKOjZo=</latexit> 1 r<latexit sha1_base64="(null)">(null)</latexit> 5 distribution can then be inferred from observations. An efficient r<latexit sha1_base64="(null)">(null)</latexit> 2 inference method is developed within the Variational Auto- r<latexit sha1_base64="(null)">(null)</latexit> 9 r<latexit sha1_base64="(null)">(null)</latexit> Encoders framework to scale DeepST to large-scale datasets. r<latexit sha1_base64="(null)">(null)</latexit> 3 7 B r<latexit sha1_base64="(null)">(null)</latexit> 8 We conduct experiments on two real-world large-scale trajectory (a) datasets to demonstrate the superiority of DeepST over the a3 existing methods on two tasks: the most likely route prediction r<latexit sha1_base64="qnNvr3D2n/FISfqvn16bzQA5SxE=">AAAB83icbVDLSsNAFL2pr1pfVZduBovgqiQi6LLqxmUF+4AmlMl00g6dTMLMjVBCf8ONC0Xc+jPu/BunbRbaemDgcM693DMnTKUw6LrfTmltfWNzq7xd2dnd2z+oHh61TZJpxlsskYnuhtRwKRRvoUDJu6nmNA4l74Tju5nfeeLaiEQ94iTlQUyHSkSCUbSS78cUR2GU62n/pl+tuXV3DrJKvILUoECzX/3yBwnLYq6QSWpMz3NTDHKqUTDJpxU/MzylbEyHvGepojE3QT7PPCVnVhmQKNH2KSRz9fdGTmNjJnFoJ2cZzbI3E//zehlG10EuVJohV2xxKMokwYTMCiADoTlDObGEMi1sVsJGVFOGtqaKLcFb/vIqaV/UPbfuPVzWGrdFHWU4gVM4Bw+uoAH30IQWMEjhGV7hzcmcF+fd+ViMlpxi5xj+wPn8ATl2kco=</latexit> A a1 a2 a4 a5 and route recovery from sparse trajectories. In particular, on r<latexit sha1_base64="JA+62BsCQpkLtyvYrKFwAKuz3RI=">AAAB83icbVDLSsNAFL2pr1pfVZduBovgqiQi6LLUjcsK9gFNKJPpTTt0MgkzE6GE/oYbF4q49Wfc+TdO2yy09cDA4Zx7uWdOmAqujet+O6WNza3tnfJuZW//4PCoenzS0UmmGLZZIhLVC6lGwSW2DTcCe6lCGocCu+Hkbu53n1BpnshHM00xiOlI8ogzaqzk+zE14zDK1WzQHFRrbt1dgKwTryA1KNAaVL/8YcKyGKVhgmrd99zUBDlVhjOBs4qfaUwpm9AR9i2VNEYd5IvMM3JhlSGJEmWfNGSh/t7Iaaz1NA7t5DyjXvXm4n9ePzPRbZBzmWYGJVseijJBTELmBZAhV8iMmFpCmeI2K2FjqigztqaKLcFb/fI66VzVPbfuPVzXGs2ijjKcwTlcggc30IB7aEEbGKTwDK/w5mTOi/PufCxHS06xcwp/4Hz+ADr6kcs=</latexit> B one public large-scale trajectory dataset, DeepST surpasses the best competing method by almost 50% on the most likely route (b) prediction task and up to 15% on the route recovery task in terms of accuracy. Fig. 1. (a) Road network. (b) Example of route recovery from sparse trajectory, in which the grey bars indicate building blocks and r , r NTRODUCTION A B I. I represent two possible routes. In this paper, we study the problem, given the origin and destination, of predicting the most likely traveling route on a road network as well as outputing a probability value to that the transition patterns of vehicles are often highly skewed: indicate the likelihood of a route that being traveled. The some routes are more likely to be traveled than others. How- problem finds applications in a variety of downstream tasks, ever, to reliably model such transition patterns, we argue that such as taxi ridesharing schedule, route recovery from sparse three key explanatory factors need to be carefully taken into trajectories [1], [2], and popular routes recommendation [3]. consideration. We illustrate these key factors with an example; As a motivating example, in taxi dispatch system the origin assuming we have observed 8 trips described in Table I, over and destination are usually given before the start of a trip, a road network showed in Figure 1a. and thus predicting the most likely route could help us better First, the spatial transition patterns demonstrate strong se- arrange the taxi sharing by picking up the potential passengers quential property. Consider that we try to predict the next that are waiting on or nearby the most likely traveled route. transition of a vehicle driving on r2. According to the historical Furthermore, if we could score the likelihood of a route being trips, P(r4 r2) = 4=8 is greater than P(r5 r2) = 3=8 and j j traveled, we can also recover the underlying route from sparse P(r7 r2) = 1=8. We have a high confidence to predict that j trajectories. The route recovery problem [1], [2] arises in the vehicle will transit to r4. However, if we also know that the real-world trajectories due to the low-sampling-rates or the traveled road sequence is r r , the prediction will 1 ! 2 turning off of location-acquisition devices. Figure 1b shows then favor r5 over r4. Second, the trip destination has a global two possible routes rA, rB from a3 to a4 in an observed impact on the transition. Now consider that a vehicle is driving trajectory Ta = [a1; a2; : : : ; a5]. If we treat a3, a4 as the origin on r5 and the trip destination is C. Based on the historical and destination respectively, we could score the likelihood of trips it will have a higher probability of transiting to r6 than the two candidate routes to help infer the truly traveled route. transiting to r10 since P(r6 r5) = 2=3 > P(r10 r5) = 1=3: But The problem addressed in this paper can be summarized if we take into considerationj the trip destination,j we would as modeling the spatial transition patterns of real-world trips predict the next road to be r10 as P(r10 r5;C) = 1. Third, on the road network. Several proposals [4], [5] have revealed the route choices are also influenced by thej real-time traffic. The vehicle drivers tend to choose those less congested routes For the first time, we develop a novel deep probabilistic • rather than the shortest one. Again, assuming that a vehicle model–DeepST– to learn the spatial transition patterns, is on r2 and the trip destination is B. The historical trips which simultaneously takes into consideration the transi- show that it has equal probability of transiting to r5 or r7 tion sequential property, the impact of destinations and as P(r5 r2;B) = P(r7 r2;B) = 1=2. However, if the traffic real-time traffic. on r ; rj is more congestedj than that on r ; r ; r , the driver We propose a novel adjoint generative model to learn the 7 8 5 6 9 • is more likely to choose r5 instead of r7 even if the route K-destination proxies, which enables effectively sharing r7 r8 is shorter. statistical strength across trips and the resulting model is It! is challenging to unify all these factors into a single robust to inaccurate destinations. model. To capture the sequential property, the authors [5] We develop an efficient inference method that scales the • propose to model the spatial transition patterns using the model to large-scale datasets within the VAEs framework. Hidden Markov Model (HMM) which requires explicit de- We conduct experiments on two real-world datasets to • pendency assumptions to make inference tractable [6]. Wu et demonstrate the superiority of DeepST over existing al. [7] consider the impact of destination on the route decision methods on two tasks: predicting the most likely routes by treating each destination separately, thus failing to share and route recovery from sparse trajectories. statistical strength across trips with nearby destinations. In addition, they assume that accurate ending streets of the trips II. RELATED WORK are available, and use the corresponding road segments to help We briefly review the related work on spatial transition make decision. However, in some applications we may only modeling on the road network and deep probabilistic models. have the rough destination coordinates at hand e.g., the driver may not end a trip on the exact street as the user requested in A. Spatial transition modeling on road network the taxi dispatch system. To incorporate the influence of traffic The spatial transition modeling on the road network in when learning the transition patterns, [2], [8] assume that the literature arises in the following area: sparse trajectory traffic conditions in the same time slot (e.g., 7:00am-8:00am similarity computation, route recovery from sparse trajectories, every weekday) are temporally-invariant, which is not real- and future movement prediction. time traffic and may not hold. To our knowledge, no existing work on spatial transition
Details
-
File Typepdf
-
Upload Time-
-
Content LanguagesEnglish
-
Upload UserAnonymous/Not logged-in
-
File Pages12 Page
-
File Size-