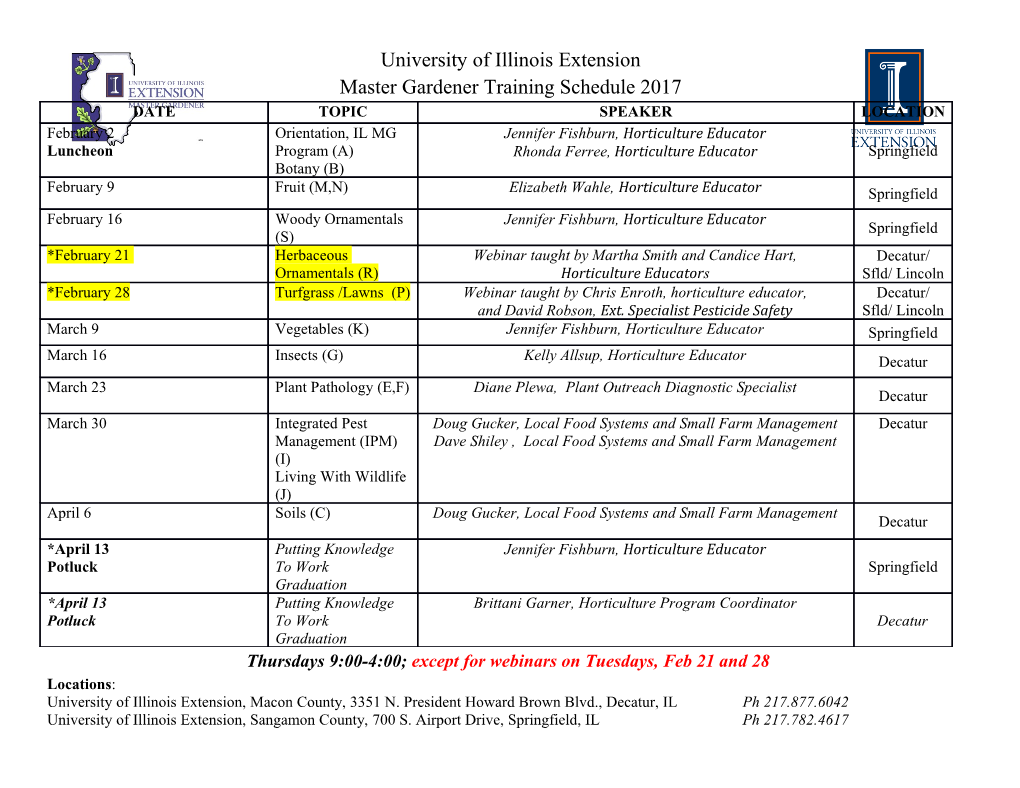
BIOT 1 In silico design of integrated downstream processes for non-mAb biologics produced in complex expression systems Nicholas Vecchiarello2, [email protected], Chaz Goodwine3, Steven M. Cramer1. (1) Ricketts Bldg, Rensselaer Polytechnic Inst, Troy, New York, United States (2) Rensselaer Polytechnic Institute, Troy, New York, United States (3) Chemical and Biological Engineering, Rensselaer Polytechnic Institute, Troy, New York, United States For biomolecules not amenable to affinity capture, determining optimal downstream processes can be a major challenge due to the broad range of experimental design space available with regard to resins, buffer pH, and salt. Previous work in our group has addressed this issue for products produced in Pichia pastoris by chromatographically characterizing process-related impurities found in spent cultivation fluids on a set of multimodal, HCIC, and ion exchange resins for a wide range of conditions using UP-RPLC. The collected data was used in concert with product retention data and a custom in silico tool to generate and rank integrated downstream processes for three non-monoclonal antibody products expressed in P. pastoris. In this work, we have chromatographically characterized the host-related impurity behavior for a CHO cell line containing a more significant HCP burden. In an effort to expedite process development, a method for assessing global orthogonal selectivity for different process-related impurities between resin pairs was employed in order to prune the number of resins required for screening. This strategy was then applied to the purification of a product with a significant variant challenge. To further reduce the number of resins to screen for product retention, sequence understanding and biophysical properties were utilized to focus on regions that would likely remove host- related impurities and offer variant selectivity. Product and variant retention data from these focused screens were used as initial inputs for the in silico tool and a fully inclusive list of integrated downstream processes was generated and ranked based on overall purification sequence orthogonality. Top process outputs were then subjected to experimental process development and refinement. This approach represents a strategy for rapidly designing integrated downstream processes in an efficient manner for non-mAb biologics produced in complex host expression systems. BIOT 2 Streamlining early DSP development through evolving integrated mechanistic models Alexander T. Hanke, [email protected], Rushd Khalaf, Lars W. Pampel. BTDM, Novartis Pharma AG, Basel, Switzerland Efficient development of chromatographic purification processes requires knowledge on how the choice of resin, buffers and operational parameters influence the behavior of both the product and its impurities. High-throughput systems capable of performing batch adsorption and parallel micro-column experiments have become staples of most process development labs due to their ability to rapidly explore this large potential process space and identify promising conditions. Even with such technologies in place it remains a challenging task for developers to find the right combination of operations to robustly and efficiently meet quality expectations. Due to the non-linear nature of preparative chromatography and changes is the upstream process during the project lifecycle, large amounts of the data generated during early process screenings are rendered obsolete once the process intermediate they were generated with is no longer representative of what the step will be challenged with in the final process. We present how predictive adsorption models can reduce this waste by narrowing screening spaces to specific windows of interest tailored to individual molecules. First experimental results generated within these windows serve to correct first prediction errors and can be fed directly into mechanistic models integrating all potential operation sequences. As these models are refined during development they allow to assess the impact of and intermediate composition changes and non-linearity effects, effectively reducing the experimental and analytical burden of conventional sequential process development. BIOT 3 Using a Bayesian framework to account for scale-down model offsets during process characterization Matthew Stork1, [email protected], Aili Cheng2, Brad Evans2, Peter Slade1, Erwin Yu1. (1) Bioprocess Research and Development, Pfizer, Inc, Andover, Massachusetts, United States (2) Non Clinical Statistics, Pfizer, Andover, Massachusetts, United States One of the primary challenges during process characterization is establishing the suitability of the small scales models used to generate the data. The traditional approach to qualification of scale-down models has been to verify that lab-scale results match large-scale results to within an arbitrary precision. An alternate approach is to directly incorporate scaling offsets into predictive models. However, obtaining a precise estimate of scaling offsets can be difficult when large-scale datasets are relatively small. As such, it is essential to use a statistical method that can account for this uncertainty. This talk will explore the use of Bayesian modeling to account for scaling offsets in predictive models. Bayesian methods are well-suited to this task because they inherently account for uncertainty, while offering a flexible platform for the implementation of hierarchal models. This talk will explore the use of Bayesian statistics for both upstream and downstream applications. In a downstream application, Bayesian statistics were used to account for scale-offsets in a high-throughput chromatography system using miniature chromatography columns. In the past, concerns about the scalability of high-throughput methods have been a barrier to using high-throughput data in process characterization models. Using Bayesian techniques, chromatography data from high-throughput and traditional lab-scale DOE studies were combined with manufacturing-scale data to generate models accounting for scaling-offsets. In an upstream application, a hierarchal Bayesian model was used to generate a predictive model to account for scaling offsets in bioreactor performance parameters. Bioreactor scale-up is not a simple linear process, and a hierarchal Bayesian approach enables an assessment of how the effects of input process parameters may differ across scales. Overall, the Bayesian approach is a flexible method that facilitates more informed predictions of large-scale performance by enabling the incorporation of scaling offsets and prior knowledge into predictive models. BIOT 4 Mechanistic modeling analysis of chromatography scale-down models Steven Benner, [email protected], John Welsh, Michael Rauscher, Jennifer Pollard. Merck, Kenilworth, New Jersey, United States Chromatography has been a cornerstone of downstream process development (PD) for years, and there is an ever increasing demand for improved speed and efficiency. Scale-down models are used in process development to optimize operating conditions and study process robustness while expending as little time and material as possible. The advent of automated liquid handling systems and miniature columns has taken the efficiency of process development to another level by allowing up to eight column runs in parallel with column volumes under 1 ml. However results between these miniature columns and typical lab scale columns can deviate, resulting in the need for a better understanding of the differences between columns and systems. Mechanistic models can be used to understand the physics of the process (fluid flow, mass transfer, etc.) as a function of scale. We have used mechanistic modeling to study the factors leading to differences in pool sizes observed between scales, and to make predictions on lab scale pool sizes from miniature column data. Results indicate that changes in mass transfer parameters, specifically axial dispersion and film mass transfer rates, between scales leads to the observed differences in pool size. Additionally, we have studied the effect of system differences between automated liquid handling systems and conventional preparative chromatography systems on process performance. This work provides new insight on the ability of high-throughput process development to be used for scale down modeling and process characterization. BIOT 5 In-silico model formulation, calibration and application for commercial CEX chromatography Christian Kunert3, [email protected], Fabrice Schlegel1, Karin Westerberg3, Oliver Kaltenbrunner2, Pablo Rolandi4, Xiaoxiang Zhu5. (1) Process Development, Amgen, Cambridge, Massachusetts, United States (2) Amgen Inc, Thousand Oaks, California, United States (3) Amgen, Cambridge, Massachusetts, United States (4) PD, Amgen, Cambridge, Massachusetts, United States Cation exchange chromatography (CEX) is an important step in the purification of some biologics such as monoclonal antibodies (mAb). Commercial Process Development and Process Characterization require a large set of experiments to establish desirable operating conditions. Lately, the biopharma industry has shifted toward developing mechanistic numerical models that can be calibrated with a limited set of experimental runs and are able to accurately predict process conditions. These mechanistic models enable the characterization of process robustness and help establishing a design space that ensures product quality by the means of high performance
Details
-
File Typepdf
-
Upload Time-
-
Content LanguagesEnglish
-
Upload UserAnonymous/Not logged-in
-
File Pages393 Page
-
File Size-