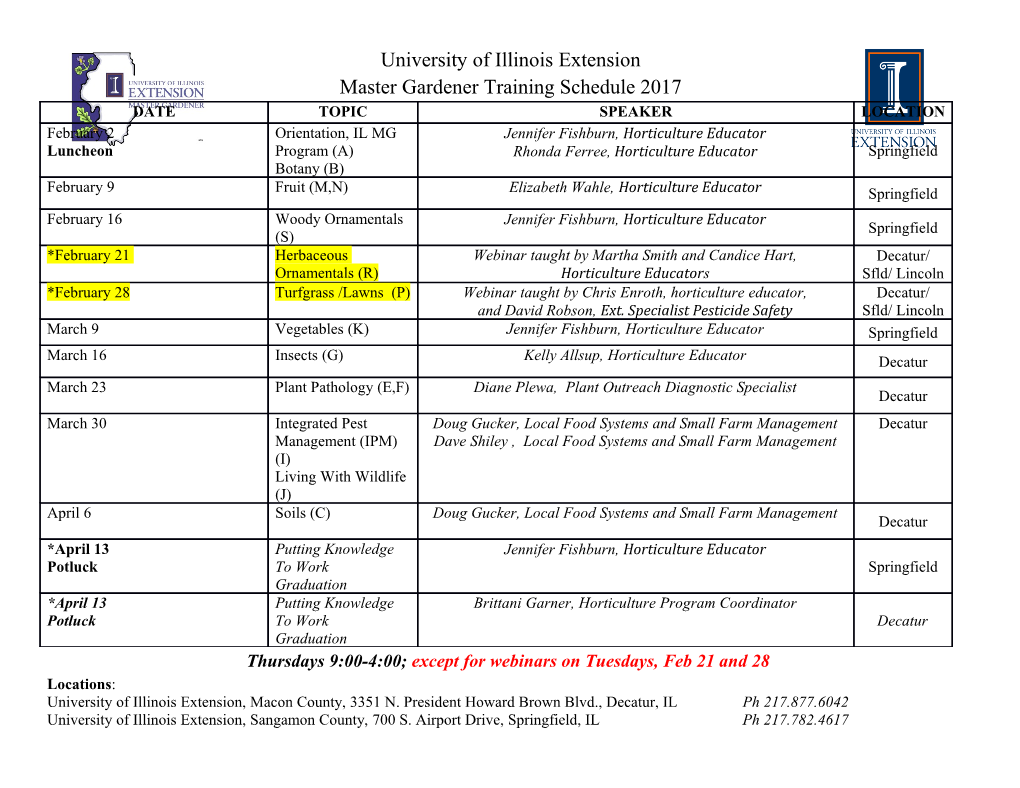
Introduction to Machine Learning Lecture 9 Mehryar Mohri Courant Institute and Google Research [email protected] Kernel Methods Motivation Non-linear decision boundary. Efficient computation of inner products in high dimension. Flexible selection of more complex features. Mehryar Mohri - Introduction to Machine Learning page 3 This Lecture Definitions SVMs with kernels Closure properties Sequence Kernels Mehryar Mohri - Introduction to Machine Learning page 4 Non-Linear Separation Linear separation impossible in most problems. Non-linear mapping from input space to high- dimensional feature space: Φ : X F . → Generalization ability: independent of dim( F ) , depends only on ρ and m . Mehryar Mohri - Introduction to Machine Learning page 5 Kernel Methods Idea: Define K : X X R , called kernel, such that: • × → Φ(x) Φ(y)=K(x, y). · • K often interpreted as a similarity measure. Benefits: • Efficiency: K is often more efficient to compute than Φ and the dot product. • Flexibility: K can be chosen arbitrarily so long as the existence of Φ is guaranteed (symmetry and positive definiteness condition). Mehryar Mohri - Introduction to Machine Learning page 6 PDS Condition Definition: a kernel K : X X R is positive definite × → symmetric (PDS) if for any x 1 ,...,x m X , the {m m }⊆ matrix K =[ K ( x i ,x j )] ij R × is symmetric ∈ positive semi-definite (SPSD). K SPSD if symmetric and one of the 2 equiv. cond.’s: its eigenvalues are non-negative. • n m 1 for any c R × , cKc = cicjK(xi,xj) 0. • ∈ ≥ i,j=1 Terminology: PDS for kernels, SPSD for kernel matrices (see (Berg et al., 1984)). Mehryar Mohri - Introduction to Machine Learning page 7 Example - Polynomial Kernels Definition: N d x, y R ,K(x, y)=(x y + c) ,c>0. ∀ ∈ · Example: for N =2 and d =2 , 2 K(x, y)=(x1y1 + x2y2 + c) 2 2 x1 y1 2 2 x2 y2 √2 x x √2 y y = 1 2 1 2 . √2cx1 · √2cy1 √2cx √2cy 2 2 c c Mehryar Mohri - Introduction to Machine Learning page 8 XOR Problem Use second-degree polynomial kernel with c =1 : √2 x1x2 x2 (-1, 1) (1, 1, +√2, √2, √2, 1) (1, 1, +√2, +√2, +√2, 1) (1, 1) − − √2 x1 x1 (-1, -1) (1, -1) (1, 1, √2, √2, +√2, 1) (1, 1, √2, +√2, √2, 1) − − − − Linearly non-separable Linearly separable by x1x2 =0 . Mehryar Mohri - Introduction to Machine Learning page 9 Other Standard PDS Kernels Gaussian kernels: x y 2 K(x, y)=exp || − || ,σ=0. − 2σ2 Sigmoid Kernels: K(x, y)=tanh(a(x y)+b),a,b 0. · ≥ Mehryar Mohri - Introduction to Machine Learning page 10 This Lecture Definitions SVMs with kernels Closure properties Sequence Kernels Mehryar Mohri - Introduction to Machine Learning page 11 Reproducing Kernel Hilbert Space (Aronszajn, 1950) Theorem: Let K : X X R be a PDS kernel. Then, × → there exists a Hilbert space H and a mapping Φ from X to H such that x, y X, K(x, y)=Φ(x) Φ(y). ∀ ∈ · Furthermore, the following reproducing property holds: f H , x X, f(x)= f,Φ(x) = f,K(x, ) . ∀ ∈ 0 ∀ ∈ · Mehryar Mohri - Introduction to Machine Learning page 12 Notes: • H is called the reproducing kernel Hilbert space (RKHS) associated to K . A Hilbert space such that there exists Φ : X H • → with K ( x, y )= Φ ( x ) Φ ( y ) for all x, y X is also · ∈ called a feature space associated to K . Φ is called a feature mapping. • Feature spaces associated to K are in general not unique. Mehryar Mohri - Introduction to Machine Learning page 13 Consequence: SVMs with PDS Kernels (Boser, Guyon, and Vapnik, 1992) Constrained optimization: Φ(xi) Φ(xj) m m · 1 max αi αiαj yiyjK(xi,xj ) α − 2 i=1 i,j=1 m subject to: 0 α C α y =0,i [1,m]. ≤ i ≤ ∧ i i ∈ i=1 Solution: Φ(xi) Φ(x) m · h(x)=sgn αiyiK(xi,x)+b , Φ(xj ) Φ(xi) m i=1 · with b = y α y K(x ,x ) for any x with i − j j j i i j=1 0<αi <C. Mehryar Mohri - Introduction to Machine Learning page 14 SVMs with PDS Kernels Constrained optimization: Hadamard product max 2 1α (α y)K(α y) α − ◦ ◦ subject to: 0 α C αy =0. ≤ ≤ ∧ Solution: m h =sgn α y K(x , )+b , i i i · i=1 with b = yi (α y)Kei for any x i with − ◦ 0<αi <C. Mehryar Mohri - Introduction to Machine Learning page 15 Generalization: Representer Theorem (Kimeldorf and Wahba, 1971) Theorem: Let K : X X R be a PDS kernel and H its corresponding RKHS.× → Then, for any non- m decreasing function G : R R and any L : R R + the optimization problem→ → ∪{ ∞} 2 argmin F (h)=argminG( h H )+L h(x1),...,h(xm) h H h H m ∈ ∈ admits a solution of the formh∗ = α K(x , ). i i · i=1 If G is further assumed to be increasing, then any solution has this form. Mehryar Mohri - Introduction to Machine Learning page 16 Proof: let H =span( K ( x , ): i [1 ,m ] ). Any h H • 1 { i · ∈ } ∈ admits the decomposition h = h 1 + h ⊥ according to H = H H ⊥ . 1 ⊕ 1 • Since G is non-decreasing, 2 2 2 2 G( h ) G( h + h⊥ )=G( h ). 1 ≤ 1 By the reproducing property, for all i [1 ,m ], • ∈ h(x )= h, K(x , ) = h ,K(x , ) = h (x ). i i · 1 i · 1 i • Thus, L h ( x 1 ) ,...,h ( x m ) = L h 1 ( x 1 ) ,...,h 1 ( x m ) and F (h ) F (h). 1 ≤ • If G is increasing, then F ( h 1 ) <F ( h ) and any solution of the optimization problem must be in H 1 . Mehryar Mohri - Introduction to Machine Learning page 17 Kernel-Based Algorithms PDS kernels used to extend a variety of algorithms in classification and other areas: • regression. • ranking. • dimensionality reduction. • clustering. But, how do we define PDS kernels? Mehryar Mohri - Introduction to Machine Learning page 18 This Lecture Definitions SVMs with kernels Closure properties Sequence Kernels Mehryar Mohri - Introduction to Machine Learning page 19 Closure Properties of PDS Kernels Theorem: Positive definite symmetric (PDS) kernels are closed under: • sum, • product, • tensor product, • pointwise limit, • composition with a power series. Mehryar Mohri - Introduction to Machine Learning page 20 Closure Properties - Proof Proof: closure under sum: cKc 0 cKc 0 c(K + K)c 0. ≥ ∧ ≥ ⇒ ≥ • closure under product: K = MM , m m m cicj(Kij Kij )= cicj MikMjk Kij i,j=1 i,j=1 k=1 m m m = c c M M K = zKz 0, i j ik jk ij k k ≥ i,j=1 k=1 k=1 c1M1k with z = . k ··· cmMmk Mehryar Mohri - Introduction to Machine Learning page 21 • Closure under tensor product: definition: for all x ,x ,y ,y X , • 1 2 1 2 ∈ (K K )(x ,y ,x ,y )=K (x ,x )K (y ,y ). 1 ⊗ 2 1 1 2 2 1 1 2 2 1 2 • thus, PDS kernel as product of the kernels (x1,y1,x2,y2) K1(x1,x2) (x ,y ,x ,y ) K (y ,y ). → 1 1 2 2 → 2 1 2 Closure under pointwise limit: if for all x, y X , • ∈ lim Kn(x, y)=K(x, y), n →∞ Then,( n, cKnc 0) lim cKnc = cKc 0. n ∀ ≥ ⇒ →∞ ≥ Mehryar Mohri - Introduction to Machine Learning page 22 • Closure under composition with power series: assumptions: K PDS kernel with K ( x, y ) < ρ for • n | | all x, y X and f ( x )= ∞ a n x ,a n 0 power ∈ n=0 ≥ series with radius of convergence ρ . f K is a PDS kernel since K n is PDS by closure • ◦ N n under product, n =0 a n K is PDS by closure under sum, and closure under pointwise limit. Example: for any PDS kernel K , exp( K ) is PDS. Mehryar Mohri - Introduction to Machine Learning page 23 This Lecture Definitions SVMs with kernels Closure properties Sequence Kernels Mehryar Mohri - Introduction to Machine Learning page 24 Sequence Kernels Definition: Kernels defined over pairs of strings. • Motivation: computational biology, text and speech classification. • Idea: two sequences are related when they share some common substrings or subsequences. • Example: sum of the product of the counts of common substrings. Mehryar Mohri - Introduction to Machine Learning page 25 Weighted Transducers b:a/0.6 b:a/0.2 a:b/0.1 1 a:a/0.4 b:a/0.3 2 3/0.1 0 a:b/0.5 T (x, y)= Sum of the weights of all accepting paths with input x and output y . T (abb, baa)=.1 .2 .3 .1+.5 .3 .6 .1 × × × × × × Mehryar Mohri - Introduction to Machine Learning page 26 Rational Kernels over Strings (Cortes et al., 2004) Definition: a kernel K : Σ ∗ Σ ∗ R is rational if K =T × → for some weighted transducer T . Definition: let T 1 : Σ ∗ ∆ ∗ R and T 2 : ∆ ∗ Ω ∗ R be two weighted transducers.× → Then, the composition× → of T and T is defined for all x Σ ∗ ,y Ω ∗ by 1 2 ∈ ∈ (T T )(x, y)= T (x, z) T (z,y). 1 ◦ 2 1 2 z ∆ ∈ ∗ Definition: the inverse of a transducer T : Σ ∗ ∆ ∗ R 1 × → is the transducer T − : ∆ ∗ Σ ∗ R obtained from T by swapping input and output× →labels. Mehryar Mohri - Introduction to Machine Learning page 27 Composition Theorem: the composition of two weighted transducer is also a weighted transducer. Proof: constructive proof based on composition algorithm. • states identified with pairs. • -free case: transitions defined by E = (q ,q ),a,c,w w , (q ,q ) .
Details
-
File Typepdf
-
Upload Time-
-
Content LanguagesEnglish
-
Upload UserAnonymous/Not logged-in
-
File Pages45 Page
-
File Size-