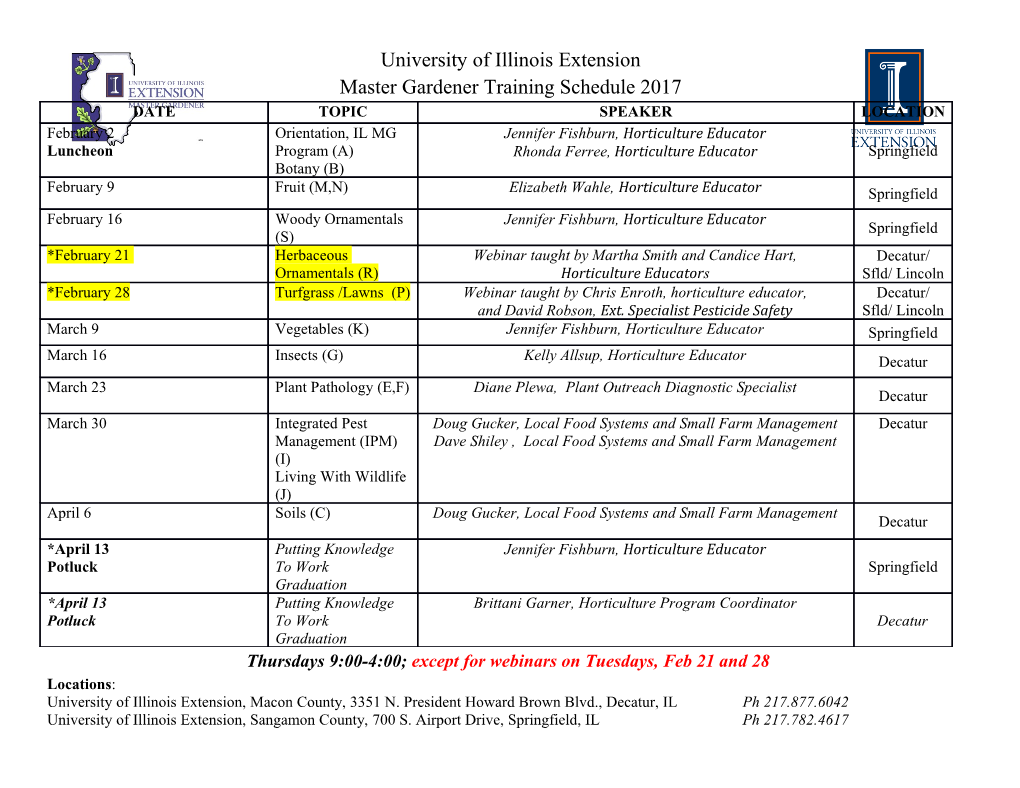
4. Linear Equations with sparse matrices 4.1 General properties of sparse matrices Full n x n – matrix: storage O(n2), solution O(n3) Æ too costly for most applications, especially for fine discretization (large n). Idea: Formulate the given problem in a clever way that leads to a linear system that is sparse: storage O(n), solution O(n)? (that is strucutured: storage O(n), solution O(n log(n)), FFT) (that is dense, but reduced from e.g.3D to 2D) Example: Tridiagonal matrix, banded matrix, block band matrix. 1 .⎛ 0 0 2 .⎞ 0 ⎜ ⎟ 3 .⎜ 4 . 0 5 .⎟ 0 Sparse example matrix 6A .= ⎜ 0 7 . 8 .⎟ , n 9 = .5, nnz = 12 ⎜ ⎟ 0 0⎜ 10 . 11⎟ . 0 ⎜ ⎟ 1 0⎝ 0 0 0⎠ 12 . 4.1.1 Storage in Coordinate Form values AA 12. 9. 7. 5. 1. 2. 11. 3. 6. 4. 8. 10. row JR5 332114 23234 columnJC5 534144 11243 To store: n, nnz, 2*nnz integer for row and column indices in JR and JC, and nnz float in AA. No sorting included. Redundant information. Code for computing c = A*b: for j = 1 : nnz(A) cJR(j) = CJR(j) + AA(j) * bJC(j) ; end aJR(j),JC(j) Disadvantage: Indirect addressing (indexing) in vector c and b Æ jumps in memory2 Advantage: No difference between columns and rows (A and AT), simple. 4.1.2 Compressed Sparse Row Format: CSR row 1 row 2 row 3 row 4 row 5 AA 2 1 5 3 4 6 7 8 9 10 11 12 Values JA 4 1 4 1 2 1 3 4 5 3 4 5 Column indices IA: 1 3 6 10 12 13 pointer to row i Storage: n, nnz, n+nnz+1 integer, nnz float. Code for computing c = A*b: for i = 1 : n for j = IA(i) : IA(i+1)-1 ci = ci + AA(j)*bJA(j) ; end end Indirect addressing only in b. Columnwise Æ compressed sparse column format3 CSR with extracted main diagonal main diagonal entries nondiagonal entries in CSR AA 1 4 7 11 12 * 2 3 5 6 8 9 10 JA 7 8 10 13 14 14 4 2 4 1 4 5 3 Pointer to begin of i-th row: Storage: n, nnz, nnz + 1 integer, nnz + 1 float for i = 1 : n ci = AAi * bi ; for j = JA(i) : JA(i+1)-1 ci = ci + AAj*bJA(j) ; end end 4 4.1.4 Diagonalwise storage 1⎛ 0 2 0⎞ 0 ⎜ ⎟ 3⎜ 4 0 5⎟ 0 A0= ⎜ 6 7 0⎟ 8 ⎜ ⎟ 0⎜ 0 9 0⎟ 0 ⎜ ⎟ 0⎝ 0 0 11⎠ 12 Diagonal number -1 0 2 *⎛ 1⎞ 2 ⎜ ⎟ 3⎜ 4⎟ 5 Values in: DIAG =6⎜ 7⎟ 8 , IOFF=1() − 0 2 ⎜ ⎟ 9⎜ 10⎟ * ⎜ ⎟ 11⎝ 12⎠ * Storage: n, nd = number of diagonals, nd integers orf IOFF, n*nd float 5 4.1.5 Rectangular Storage Scheme by Pressing from the Right 1 0 2 0 0 1⎛ 2⎞ 0 ⎜ ⎟ 3 4 0 5 0 3⎜ 4⎟ 5 0 6 7 0 8 gives COEF =6⎜ 7⎟ 8 ⎜ ⎟ 0 0 9 10 0 9⎜ 10⎟ 0 ⎜ ⎟ 0 0 0 11 12 11⎝ 12⎠ 0 1⎛ 3⎞ * ⎜ ⎟ 1⎜ 2⎟ 4 JCOEF =2⎜ 3⎟ 5 ⎜ ⎟ 3⎜ 4⎟ * ⎜ ⎟ nl := nnz of longest row. 4⎝ 5⎠ * Storage: n, n*nl integer and float 6 Code for c = A b: for i = 1 : n for j = 1 : nl ci = ci + COEFF(i,j) * b(JCOEFF(i,j)); end end This format was used in ELLPACK (package of subroutines for elliptic PDE). Coordinate form is used by MATLAB. 7 4.1.6 Jagged Diagonal Form Prestep: Sort rows after heirt length. Long rows first. 1⎛ 0 2 0⎞ 0 3⎛ 4 0 5⎞ 0 ⎜ ⎟ ⎜ ⎟ Length 3 3⎜ 4 0 5⎟ 0 0⎜ 6 7 0⎟ 8 A0= ⎜ 6 7 0⎟ 8⇒PA1⎜ = 0 2 0⎟ 0 ⎜ ⎟ ⎜ ⎟ 0⎜ 0 9 10⎟ 0 0⎜ 0 9 10⎟ 0Length 2 ⎜ ⎟ ⎜ ⎟ 0 0⎝ 0 11⎠ 12 0⎝ 0 0 11⎠ 12 Values3 of PA: 6DJ 1= ( 9 11 4 7 2 10 12) 5 8 First jagged diagonal second jagged diag. Column indices:12134JDIAG = ( 23345) 45 Pointer to beginning of j-th diagonal: IDIAG1= () 6 11 13 8 NDIAG = number of jagged diagonals Storage: n, NDIAG, nnz float, nnz + NDIAG integer Code for c = A b: for j = 1 : NDIAG for i = 1 : IDIAG(j+1) - IDIAG(j) Length of j-th jagged diag. k = IDIAG(j) + i - 1; ci = ci + DJ(k) * b(JDIAG(k)); end end Advantage: Always start with row 1. More operations on neighboring data. Prepermutation changes only rows. Can be done implicitly. 9 4.2 Sparse Matrices and Graphs 4.2.1 Graph G(A) for symmetric positive definite spd A=AT >0 n x n –matrix: vertices e1, … , en with edges (ei,ek) for aik ≠ 0 , undirected Graph *⎛ * 0⎞ * ⎜ ⎟ *⎜ * *⎟ 0 A = G(A): e e e e 0⎜ * *⎟ * 1 2 3 4 ⎜ ⎟ ⎜ ⎟ *⎝ 0 *⎠ * G(A) as directed graph: e1 e2 e3 e4 10 Adjacency Matrix for G(A) or A: 1⎛ 1 0⎞ 1 ⎜ ⎟ 1⎜ 1 1⎟ 0 AGA( ( ))= can be obtained directly by replacing 0⎜ 1 1⎟ 1 ⎜ ⎟ in A each nonzero entry by 1. ⎜ ⎟ 1⎝ 0 1⎠ 1 Symmetric permutations of A in the form P A PT change the ordering of the rows and columns of A simultaneously. Therefore, the graph of P A PT can be obtained by the graph of A by renumbering the vertices: Example: P permutation that changes 3 ÅÆ4: G(A): e1 e2 e3 e4 T G(PAP ): e1 e2 e4 e3 e1 e2 e3 e4 11 4.2.2 Matrix A nonsymmetric, G(A) undirected *⎛ * 0⎞ 0 1⎛ 1 0⎞ 0 ⎜ ⎟ ⎜ ⎟ 0⎜ * *⎟ 0 0⎜ 1 1⎟ 0 A = ⇒ AGA( ( ))= 0⎜ * *⎟ * 0⎜ 1 1⎟ 1 ⎜ ⎟ ⎜ ⎟ ⎜ ⎟ ⎜ ⎟ *⎝ 0 0⎠ * 1⎝ 0 0⎠ 1 G(A): e1 e2 e3 e4 How can we characterize „good“ sparsity patterns? „good“: Gaussian Elimination can eb reduced to smaller subproblems or produces no (or small) fill-in. 12 Block Diagonal Pattern *⎛ 0 *⎞ 0 1⎛ 0 1⎞ 0 ⎜ ⎟ ⎜ ⎟ 0⎜ * 0⎟ * 0⎜ 1 0⎟ 1 A = ⇒ AGA( ( ))= *⎜ 0 *⎟ 0 1⎜ 0 1⎟ 0 ⎜ ⎟ ⎜ ⎟ ⎜ ⎟ ⎜ ⎟ 0⎝ * 0⎠ * 0⎝ 1 0⎠ 1 G(A): e1 e2 e3 e4 *⎛ * 0⎞ 0 ⎜ ⎟ T *⎜ * 0⎟ 0⎛ A1 0 ⎞ 2 ÅÆ 3: e1 e3 e2 e4 PAP = = ⎜ ⎟ 0⎜ 0 *⎟ *⎜ 0 A ⎟ ⎜ ⎟ ⎝ 2 ⎠ ⎜ ⎟ 0⎝ 0 *⎠ * −1 By this permutation, A can be ⎛ A 0 ⎞ ⎛ A−1 0 ⎞ transformed into block diagonal ⎜ 1 ⎟ = ⎜ 1 ⎟ ⎜ ⎟ ⎜ −1 ⎟ form Æ easy to solve! ⎝ 0 A2 ⎠ ⎝ 0 A2 ⎠ 13 Banded Pattern ⎛a a ⎞ ⎜ 11 L 1p ⎟ MO⎜ O ⎟ ⎜ ⎟ aq1 O O A = ⎜ ⎟ ⎜ a ⎟ ⎜ O O n− p1 + , ⎟ n ⎜ O OM ⎟ ⎜ ⎟ ⎝ na, n− q + 1 L ann ⎠ Gauss Elimination without pivoting preserves het sparsity pattern ⎛l ⎞ u⎛ u ⎞ ⎜ 11 ⎟ ⎜ 11 L 1p ⎟ MO⎜ ⎟ ⎜ O O ⎟ ⎜ ⎟ ⎜ ⎟ lq1 O O O ⎜ ⎟ U = ⎜ ⎟ L = ⎜ ⎟ ⎜ ⎟ O nu− p1 + , n ⎜ OO ⎟ ⎜ ⎟ ⎜ O O ⎟ ⎜ OM ⎟ ⎜ ⎟ ⎜ ⎟ ⎝ unn ⎠ ⎝ nl, n− q + 1 L lnn ⎠ With pivoting the bandwidth in U grows, but remains <= p+q. 14 Overlapping Block Diagonal ⎛ ⎞ ⎜ ⎟ ⎜ ⎟ ⎜ ⎟ ⎜ ⎟ ⎜ ⎟ A = ⎜ ⎟ ⎜ ⎟ ⎜ ⎟ ⎜ ⎟ ⎜ ⎟ ⎜ ⎟ ⎝ ⎠ Pattern is preserved by Gaussian Elimination (with restricted pivoting). 15 Dissection Form Nested (recursive) dissection: 0 0 0 0 0 0 0 0 0 0 Pattern are preserved 0 during GE without 0 pivoting. No fill in 0 0 0 0 16 Schur Complement Reduction Idea: Write matrix B in terms of smaller submatrices: BB⎛ ⎞ BD⎛ −1 ⎞IBDBS⎛ + −1 ⎞ ! ⎛ I 0⎞ ⎜ 1 2⎟⋅⎜ 1 ⎟ = ⎜ 1 2 ⎟ =⎜ ⎟ ⎜ ⎟ ⎜ −1 ⎟ ⎜ −1 −1 ⎟ ⎜ ⎟ BB⎝ 3 4⎠ ⎝ 0 SBBBDBS⎠ ⎝ 3 1 3+ 4 ⎠ ⎝* I ⎠ To satisfy hist equation we aveh o set:t −1 −1 1 − BDBSDBBS+1 2 =0 ⇒ =1 2 − −1 −1 1 − −1 BDBSIIBBBSBS+3 4= ⇒ =3 1 − 2 + 4 −1 −1 1 − S B⇒ B = B4 3B − 1 2 and= − D1 2 B B S S Schur Complement 17 BB⎛ ⎞ ⎛ I 0⎞ BB⎛ ⎞ B = ⎜ 1 2⎟ = ⎜ ⎟⋅⎜ 1 2⎟ ⎜ ⎟ ⎜ −1 ⎟ ⎜ ⎟ BB⎝ 3 4⎠ BBI⎝ 3 1 ⎠ ⎝ 0 S ⎠ Therefore, solving linear system in B is reduced to solving wot smaller linear systems, one in B1 and the other in the Schur complement S. B sparse Æ B1 also sparse, but S usually dense! Example: Schur complement and dissection orm:f AF⎛ 0 ⎞ ⎜ 1 1 ⎟ A = ⎜ 0 AF2 2⎟ ⎜ ⎟ Schur complement: GGA⎝ 1 2⎠ 3 ⎛ A−1 0 ⎞ ⎛ F ⎞ SAGG= −()⎜ ⋅1 ⎟⋅⎜ 1 ⎟ = 3 1 2 ⎜ −1 ⎟ ⎜ ⎟ ⎝ 0 A2 ⎠ ⎝ F2 ⎠ −1 −1 18 AGAFGAF=3 − 1 11 − 2 2 2 Direct derivation of Schur complement: AF⎛ 0 ⎞ ⎛ x ⎞ ⎛ b ⎞ A x+ F x= b ⎜ 1 1 ⎟ ⎜ 1 ⎟ ⎜ 1 ⎟ 1 1 1 3 1 ⎜ 0 AF2 2⎟⋅⎜ x2 ⎟ = ⎜b2 ⎟ ⇒ A x2+ 2 F2 3 x = 2 b ⎜ ⎟ ⎜ ⎟ ⎜ ⎟ GGA⎝ 1 2⎠ 3 ⎝ x3 ⎠ ⎝Gb3 ⎠ x1+ 1 G2 x 2 +3 A 3 x = 3 b −1 −1 x1 A= b 1 1 − A 1 1 3 F x ⇒ −1 −1 x2 A= b 2 2 − A 2 2 3 F x −1 −1 −1 −1 GAbGAFx⇒ ( 1 11− 1 1 1)( 3+ GAb2 22 − 22 2 GAFx 3+) 3 3 = 3 Axb −1 −1 −1 −1 A GAF⇒(3 − 1GAFxb 11 2 − 2 2 ) 3= 3 − 11 GAbGAb 1 − 2 2 2 ~ 19 ⇒Sx3 = 3 b Algorithm for solving Ax=b based on Schur complement: 1. Compute S by using inv(A1) and inv(A2) ~ 2. Solve Sx3 =b 3 3. Compute x1 and x2 by using inv(A1) and inv(A2) The explicit computation of S can be avoided by solving the linear system in S iteratively, e.g. Jacobi, pcg, …. Then we need only part of S and in every iteration step we have to compute S * intermediate vector. To achieve fast convergence, a preconditioner (approximation) for S has to be used! Iterative methods and preconditioning will be subject of later chapters.
Details
-
File Typepdf
-
Upload Time-
-
Content LanguagesEnglish
-
Upload UserAnonymous/Not logged-in
-
File Pages47 Page
-
File Size-