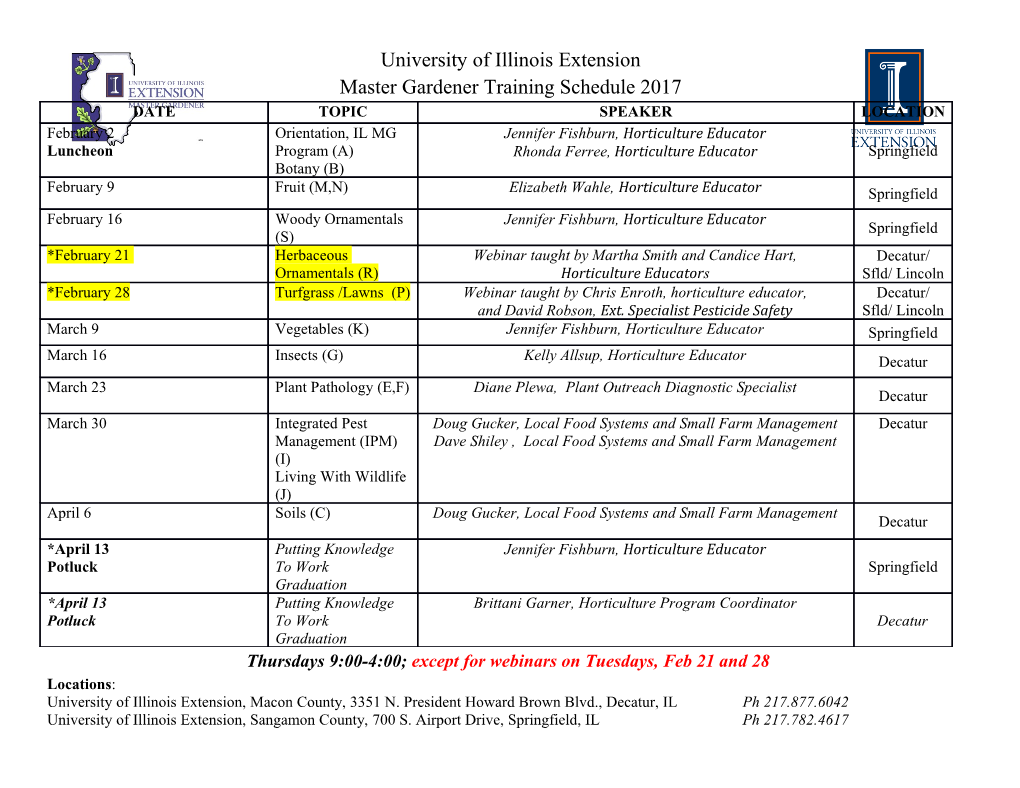
Means • Recall: We model a time series as a collection of random variables: x1; x2; x3;::: , or more generally fxt; t 2 T g. • The mean function is Z 1 µx;t = E(xt) = xft(x)dx 1 where the expectation is for the given t, across all the possible values of xt. Here ft(·) is the pdf of xt. 1 Example: Moving Average • wt is white noise, with E (wt) = 0 for all t • the moving average is 1 v = w + w + w t 3 t−1 t t+1 • so 1 h i µ = E (v ) = E w + E (w ) + E w = 0: v;t t 3 t−1 t t+1 2 Moving Average Model with Mean Function 1 0 v −1 −2 0 100 200 300 400 500 Time 3 Example: Random Walk with Drift • The random walk with drift δ is t X xt = δt + wj j=1 • so t X µx;t = E (xt) = δt + E wj = δt; j=1 a straight line with slope δ. 4 Random Walk Model with Mean Function 80 60 x 40 20 0 0 100 200 300 400 500 Time 5 Example: Signal Plus Noise • The \signal plus noise" model is xt = 2 cos(2πt=50 + 0:6π) + wt • so µx;t = E (xt) = 2 cos(2πt=50 + 0:6π) + E (wt) = 2 cos(2πt=50 + 0:6π); the (cosine wave) signal. 6 Signal-Plus-Noise Model with Mean Function 4 2 x 0 −2 −4 0 100 200 300 400 500 Time 7 Covariances • The autocovariance function is, for all s and t, h i γx(s; t) = E (xs − µx;s) xt − µx;t : • Symmetry: γx(s; t) = γx(t; s). • Smoothness: { if a series is smooth, nearby values will be very similar, hence the autocovariance will be large; { conversely, for a \choppy" series, even nearby values may be nearly uncorrelated. 8 Example: White Noise 2 • If wt is white noise wn(0; σw), then 8 2 <σw; s = t; γw(s; t) = E (wswt) = :0; s 6= t: • definitely choppy! 9 Autocovariances of White Noise gamma t s 10 Example: Moving Average • The moving average is 1 v = w + w + w t 3 t−1 t t+1 and E (vt) = 0, so γv(s; t) = E (vsvt) 1 h i = E w + ws + w w + w + w 9 s−1 s+1 t−1 t t+1 8 2 >(3=9)σ ; s = t > w > 2 <(2=9)σw; s = t ± 1 = 2 >(1=9)σw; s = t ± 2 > :>0; otherwise: 11 Autocovariances of Moving Average gamma t s 12 Example: Random Walk • The random walk with zero drift is t X xt = wj j=1 and E (xt) = 0 • so γx(s; t) = E (xsxt) 0 s t 1 X X = E @ wj wjA j=1 j=1 2 = minfs; tgσw: 13 Autocovariances of Random Walk gamma t s 14 • Notes: { For the first two models, γx(s; t) depends on s and t only through js − tj, but for the random walk γx(s; t) depends on s and t separately. { For the first two models, the variance γx(t; t) is constant, 2 but for the random walk γx(t; t) = tσw increases indefi- nitely as t increases. 15 Correlations • The autocorrelation function (ACF) is γ(s; t) ρ(s; t) = q γ(s; s)γ(t; t) • Measures the linear predictability of xt given only xs. • Like any correlation, −1 ≤ ρ(s; t) ≤ 1. 16 Across Series • For a pair of time series xt and yt, the cross covariance function is h i γx;y(s; t) = E (xs − µx;s) yt − µy;t : • The cross correlation function (CCF) is γx;y(s; t) ρx;y(s; t) = q : γx(s; s)γy(t; t) 17 Stationary Time Series • Basic idea: the statistical properties of the observations do not change over time. • Two specific forms: strong (or strict) stationarity and weak stationarity. • A time series xt is strongly stationary if the joint distribution of every collection of values is the same as fxt1; xt2; : : : ; xtkg that of the time-shifted values fx ; x ; : : : ; x g, for t1+h t2+h tk+h every dimension k and shift h. • Strong stationarity is hard to verify. 18 If fxtg is strongly stationary, then for instance: • k = 1: the distribution of xt is the same as that of xt+h, for any h; { in particular, if we take h = −t, the distribution of xt is the same as that of x0; { that is, every xt has the same distribution; 19 • k = 2: the joint (bivariate) distribution of (xs; xt) is the same as that of (xs+h; xt+h), for any h; { in particular, if we take h = −t, the joint distribution of (xs; xt) is the same as that of (xs−t; x0); { that is, the joint distribution of (xs; xt) depends on s and t only through s − t; • and so on... 20 • A time series xt is weakly stationary if: { the mean function µt is constant; that is, every xt has the same mean; { the autocovariance function γ(s; t) depends on s and t only through their difference js − tj. • Weak stationarity depends only on the first and second mo- ment functions, so is also called second-order stationarity. • Strongly stationary (plus finite variance) ) weakly stationary. • Weakly stationary 6) strongly stationary (unless some other property implies it, like normality of all joint distributions). 21 Simplifications • If xt is weakly stationary, cov xt+h; xt depends on h but not on t, so we write the autocovariances as γ(h) = cov xt+h; xt • Similarly corr xt+h; xt depends only on h, and can be written γ(t + h; t) γ(h) ρ(h) = q = : γ(t + h; t + h)γ(t; t) γ(0) 22 Examples • White noise is weakly stationary. • A moving average is weakly stationary. • A random walk is not weakly stationary. 23.
Details
-
File Typepdf
-
Upload Time-
-
Content LanguagesEnglish
-
Upload UserAnonymous/Not logged-in
-
File Pages23 Page
-
File Size-