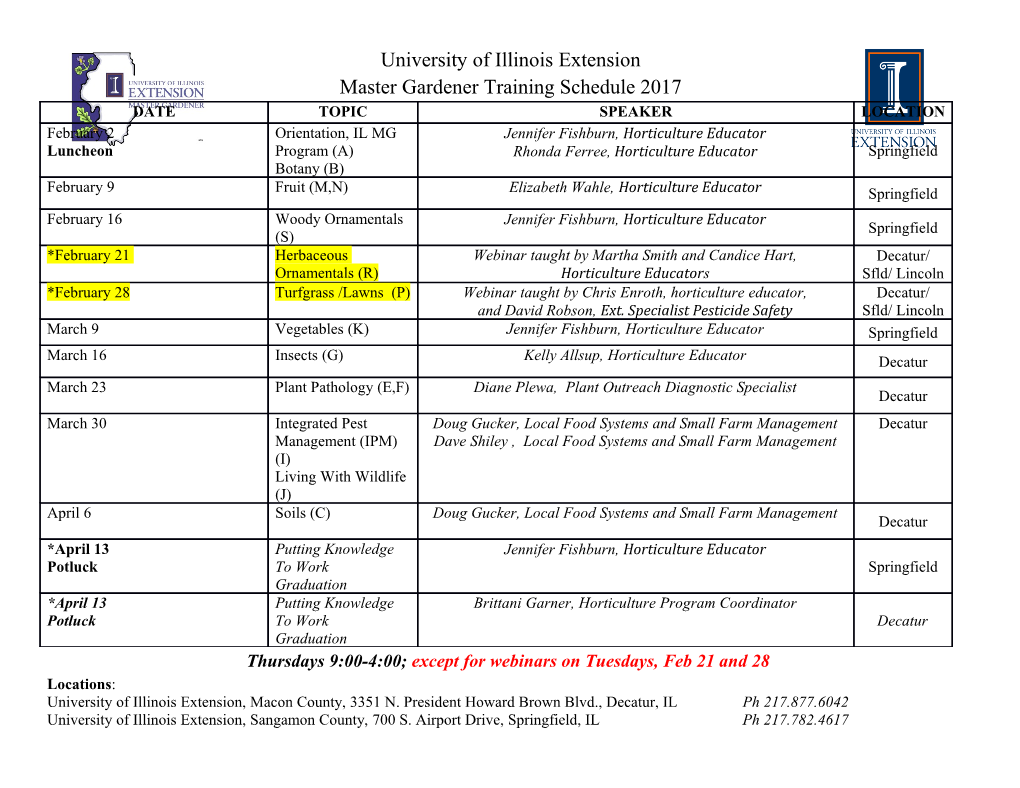
Approximation of the expected value of the harmonic mean and some applications Calyampudi Radhakrishna Raoa,b,1, Xiaoping Shic, and Yuehua Wuc aDepartment of Biostatistics, University at Buffalo, The State University of New York, Buffalo, NY 14221-3000; bCRRAO Advanced Institute of Mathematics, Statistics And Computer Science, Hyderabad-500046, India; and cDepartment of Mathematics and Statistics, York University, Toronto, ON, Canada M3J 1P3 Contributed by Calyampudi R. Rao, July 1, 2014 (sent for review June 5, 2014) Although the harmonic mean (HM) is mentioned in textbooks asymptotic expansion of EðHnÞ or the general version EðHnðwÞÞ along with the arithmetic mean (AM) and the geometric mean (GM) under more general assumptions on distributions of Zi s. We as three possible ways of summarizing the information in a set of show that under mild assumptions, observations, its appropriateness in some statistical applications is ( ) notmentionedintextbooks.Duringthelast10yanumberof 1 pffiffiffiffiffiffiffiffiffiffiffiffiffic1 papers were published giving some statistical applications where EðHnÞ ∼ 1 + ; [5] logðnÞ HM is appropriate and provides a better performance than AM. In logðnÞ the present paper some additional applications of HM are consid- ered. The key result is to find a good approximation to E(Hn), the where the constant c1 will be given. In addition, we use the 5 expectation of the harmonic mean of n observations from a proba- approach for obtaining [ ] to the case that the first moment of = bility distribution. In this paper a second-order approximation to 1 Zi is finite, motivated by evaluation of the marginal likelihood E(Hn) is derived and applied to a number of problems. in ref. 11. Approximations harmonic mean | second-order approximation | arithmetic mean | image denoising | marginal likelihood We derive the asymptotic approximation of EðHnÞ when the first moment of 1=Zi is not finite. Let fZig be a sequence of inde- pendent and identically distributed (i.i.d.) random variables with he harmonic mean H of n observations Z ; ...; Z drawn n 1 n possible infinite first moment. Suppose that there exist constants Tfrom a population is defined by An and Bn, such that the distribution FnðxÞ of n H = P : [1] n n 1 = + = + ⋯ + = i=1 Z 1 Z1 1 Z2 1 Zn i Xn = − An [6] Bn There have been a number of applications of the harmonic mean in recent papers. A more general version of Hn with weights converges weakly to a nondegenerate distribution FðxÞ such that w ; ...; w is 1 n P n d1 + oð1Þ = wi FðxÞ = α as x → −∞; [7] H w = Pi 1 : [2] nð Þ n wi jxj = i 1 Zi + w = ; ...; T d2 oð1Þ where ðw1 wnÞ . The harmonic mean Hn is used to provide 1 − FðxÞ = α as x → ∞; [8] the average rate in physics and to measure the price ratio in finance jxj as well as the program execution rate in computer engineering. α ≤ α < ; ≥ Some statistical applications of the harmonic mean are given in refs. where , d1, and d2 are constants with 0 2, d1 d2 0, and + > 1-4, among others. HnðwÞ has been used in evaluation of the port- d1 d2 0, respectively. The set of all distributions converging to folio price-to-earnings ratio value (ref. 5, p. 339) and the signal-to- FðxÞ is called the domain of attraction of FðxÞ. It is known that only interference-and-noise ratio (6) among others. The asymptotic stable laws with index αð0 ≤ α < 2Þ have the nonempty domains of properties of Hn including the asymptotic expansion of EðHnÞ are attraction as shown by refs. 12 (chap. 7) and 13 (chap. 2). investigated in refs. 7 and 8 by either assuming that some moments of 1=Zi are finite or that Zi s follow the Poisson distribution. It is Significance noted that recent papers (9, 10) enable one to use saddle-point STATISTICS approximation to give the asymptotic expansion of EðHnÞ to any The harmonic mean (HM) filter is better at removing positive given order of 1=n for some constants c0; c1; c2; ..., i.e., outliers than the arithmetic mean (AM) filter. There are espe- c c cially difficult issues when an accurate evaluation of expected EðH Þ = c + 1 + 2 + ⋯: [3] n 0 n n2 HM is needed such as, for example, in image denoising and marginal likelihood evaluation. A major challenge is to develop However, such methods are not applicable for obtaining the a higher-order approximation of the expected HM when the asymptotic expansion of Hn when the first moment of 1=Zi is central limit theorem is not applicable. A two-term approxi- infinite. In ref. 3, Zi s are assumed to follow a uniform distribu- mation of the expected HM is derived in this paper. This ap- tion in the interval ð0; 1Þ, i.e., Uð0; 1Þ, motivated by learning proximation enables us to develop a new filtering procedure to theory. Using the property that the inverse of Hn converges to denoise the noisy image with an improved performance, and the stable law, ref. 3 showed that construct a truncated HM estimator with a faster convergence rate in marginal likelihood evaluation. 1 EðHnÞ ∼ ; [4] logðnÞ Author contributions: C.R.R. designed research; C.R.R., X.S., and Y.W. performed research; X.S. and Y.W. analyzed data; and C.R.R., X.S., and Y.W. wrote the paper. where the symbol “∼” means asymptotic equivalence as n → ∞. The authors declare no conflict of interest. Our interest in this paper is to determine the second term in the 1To whom correspondence should be addressed. Email: [email protected]. www.pnas.org/cgi/doi/10.1073/pnas.1412216111 PNAS | November 4, 2014 | vol. 111 | no. 44 | 15681–15686 Downloaded by guest on September 28, 2021 Assume that there is a positive constant d which does not 3 1 log½logðnÞ depend on n such that logðnÞHn − 1 + pffiffiffiffiffiffiffiffiffiffiffiffiffi = β : logðnÞ logðnÞ Xn + An ≥ d3 > 0: [9] Thus, we obtain the following approximation: We further assume a uniform rate of convergence of FnðxÞ to F x such that ∼ ℓ−2 − ℓ−3 + : ℓ−4: [16] ð Þ EðHnÞ n n 0 5673 logðlogðnÞÞ n À Á −β supjFnðxÞ − FðxÞj = o n ; [10] As in ref. 3, suppose that Zi s follow a uniform distribution x Uð0; 1Þ. The distribution of Yi = 1=Zi is easily seen to be given by for some positive constant β < 1. Our assumptions are mild. Ref. 14 showed that sup jF ðxÞ − FðxÞj has the rate of ofn−1 log ðnÞg x n PðY ≤ tÞ = ð1 − 1=tÞIðt ≥ 1Þ; under some assumptions. We have the following asymptotic approximation of EðHnÞ: · Theorem 1. 7 – 10 where Ið Þ is an indicator function. It is well known that the Assume that conditions [ ] [ ] are satisfied and r < ∞ < = = α = = = mean of Yi is infinite but EYi for r 1. By considering the An logðnÞ, Bn n, 1, d1 0, and d2 1. Then we have the limit stable distribution with index α = 1 of the distribution of following first approximation: Xn for An = logðnÞ and Bn = n, ref. 3 obtained the result [4], À Á which is = + −1 = ℓ−2 − ℓ−3 + ℓ−3 ; [11] EðHnÞ EðXn log nÞ n n o n pffiffiffiffiffiffiffiffiffiffiffiffiffi Ef½logðnÞHng ∼ 1: [17] where ℓn = logðnÞ. The proof is given in Appendix: Proof of Theorem 1. Because According to our Theorem 1 and the approximation [16], n−β in [10] is smaller than the remaining terms in [11], the coefficients of both ℓ−2 and ℓ−3 are independent of β in [11]. 1 n n Ef½logðnÞHng ∼ 1 − pffiffiffiffiffiffiffiffiffiffiffiffiffi; [18] Remark 1: For an extension of Theorem 1 to the weighted logðnÞ harmonic mean in [2], we consider the following partial sum: : = + = + ⋯ + = ∼ − pffiffiffiffiffiffiffiffiffiffiffiffiffi1 + 0 5673 log½logðnÞ: [19] w = w1 Z1 w2 Z2 wn Zn − ; [12] Ef½logðnÞHng 1 Xnð Þ An logðnÞ logðnÞ Wn P α =α 17 – 19 where W = ð n jw j Þ1 . Motivated by ref. 15, we may assume Fig. 1 displays the approximations given in [ ] [ ]compared n i = 1 i with the sample mean of 1,000,000 replications of n independent the following two conditions on the weights wi s: observations from the uniform distribution Uð0; 1Þ that serves as a ; ; max jwij = oðWnÞ; [13] proxy for the exact value of EðHnÞ.Heren takesvalues1015 1≤i≤n 20; ..., and 200. From Fig. 1, it can be seen that the approxi- mation [18] is better than the approximation [17]. Although the and the characteristic function of 1=Z in [6] satisfies that i approximation [19] is purely empirical, this empirical exercise α À αÁ 1 − cjtj + o jtj as t → 0: [14] basically achieves the desired result as shown in Fig. 1; it clearly gives much better approximation of Ef½logðnÞHng than its other Under the conditions [13]and[14], ref. 15 showed that the two counterparts. α > = 1=α distribution of XnðwÞ converges to a stable distribution with We now consider the case that 1. In this case, Bn n and α = = 1−1=α characteristic function expð−cjtj Þ. For example, if Zi s follow An Eð1 Z1Þn . Thus, we have uniform distribution Uð0; 1Þ,thecondition[14] is satisfied when A = log n and α = 1. Following the proof of Theorem 1,itcanbe 1−1=α n = n : [20] shown that Hn 1−1=α Xn + n Eð1=Z1Þ À Á w = ℓ−2 − ℓ−3 + ℓ−3 ; [15] EfHnð Þg n n o n In light of the proof of Theorem 1, we have the following asymp- pffiffiffiffiffiffiffiffiffiffiffiffiffi totic approximation of EðH Þ: ℓ = 2 n where n logðnÞ.
Details
-
File Typepdf
-
Upload Time-
-
Content LanguagesEnglish
-
Upload UserAnonymous/Not logged-in
-
File Pages6 Page
-
File Size-