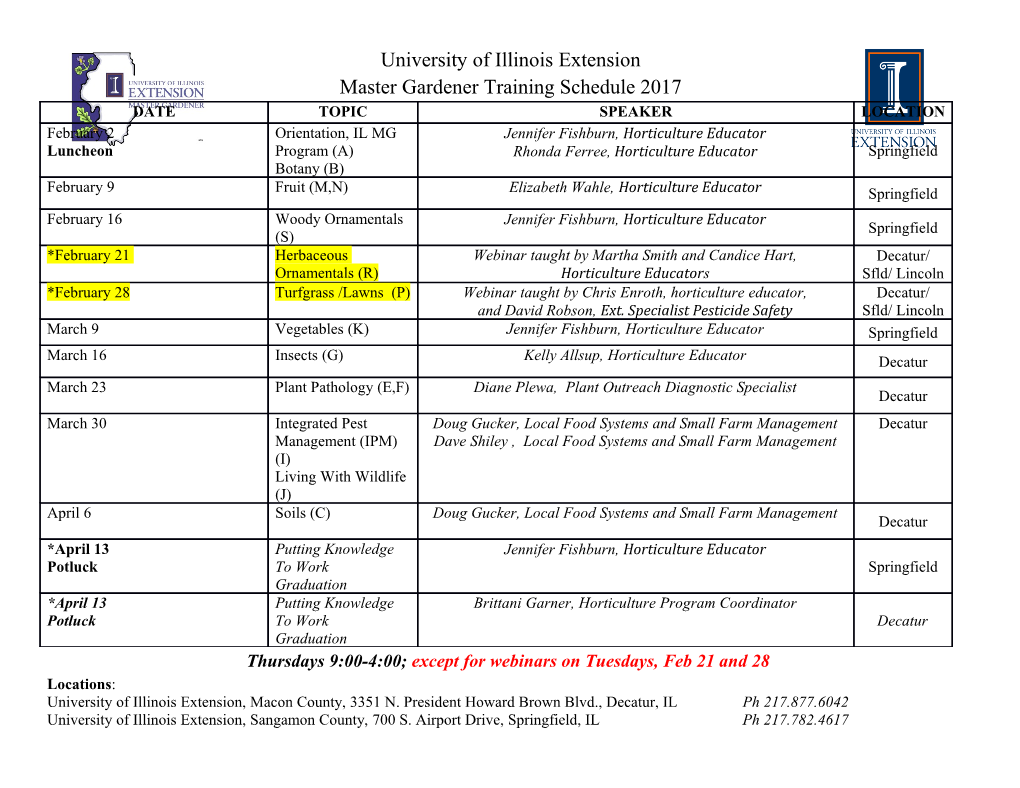
xgboost Release 0.80 xgboost developers Sep 28, 2018 Contents 1 Contents 3 1.1 Installation Guide............................................3 1.2 Get Started with XGBoost........................................ 10 1.3 XGBoost Tutorials............................................ 12 1.4 Frequently Asked Questions....................................... 30 1.5 XGBoost GPU Support......................................... 32 1.6 XGBoost Parameters........................................... 34 1.7 XGBoost Python Package........................................ 42 1.8 XGBoost R Package........................................... 64 1.9 XGBoost JVM Package......................................... 81 1.10 XGBoost.jl................................................ 96 1.11 XGBoost Command Line version.................................... 96 1.12 Contribute to XGBoost.......................................... 96 Python Module Index 101 i ii xgboost, Release 0.80 XGBoost is an optimized distributed gradient boosting library designed to be highly efficient, flexible and portable. It implements machine learning algorithms under the Gradient Boosting framework. XGBoost provides a parallel tree boosting (also known as GBDT, GBM) that solve many data science problems in a fast and accurate way. The same code runs on major distributed environment (Hadoop, SGE, MPI) and can solve problems beyond billions of examples. Contents 1 xgboost, Release 0.80 2 Contents CHAPTER 1 Contents 1.1 Installation Guide Note: Pre-built binary wheel for Python If you are planning to use Python, consider installing XGBoost from a pre-built binary wheel, available from Python Package Index (PyPI). You may download and install it by running # Ensure that you are downloading one of the following: # * xgboost-{version}-py2.py3-none-manylinux1_x86_64.whl # * xgboost-{version}-py2.py3-none-win_amd64.whl pip3 install xgboost • The binary wheel will support GPU algorithms (gpu_exact, gpu_hist) on machines with NVIDIA GPUs. How- ever, it will not support multi-GPU training; only single GPU will be used. To enable multi-GPU training, download and install the binary wheel from this page. • Currently, we provide binary wheels for 64-bit Linux and Windows. 1.1.1 Building XGBoost from source This page gives instructions on how to build and install XGBoost from scratch on various systems. It consists of two steps: 1. First build the shared library from the C++ codes (libxgboost.so for Linux/OSX and xgboost.dll for Windows). (For R-package installation, please directly refer to R Package Installation.) 2. Then install the language packages (e.g. Python Package). Note: Use of Git submodules 3 xgboost, Release 0.80 XGBoost uses Git submodules to manage dependencies. So when you clone the repo, remember to specify --recursive option: git clone --recursive https://github.com/dmlc/xgboost For windows users who use github tools, you can open the git shell and type the following command: git submodule init git submodule update Please refer to Trouble Shooting section first if you have any problem during installation. If the instructions do not work for you, please feel free to ask questions at the user forum. Contents • Building the Shared Library – Building on Ubuntu/Debian – Building on OSX – Building on Windows – Building with GPU support – Customized Building • Python Package Installation • R Package Installation • Trouble Shooting 1.1.2 Building the Shared Library Our goal is to build the shared library: • On Linux/OSX the target library is libxgboost.so • On Windows the target library is xgboost.dll The minimal building requirement is • A recent C++ compiler supporting C++11 (g++-4.8 or higher) We can edit make/config.mk to change the compile options, and then build by make. If everything goes well, we can go to the specific language installation section. Building on Ubuntu/Debian On Ubuntu, one builds XGBoost by running git clone --recursive https://github.com/dmlc/xgboost cd xgboost; make -j4 4 Chapter 1. Contents xgboost, Release 0.80 Building on OSX Install with pip: simple method First, make sure you obtained gcc-5 (newer version does not work with this method yet). Note: installation of gcc can take a while (~ 30 minutes). brew install gcc@5 Then install XGBoost with pip: pip3 install xgboost You might need to run the command with sudo if you run into permission errors. Build from the source code - advanced method First, obtain gcc-7 with homebrew (https://brew.sh/) if you want multi-threaded version. Clang is okay if multi- threading is not required. Note: installation of gcc can take a while (~ 30 minutes). brew install gcc@7 Now, clone the repository: git clone --recursive https://github.com/dmlc/xgboost cd xgboost; cp make/config.mk ./config.mk Open config.mk and uncomment these two lines: exportCC= gcc export CXX= g++ and replace these two lines as follows: (specify the GCC version) exportCC= gcc-7 export CXX= g++-7 Now, you may build XGBoost using the following command: make -j4 You may now continue to Python Package Installation. Building on Windows You need to first clone the XGBoost repo with --recursive option, to clone the submodules. We recommend you use Git for Windows, as it comes with a standard Bash shell. This will highly ease the installation process. git submodule init git submodule update XGBoost support compilation with Microsoft Visual Studio and MinGW. 1.1. Installation Guide 5 xgboost, Release 0.80 Compile XGBoost using MinGW After installing Git for Windows, you should have a shortcut named Git Bash. You should run all subsequent steps in Git Bash. In MinGW, make command comes with the name mingw32-make. You can add the following line into the . bashrc file: alias make='mingw32-make' (On 64-bit Windows, you should get MinGW64 instead.) Make sure that the path to MinGW is in the system PATH. To build with MinGW, type: cp make/mingw64.mk config.mk; make -j4 Compile XGBoost with Microsoft Visual Studio To build with Visual Studio, we will need CMake. Make sure to install a recent version of CMake. Then run the following from the root of the XGBoost directory: mkdir build cd build cmake .. -G"Visual Studio 12 2013 Win64" This specifies an out of source build using the MSVC 12 64 bit generator. Open the .sln file in the build directory and build with Visual Studio. To use the Python module you can copy xgboost.dll into python-package/ xgboost. After the build process successfully ends, you will find a xgboost.dll library file inside ./lib/ folder, copy this file to the the API package folder like python-package/xgboost if you are using Python API. Unofficial windows binaries and instructions on how to use them are hosted on Guido Tapia’s blog. Building with GPU support XGBoost can be built with GPU support for both Linux and Windows using CMake. GPU support works with the Python package as well as the CLI version. See Installing R package with GPU support for special instructions for R. An up-to-date version of the CUDA toolkit is required. From the command line on Linux starting from the XGBoost directory: mkdir build cd build cmake .. -DUSE_CUDA=ON make -j Note: Enabling multi-GPU training By default, multi-GPU training is disabled and only a single GPU will be used. To enable multi-GPU training, set the option USE_NCCL=ON. Multi-GPU training depends on NCCL2, available at this link. Since NCCL2 is only available for Linux machines, multi-GPU training is available only for Linux. 6 Chapter 1. Contents xgboost, Release 0.80 mkdir build cd build cmake .. -DUSE_CUDA=ON -DUSE_NCCL=ON make -j On Windows, see what options for generators you have for CMake, and choose one with [arch] replaced with Win64: cmake -help Then run CMake as follows: mkdir build cd build cmake .. -G"Visual Studio 14 2015 Win64" -DUSE_CUDA=ON Note: Visual Studio 2017 Win64 Generator may not work Choosing the Visual Studio 2017 generator may cause compilation failure. When it happens, specify the 2015 compiler by adding the -T option: make .. -G"Visual Studio 15 2017 Win64" -T v140,cuda=8.0 -DR_LIB=ON -DUSE_CUDA=ON To speed up compilation, the compute version specific to your GPU could be passed to cmake as, e.g., -DGPU_COMPUTE_VER=50. The above cmake configuration run will create an xgboost.sln solution file in the build directory. Build this solution in release mode as a x64 build, either from Visual studio or from command line: cmake --build . --target xgboost --config Release To speed up compilation, run multiple jobs in parallel by appending option -- /MP. Customized Building The configuration file config.mk modifies several compilation flags: - Whether to enable support for various dis- tributed filesystems such as HDFS and Amazon S3 - Which compiler to use - And some more To customize, first copy make/config.mk to the project root and then modify the copy. Python Package Installation The python package is located at python-package/. There are several ways to install the package: 1. Install system-wide, which requires root permission: cd python-package; sudo python setup.py install You will however need Python distutils module for this to work. It is often part of the core python package or it can be installed using your package manager, e.g. in Debian use sudo apt-get install python-setuptools 1.1. Installation Guide 7 xgboost, Release 0.80 Note: Re-compiling XGBoost If you recompiled XGBoost, then you need to reinstall it again to make the new library take effect. 2. Only set the environment variable PYTHONPATH to tell python where to find the library. For example, assume we cloned xgboost on the home directory ~. then we can added the following line in ~/.bashrc. This option is recommended for developers who change the code frequently. The changes will be immediately reflected once you pulled the code and rebuild the project (no need to call setup again) export PYTHONPATH=~/xgboost/python-package 3. Install only for the current user. cd python-package; python setup.py develop --user 4.
Details
-
File Typepdf
-
Upload Time-
-
Content LanguagesEnglish
-
Upload UserAnonymous/Not logged-in
-
File Pages108 Page
-
File Size-