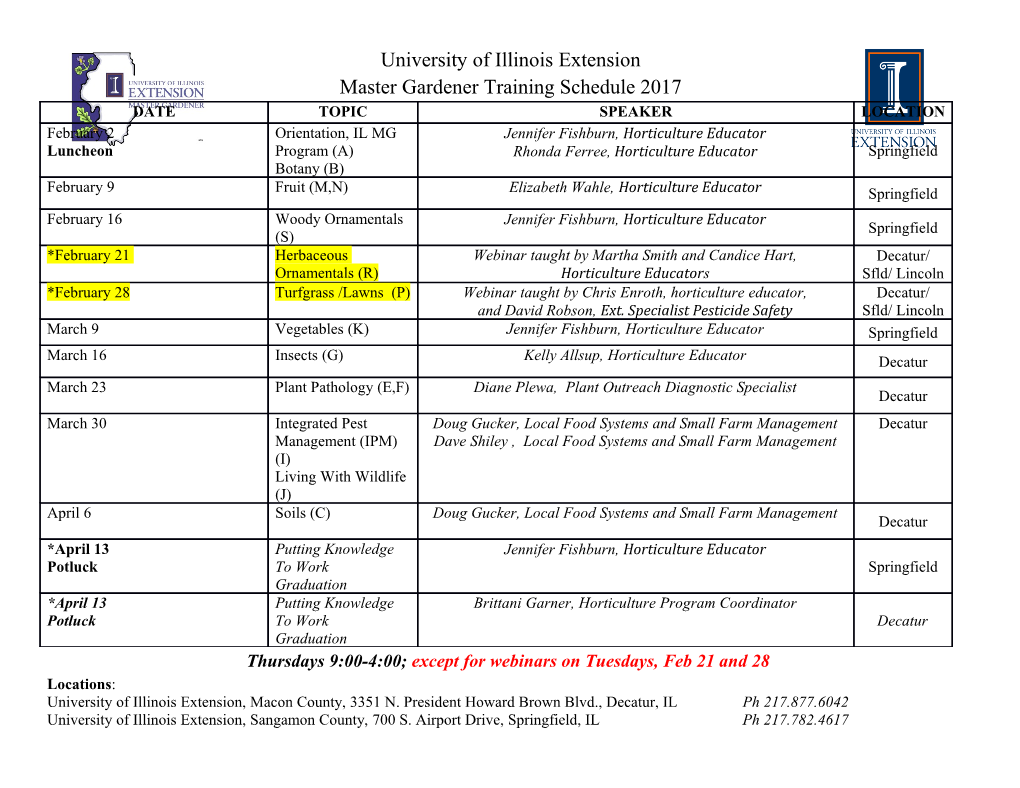
Fast Image Registration Using Pyramid Edge Images Kee-Baek Kim, Jong-Su Kim, Sangkeun Lee, and Jong-Soo Choi* The Graduate School of Advanced Imaging Science, Multimedia and Film, Chung-Ang University, Seoul, Korea(R.O.K) *Corresponding author’s E-mail address: [email protected] Abstract: Image registration has been widely used in many image processing-related works. However, the exiting researches have some problems: first, it is difficult to find the accurate information such as translation, rotation, and scaling factors between images; and it requires high computational cost. To resolve these problems, this work proposes a Fourier-based image registration using pyramid edge images and a simple line fitting. Main advantages of the proposed approach are that it can compute the accurate information at sub-pixel precision and can be carried out fast for image registration. Experimental results show that the proposed scheme is more efficient than other baseline algorithms particularly for the large images such as aerial images. Therefore, the proposed algorithm can be used as a useful tool for image registration that requires high efficiency in many fields including GIS, MRI, CT, image mosaicing, and weather forecasting. Keywords: Image registration; FFT; Pyramid image; Aerial image; Canny operation; Image mosaicing. registration information as image size increases [3]. 1. Introduction To solve these problems, this work employs a pyramid-based image decomposition scheme. Image registration is a basic task in image Specifically, first, we use the Gaussian filter to processing to combine two or more images that are remove the noise because it causes many undesired partially overlapped. Image registration has been problems in image registration works. Next, Canny used in various fields such as geographic edge operator is applied on a given input image to information system (GIS), aerial, satellite, weather, reduce the computational cost dealt with. Next, the underwater images, and etc [3-5]. Many pyramid-based image decomposition is conducted to image-related works can be separated into three obtain the sub-pixel accuracy information and to main classes which are the correlation-based, improve the computation speed by using smaller feature-based, and FFT-based methods. However, image size if necessary. Then, we estimate the they cannot find the sub-pixel accuracy information registration information integrated with the for image registration when the full-sized images computed values from each pyramid level. It is need to be registered. In particular, FFT-based noted that this work estimates the fundamental approach has been widely used since it is fast and geometric changes such as image rotation, scaling, more robust than others in image brightness changes and translation for robust and effective registration. even though it may have some problems when it The experiment shows that the proposed handles noisy images in the feature detection stage. algorithm saves computational cost with maintaining However, its computation cost increases the image quality, and it is particularly efficient in significantly, and it cannot find the accurate aerial images that contain relatively less geometric warping problem. This paper is organized as follows. In Section 2, the existing works are reviewed, and we present a proposed scheme for image registration in Section 3. Section 4 shows the experimental results and compares with other baseline algorithms. In Section 5 concludes this work with some discussions. 2. Background (a) (b) 2.1. Canny Edge Detection Canny edge detector is defined by three concepts which are error rate, localization, and response [1-2]. Basic idea is to detect the zero-crossings of the second directional derivative of the smoothed image in the direction of the gradient where the gradient magnitude of the smoothed image is greater than some threshold depending on image statistics. (c) (d) Specifically, it first employs a Gaussian function for Fig. 1. Filtering results of Canny edge detector : (a) An the image smoothing and uses the first derivative on Origin image, (b) Gaussian filtering with σ=0.5, (c) it, respectively, as Non-maximal suppression, and (d) Hystersis Thresholding with t =128 and t =64. x2+ y 2 h l − 1 2σ 2 G(,) x y= 2 e 2πσ 2.2. Fourier Based Registration x2+ y 2 x2+ y 2 − − ∂G(,) x y 2 ∂G(,) x y 2 = αxe 2σ , = αye 2σ . (1) In Fourier-based image registration, the first step ∂x ∂y is to find the translation information, and then the ⎛ 1 ⎞ rotation and scaling Information are computed [4-5]. ⎜∴α = ⎟ ⎝ 2πσ 2 ⎠ Next, original image and this function are convolved 2.2.1. Translation in both the vertical and horizontal directions. This A displacement (x , y ) between two consecutive operation is described in the following equation [1-2] 0 0 images is formulated by as, f(,)(,) x y= f x − x y − y . (3) I'( x , y )= g ( k , l ) * I ( x , y ) 2 1 0 0 Using a Fourier shifting property, we get N N (2) −j(ω1 x 0 + ω 2 y 0 ) = ∑∑g(,)(,) k l I x− k y − l F2(,)(,)ω 1 ω 2= F 1 ω 1 ω 2 e . (4) k=−− N l= N Where, I is an original image, and g is a convolution kernel. The non-maximal suppression step finds the local maxima in the direction of gradient, and suppresses all others for minimizing false edges. Then, a hysteresis threshold is applied. The hysteresis threshold uses two level threshold values (a) (b) (c) which are th and tl (th > tl) for a high and low levels, Fig.2. Translation : (a) Input image1, (b) Input image2, respectively [2]. and (c) Cross-power spectrum The performance of Canny edge detector depends on Next, we can find the cross-power-spectrum the parameters σ, th, and tl. If σ is bigger, we can between images (see Fig. 2) [4-5] as make a stronger Gaussian filter. Fig. 1 shows the * FF2(,)(,)ω 1 ω 2 1 ω 1 ω 2 −j(ω1 x 0 + ω 2 y 0 ) procedures of Canny edge detection. * = e |FF2 (ω 1 , ω 2 ) 1 ( ω 1 , ω 2 ) | . (5) F −1 =δ (x − x0 , y − y0 ) From this, we can compute the translation information easily. 2.2.2. Rotation Similarly, to get the rotation information, we first formulate the rotation relation in the spatial domain [4-5] as f(,) x y 2 . (6) = f1 ( x cosθ0 + ysin θ0 , − x cos θ0 + y sin θ0 ) Next, we convert it to the Fourier space as F2(,)ω 1 ω 2 Fig.4. Flow chart of the proposed Algorithm . (7) = f1(ω 1 cos θ0+ ω 2 sin θ0 , − ω 1 cos θ0 + ω 2 sin θ0 ) After converting the above equation to the polar 3.1. Feature detection coordinates [4-5] as In this work, to reduce the computation cost, a M (,)ρ θ− θ , (8) 2 0 pyramid image decomposition scheme is used. Let we can get the rotation information θ0 (see Fig. 3 for the corresponding pyramid pairs and input images n n the procedure). are g1 , g2 and I1, I2 respectively. Then, the pyramid decomposition [2] is described by 2.2.3. Scale gn ( x , y )= Pn ( I ( x , y )) 1 1 . (11) n n , n=1,2,..., n When an image is scaled by a to another image, g2 ( x , y )= P ( I2 ( x , y )) the relation in Fourier transform is expressed by Where, n and P means pyramid levels and a pyramid 1 function. It is noted that the pyramid levels are F (,)ω ω = F(/,/)ω a ω a . (9) 2 1 2 a 2 1 1 2 determined by the degree of feature matching. In Taking the logarithm, this can be rewritten by [4-5] other words, an image is inputted to the next level of F (ω , ω )= F (logω − loga ,logω − loga ) . (10) pyramid until registration information is not found 2 1 2 1 1 2 in feature matching. We can get the scale factor a from this. Note that we ignore a constant 1/a2 for simplicity. a) Input image 1 and its pyramid edge pairs (a) (b) Fig.3. Rotation and scaling : (a) Input image 1 and Log-Polar, (b) Input image 2 and Log-Polar. 3. Proposed Algorithm There are three main steps in image registration b) Input image 2 and its pyramid edge pairs procedures. They are a feature detection, a feature Fig.5. Pyramid edge pairs matching, and the panorama image generation. In particular, the feature detection in registration To reduce more computation cost, the edges in related works is severely affected by brightness pyramid images are used since pixel-by-pixel images between two given images. To solve this processing is generally more complicated. Moreover, problem and to reduce the computation complexity, when edge images are used in image registration, the we use pyramid edge images in feature detection. noise from brightness differences is reduced [3-5]. Moreover, to accelerate the processing speed, we For this purpose, we use a Canny operator. Canny collect the registration information from FFT-based operator has many merits. For example, this can approach. Fig. 4 shows the whole procedures. reduce the noise by a Gaussian function and it gives n n the ideal edges. Let pyramid edge images be c1 , c2 and the first derivative of a Gaussian function be ’n h(k,l). Then, the corresponding pyramid edge images image 2 C2 by Fourier shift property [4-5] as [2] can be defined by 'n n * C2 (,)(,)ω1 ω 2C 1 ω 1 ω 2 −j(ϖ x + ϖ y ) n n 1 0 2 0 'n n = e (16) c1 (,)(,)*(,) x y= h k l g1 x y .
Details
-
File Typepdf
-
Upload Time-
-
Content LanguagesEnglish
-
Upload UserAnonymous/Not logged-in
-
File Pages8 Page
-
File Size-