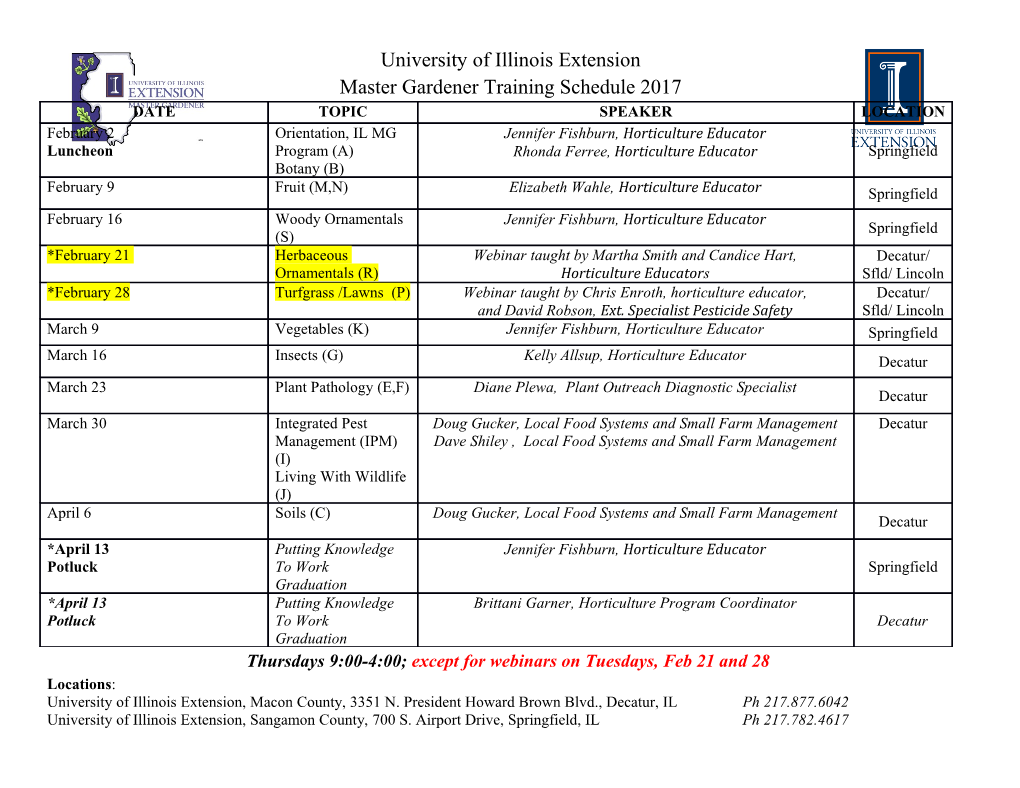
Chapter 6 Direct Methods for Solving Linear Systems Per-Olof Persson [email protected] Department of Mathematics University of California, Berkeley Math 128A Numerical Analysis Direct Methods for Linear Systems Consider solving a linear system of the form: 퐸1 ∶ 푎11푥1 + 푎12푥2 + ⋯ + 푎1푛푥푛 = 푏1, 퐸2 ∶ 푎21푥1 + 푎22푥2 + ⋯ + 푎2푛푥푛 = 푏2, ⋮ 퐸푛 ∶ 푎푛1푥1 + 푎푛2푥2 + ⋯ + 푎푛푛푥푛 = 푏푛, for 푥1, … , 푥푛. Direct methods give an answer in a fixed number of steps, subject only to round-off errors. We use three row operations to simplify the linear system: 1. Multiply Eq. 퐸푖 by 휆 ≠ 0: (휆퐸푖) → (퐸푖) 2. Multiply Eq. 퐸푗 by 휆 and add to Eq. 퐸푖: (퐸푖 + 휆퐸푗) → (퐸푖) 3. Exchange Eq. 퐸푖 and Eq. 퐸푗: (퐸푖) ↔ (퐸푗) Gaussian Elimination Gaussian Elimination with Backward Substitution Reduce a linear system to triangular form by introducing zeros using the row operations (퐸푖 + 휆퐸푗) → (퐸푖) Solve the triangular form using backward-substitution Row Exchanges If a pivot element on the diagonal is zero, the reduction to triangular form fails Find a nonzero element below the diagonal and exchange the two rows Definition An 푛 × 푚 matrix is a rectangular array of elements with 푛 rows and 푚 columns in which both value and position of an element is important Operation Counts Count the number of arithmetic operations performed Use the formulas 푚 푚(푚 + 1) 푚 푚(푚 + 1)(2푚 + 1) ∑ 푗 = , ∑ 푗2 = 푗=1 2 푗=1 6 Reduction to Triangular Form Multiplications/divisions: 푛−1 2푛3 + 3푛2 − 5푛 ∑(푛 − 푖)(푛 − 푖 + 2) = ⋯ = 푖=1 6 Additions/subtractions: 푛−1 푛3 − 푛 ∑(푛 − 푖)(푛 − 푖 + 1) = ⋯ = 푖=1 3 Operation Counts Backward Substitution Multiplications/divisions: 푛−1 푛2 + 푛 1 + ∑((푛 − 푖) + 1) = 푖=1 2 Additions/subtractions: 푛−1 푛2 − 푛 ∑((푛 − 푖 − 1) + 1) = 푖=1 2 Operation Counts Gaussian Elimination Total Operation Count Multiplications/divisions: 푛3 푛 + 푛2 − 3 3 Additions/subtractions: 푛3 푛2 5푛 + − 3 2 6 Partial Pivoting (푘) In Gaussian elimination, if a pivot element 푎푘푘 is small (푘) compared to an element 푎푗푘 below, the multiplier 푎(푘) 푚 = 푗푘 푗푘 (푘) 푎푘푘 will be large, resulting in round-off errors Partial pivoting finds the smallest 푝 ≥ 푘 such that (푘) (푘) |푎푝푘 | = max |푎푖푘 | 푘≤푖≤푛 and interchanges the rows (퐸푘) ↔ (퐸푝) Scaled Partial Pivoting If there are large variations in magnitude of the elements within a row, scaled partial pivoting can be used Define a scale factor 푠푖 for each row 푠푖 = max |푎푖푗| 1≤푗≤푛 At step 푖, find 푝 such that |푎푝푖| |푎 | = max 푘푖 푠푝 푖≤푘≤푛 푠푘 and interchange the rows (퐸푖) ↔ (퐸푝) Linear Algebra Definition Two matrices 퐴 and 퐵 are equal if they have the same number of rows and columns 푛 × 푚 and if 푎푖푗 = 푏푖푗. Definition If 퐴 and 퐵 are 푛 × 푚 matrices, the sum 퐴 + 퐵 is the 푛 × 푚 matrix with entries 푎푖푗 + 푏푖푗. Definition If 퐴 is 푛 × 푚 and 휆 a real number, the scalar multiplication 휆퐴 is the 푛 × 푚 matrix with entries 휆푎푖푗. Properties Theorem Let 퐴, 퐵, 퐶 be 푛 × 푚 matrices, 휆, 휇 real numbers. (a) 퐴 + 퐵 = 퐵 + 퐴 (b) (퐴 + 퐵) + 퐶 = 퐴 + (퐵 + 퐶) (c) 퐴 + 0 = 0 + 퐴 = 퐴 (d) 퐴 + (−퐴) = −퐴 + 퐴 = 0 (e) 휆(퐴 + 퐵) = 휆퐴 + 휆퐵 (f) (휆 + 휇)퐴 = 휆퐴 + 휇퐴 (g) 휆(휇퐴) = (휆휇)퐴 (h) 1퐴 = 퐴 Matrix Multiplication Definition Let 퐴 be 푛 × 푚 and 퐵 be 푚 × 푝. The matrix product 퐶 = 퐴퐵 is the 푛 × 푝 matrix with entries 푚 푐푖푗 = ∑ 푎푖푘푏푘푗 = 푎푖1푏1푗 + 푎푖2푏2푗 + ⋯ + 푎푖푚푏푚푗 푘=1 Special Matrices Definition A square matrix has 푚 = 푛 A diagonal matrix 퐷 = [푑푖푗] is square with 푑푖푗 = 0 when 푖 ≠ 푗 The identity matrix of order 푛, 퐼푛 = [훿푖푗], is diagonal with 1, if 푖 = 푗, 훿푖푗 = { 0, if 푖 ≠ 푗. Definition An upper-triangular 푛 × 푛 matrix 푈 = [푢푖푗] has 푢푖푗 = 0, if 푖 = 푗 + 1, … , 푛. A lower-triangular 푛 × 푛 matrix 퐿 = [푙푖푗] has 푙푖푗 = 0, if 푖 = 1, … , 푗 − 1. Properties Theorem Let 퐴 be 푛 × 푚, 퐵 be 푚 × 푘, 퐶 be 푘 × 푝, 퐷 be 푚 × 푘, and 휆 a real number. (a) 퐴(퐵퐶) = (퐴퐵)퐶 (b) 퐴(퐵 + 퐷) = 퐴퐵 + 퐴퐷 (c) 퐼푚퐵 = 퐵 and 퐵퐼푘 = 퐵 (d) 휆(퐴퐵) = (휆퐴)퐵 = 퐴(휆퐵) Matrix Inversion Definition An 푛 × 푛 matrix 퐴 is nonsingular or invertible if 푛 × 푛 퐴−1 exists with 퐴퐴−1 = 퐴−1퐴 = 퐼 The matrix 퐴−1 is called the inverse of 퐴 A matrix without an inverse is called singular or noninvertible Theorem For any nonsingular 푛 × 푛 matrix 퐴, (a) 퐴−1 is unique (b) 퐴−1 is nonsingular and (퐴−1)−1 = 퐴 (c) If 퐵 is nonsingular 푛 × 푛, then (퐴퐵)−1 = 퐵−1퐴−1 Matrix Transpose Definition 푡 The transpose of 푛 × 푚 퐴 = [푎푖푗] is 푚 × 푛 퐴 = [푎푗푖] A square matrix 퐴 is called symmetric if 퐴 = 퐴푡 Theorem (a) (퐴푡)푡 = 퐴 (b) (퐴 + 퐵)푡 = 퐴푡 + 퐵푡 (c) (퐴퐵)푡 = 퐵푡퐴푡 (d) if 퐴−1 exists, then (퐴−1)푡 = (퐴푡)−1 Determinants Definition (a) If 퐴 = [푎] is a 1 × 1 matrix, then det 퐴 = 푎 (b) If 퐴 is 푛 × 푛, the minor 푀푖푗 is the determinant of the (푛 − 1) × (푛 − 1) submatrix deleting row 푖 and column 푗 of 퐴 푖+푗 (c) The cofactor 퐴푖푗 associated with 푀푖푗 is 퐴푖푗 = (−1) 푀푖푗 (d) The determinant of 푛 × 푛 matrix 퐴 for 푛 > 1 is 푛 푛 푖+푗 det 퐴 = ∑ 푎푖푗퐴푖푗 = ∑(−1) 푎푖푗푀푖푗 푗=1 푗=1 or 푛 푛 푖+푗 det 퐴 = ∑ 푎푖푗퐴푖푗 = ∑(−1) 푎푖푗푀푖푗 푖=1 푖=1 Properties Theorem (a) If any row or column of 퐴 has all zeros, then det 퐴 = 0 (b) If 퐴 has two rows or two columns equal, then det 퐴 = 0 ̃ ̃ (c) If 퐴 comes from (퐸푖) ↔ (퐸푗) on 퐴, then det 퐴 = − det 퐴 ̃ ̃ (d) If 퐴 comes from (휆퐸푖) ↔ (퐸푖) on 퐴, then det 퐴 = 휆 det 퐴 ̃ (e) If 퐴 comes from (퐸푖 + 휆퐸푗) ↔ (퐸푖) on 퐴, with 푖 ≠ 푗, then det 퐴̃ = det 퐴 (f) If 퐵 is also 푛 × 푛, then det 퐴퐵 = det 퐴 det 퐵 (g) det 퐴푡 = det 퐴 (h) When 퐴−1 exists, det 퐴−1 = (det 퐴)−1 (i) If 퐴 is upper/lower triangular or diagonal, then 퐴 = ∏푛 푎 det 푖=1 푖푖 Linear Systems and Determinants Theorem The following statements are equivalent for any 푛 × 푛 matrix 퐴: (a) The equation 퐴x = 0 has the unique solution x = 0 (b) The system 퐴x = b has a unique solution for any b (c) The matrix 퐴 is nonsingular; that is, 퐴−1 exists (d) det 퐴 ≠ 0 (e) Gaussian elimination with row interchanges can be performed on the system 퐴x = b for any b LU Factorization The 푘th Gaussian transformation matrix is defined by 1 0 ⋯ ⋯ 0 ⎡0 ⋱ ⋱ ⋮ ⎤ ⎢ ⎥ ⎢ ⋮ ⋱ ⋱ ⋱ ⋮ ⎥ ⎢ ⋮ 0 ⋱ ⋱ ⋮ ⎥ 푀 (푘) = ⎢ ⋮ ⋮ −푚 ⋱ ⋱ ⋮ ⎥ ⎢ 푘+1,푘 ⎥ ⎢ ⋮ ⋮ ⋮ 0 ⋱ ⋮ ⎥ ⎢ ⋮ ⋮ ⋮ ⋮ ⋱ ⋱ 0⎥ ⎣0 ⋯ 0 −푚푛,푘 0 ⋯ 0 1⎦ LU Factorization Gaussian elimination can be written as (1) (1) (1) 푎11 푎12 ⋯ 푎1푛 ⎡ (2) ⎤ (푛) (푛−1) (1) ⎢ 0 푎22 ⋱ ⋮ ⎥ 퐴 = 푀 ⋯ 푀 퐴 = ⎢ (푛−1) ⎥ ⎢ ⋮ ⋱ ⋱ 푎푛−1,푛⎥ (푛) ⎣ 0 ⋯ 0 푎푛푛 ⎦ LU Factorization Reversing the elimination steps gives the inverses: 1 0 ⋯ ⋯ 0 ⎡0 ⋱ ⋱ ⋮ ⎤ ⎢ ⎥ ⎢ ⋮ ⋱ ⋱ ⋱ ⋮ ⎥ ⎢ ⋮ 0 ⋱ ⋱ ⋮ ⎥ 퐿(푘) = [푀 (푘)]−1 = ⎢ ⋮ ⋮ 푚 ⋱ ⋱ ⋮ ⎥ ⎢ 푘+1,푘 ⎥ ⎢ ⋮ ⋮ ⋮ 0 ⋱ ⋮ ⎥ ⎢ ⋮ ⋮ ⋮ ⋮ ⋱ ⋱ 0⎥ ⎣0 ⋯ 0 푚푛,푘 0 ⋯ 0 1⎦ and we have 퐿푈 = 퐿(1) ⋯ 퐿(푛−1) ⋯ 푀 (푛−1) ⋯ 푀 (1)퐴 = [푀 (1)]−1 ⋯ [푀 (푛−1)]−1 ⋯ 푀 (푛−1) ⋯ 푀 (1)퐴 = 퐴 LU Factorization Theorem If Gaussian elimination can be performed on the linear system 퐴x = b without row interchanges, 퐴 can be factored into the product of lower-triangular 퐿 and upper-triangular 푈 as 퐴 = 퐿푈, (푖) (푖) where 푚푗푖 = 푎푗푖 /푎푖푖 : 푎(1) 푎(1) ⋯ 푎(1) ⎡ 11 12 1푛 ⎤ 1 0 ⋯ 0 0 푎(2) ⋱ ⋮ ⎡푚 1 ⋱ ⋮ ⎤ 푈 = ⎢ 22 ⎥ , 퐿 = ⎢ 21 ⎥ ⎢ (푛−1) ⎥ ⎢ ⋮ ⋱ ⋱ 0⎥ ⎢ ⋮ ⋱ ⋱ 푎푛−1,푛⎥ (푛) ⎣푚 ⋯ 푚 1⎦ ⎣ 0 ⋯ 0 푎푛푛 ⎦ 푛1 푛,푛−1 Permutation Matrices Suppose 푘1, … , 푘푛 is a permutation of 1, … , 푛. The permutation matrix 푃 = (푝푖푗) is defined by 1, if 푗 = 푘푖, 푝푖푗 = { 0, otherwise. (i) 푃 퐴 permutes the rows of 퐴: 푎푘 1 ⋯ 푎푘 푛 ⎡ 1 1 ⎤ 푃 퐴 = ⎢ ⋮ ⋱ ⋮ ⎥ 푎 ⋯ 푎 ⎣ 푘푛1 푘푛푛⎦ (ii) 푃 −1 exists and 푃 −1 = 푃 푡 Gaussian elimination with row interchanges then becomes: 퐴 = 푃 −1퐿푈 = (푃 푡퐿)푈 Diagonally Dominant Matrices Definition The 푛 × 푛 matrix 퐴 is said to be strictly diagonally dominant when |푎푖푖| > ∑ |푎푖푗| 푗≠푖 Theorem A strictly diagonally dominant matrix 퐴 is nonsingular, Gaussian elimination can be performed on 퐴x = b without row interchanges, and the computations will be stable. Positive Definite Matrices Definition A matrix 퐴 is positive definite if it is symmetric and if x푡퐴x > 0 for every x ≠ 0. Theorem If 퐴 is an 푛 × 푛 positive definite matrix, then (a) 퐴 has an inverse (b) 푎푖푖 > 0 (c) max1≤푘,푗≤푛 |푎푘푗| ≤ max1≤푖≤푛 |푎푖푖| 2 (d) (푎푖푗) < 푎푖푖푎푗푗 for 푖 ≠ 푗 Principal Submatrices Definition A leading principal submatrix of a matrix 퐴 is a matrix of the form 푎11 푎12 ⋯ 푎1푘 ⎡푎 푎 ⋯ 푎 ⎤ 퐴 = ⎢ 21 22 2푘⎥ 푘 ⎢ ⋮ ⋮ ⋮ ⎥ ⎣푎푘1 푎푘2 ⋯ 푎푘푘⎦ for some 1 ≤ 푘 ≤ 푛. Theorem A symmetric matrix 퐴 is positive definite if and only if each ofits leading principal submatrices has a positive determinant. SPD and Gaussian Elimination Theorem The symmetric matrix 퐴 is positive definite if and only if Gaussian elimination without row interchanges can be done on 퐴x = b with all pivot elements positive, and the computations are then stable.
Details
-
File Typepdf
-
Upload Time-
-
Content LanguagesEnglish
-
Upload UserAnonymous/Not logged-in
-
File Pages28 Page
-
File Size-