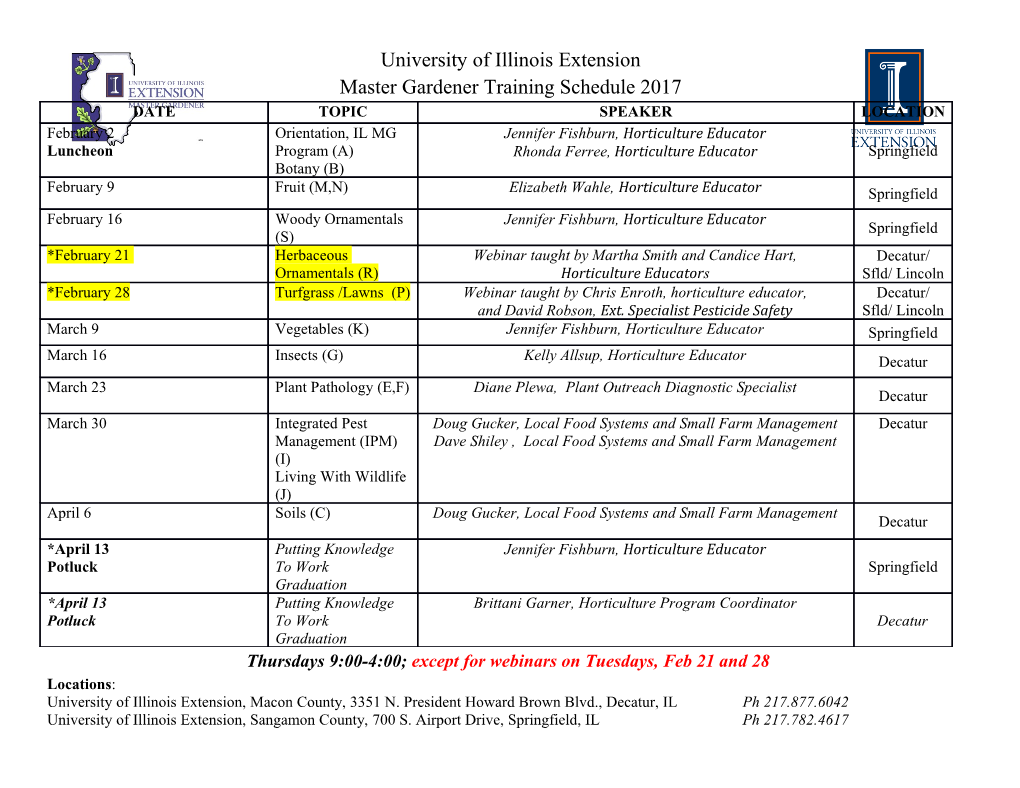
Experiment 7 IMAGE COMPRESSION I Introduction A digital image obtained by sampling and quantizing a continuous tone picture requires an enormous storage. For instance, a 24 bit color image with 512x512 pixels will occupy 768 Kbyte storage on a disk, and a picture twice of this size will not fit in a single floppy disk. To transmit such an image over a 28.8 Kbps modem would take almost 4 minutes. The purpose for image compression is to reduce the amount of data required for representing sampled digital images and therefore reduce the cost for storage and transmission. Image compression plays a key role in many important applications, including image database, image communications, remote sensing (the use of satellite imagery for weather and other earth-resource applications), document and medical imaging, facsimile transmission (FAX), and the control of remotely piloted vehicles in military, space, and hazardous waste control applications. In short, an ever-expanding number of applications depend on the efficient manipulation, storage, and transmission of binary, gray-scale, or color images. An important development in image compression is the establishment of the JPEG standard for compression of color pictures. Using the JPEG method, a 24 bit/pixel color images can be reduced to between 1 to 2 bits/pixel, without obvious visual artifacts. Such reduction makes it possible to store and transmit digital imagery with reasonable cost. It also makes it possible to download a color photograph almost in an instant, making electronic publishing/advertising on the Web a reality. Prior to this event, G3 and G4 standards have been developed for compression of facsimile documents, reducing the time for transmitting one page of text from about 6 minutes to 1 minute. In this experiment, we will introduce the basics of image compression, including both binary images and continuous tone images (gray-scale and color). Video compression will be covered in the next experiment. II Theories and Techniques for Image Compression In general, coding method can be classified into Lossless and Lossy. With lossless coding, the original sample values are retained exactly and compression is achieved by exploring the statistical redundancies in the signal. With lossy coding, the original signal is altered to some extent to achieve a higher compression radio. 1 II.1 Lossless Coding II.1.1 Variable Length Coding [1, Chapter6.4] In variable length coding (VLC), the more probable symbol is represented with fewer bits (using a shorter codeword). The Shannon’s first theorem [3] states that the average length per symbol, l, is bounded by the entropy of source, H, i.e., =−∑∑∑ ≤ = ≤ − + = + HpplplppHnnlog22 nnn (log n11 ) (10.1) where pn is the probability of the n-th symbol, H is the entropy of the source, which represents the average information, ln is the length of the codeword for symbol n, and l is the average codeword length. II.1.2 Huffman Coding The Shannon theorem only gives the bound but not the actual way of constructing the code to achieve the bound. On way to accomplish the later task is by a method known as Huffman Coding. Example: Consider an image that is quantized to 4 levels: 0, 1, 2, and 3. Suppose the probability of these levels are respectively 1/49, 4/49, 36/49 and 8/49. The design of a Huffman code is illustrated in the Figure 1. Symbol Prob Codeword Length 1 “ 2 “ 36/49 “ 1 “ 1 1 1 “ 3 “ 8/49 “ 01 “ 2 0 1 “ 1 “ 4/49 13/49 “ 001 “ 3 0 0 5/49 “ 0 “ 1/49 “ 000 “ 3 Figure 1 An Example of Huffman Coding In this example, we have 2 36 8 4 1 67 Average length l =⋅+⋅++1 2 () ⋅==3 14 . 49 49 49 49 49 =−∑ = Entropy of the source Hppkklog116. ∴<<+H l H 1 II.1.3 Other Variable Length Coding Methods LZW Coding (Lempel, Ziv, And Welsh)[2] is the algorithm used in several public domain software for lossless data compression, such as gzip (UNIX) and pkzip (DOS). One of the most famous graphic file formats GIF also incorporates the LZW coding scheme. Another method known as Arithmetic Coding [2] is more powerful than both Huffman coding and LZW Coding. But it also requires more computation. II.1.4 Runlength Coding (RLC) of Bilevel Images [1, Chapter 6.6] In one dimensional runlength coding of bilevel images, one scans the pixels from left to right along each scan line. Assume that a line always starts and ends with white pixels, one counts the number (referred to as runlength) of white pixels and that of the black pixels alternatively. The last run of white pixels are replaced with a special symbol “EOL” (end of line). The runlengths of white and black are coded using separate codebooks. The codebook, say, for the white runlengths is designed using Huffman Coding method by treating each possible runlength (including EOL) as a symbol. An example of runlengths Coding is illustrated in the Fig. 2. 3 ⊗ EOL (End of line) RUN-LENGTH CODING → White Runlength ⇒ Black Runlength ------------------------------------------------------------------- ------------------------------------------------------------------- - - x x x x - - x - - - - - x x x x - - x x x x - - x x x x - - - - x - - - - - x - - - - - x - - - - - x - - x - - - - - x - - - - x - - - - - x - - - - - x - - - - - x - - x - - - - - x - - - - x x x x - - x - - - - - x x x x - - x x x x - - x x x x - - - - x - - - - - x - - - - - - - - x - - - - - x - - - - - x - - - - x - - - - - x - - - - - - - - x - - - - - x - - - - - x - - - - x x x x - - x x x x - x x x x - - x x x x - - x x x x - - ------------------------------------------------------------------- ------------------------------------------------------------------- ⊗ ⊗ 2 4 2 1 5 4 2 4 2 4 → ⇒ → ⇒ → ⇒ → ⇒ → ⇒ ⊗ 2 1 5 1 5 1 5 1 2 1 2 1 → ⇒ → ⇒ → ⇒ → ⇒ → ⇒ → ⇒ ⊗ . 2 4 2 4 1 4 2 4 2 4 → ⇒ → ⇒ → ⇒ → ⇒ → ⇒ ⊗ Fig. 2 An example of runlength coding II.1.5 Two Dimensional Runlength Coding [1, Chapter 6.6] One dimensional runlength coding method only explores the correlation among pixels in the same line. In two dimensional runlength coding or relative address coding, the correlation among pixels in the current line as well as the previous line is explored. With this method, when a transition in color occurs, the distance of this pixel to the most closest transition pixel (both before and after this pixel) in the previous line as well as to the last transition pixel in the same line are calculated, and the one with the shortest distance is coded, along with an index indicating which type of distance is coded. See Fig. 6.17 in [1]. 4 II.1.6 CCITT Group 3 and Group 4 Facsimile Coding Standard - The READ Code [1,Chapter 6.6] In the Group 3 method, the first line in every K lines is coded using 1-D runlength coding, and the following (K-1) lines are coded using a 2-D runlength coding method known as Relative Element Address Designate (READ). For details of this method and the actual code tables, see [1], Sec. 6.6.1. The reason that the 1-D RLC is used for every K line is to suppress propagation of transmission errors. Otherwise, if the READ method is used continuously, when one bit error occurs somewhere during transmission, it will affect the entire page. The Group 4 method is designed for more secure transmission media, such as leased data lines where the bit error rate is very low. The algorithm is basically a streamline of the Group 3 method, with 1-D RLC eliminated. II.1.7 Lossless Predictive Coding Motivation: The value of a current pixel usually does not change rapidly from those of adjacent pixels. Thus it can be predicted quite accurately from the previous samples. The prediction error will have a non-uniform distribution, centered mainly near zero, which has a lower entropy than the original samples, which usually have a uniform distribution. For detail see [2] Sec. 9.4. With entropy coding (e.g. Huffman coding), the error values can be specified with fewer bits than that required for specifying the original sample values. II.2 Transform Coding (Lossy Coding) [1,Chapter 6.5] Lossless coding can achieve a compression ratio of 2 -- 3 for most images. To further reduce the data amount, lossy coding methods apply quantization to the original samples or parameters of some transformation of the original signal ( e.g. prediction or transformation). The transformation is to exploit the statistical correlation among original samples. Popular methods include linear prediction and unitary transforms. We have discussed linear prediction coding and its application in speech and audio coding in the previous experiment. You have learnt and experimented with uniform and non-uniform quantization in the previous experiment as well. In this section, we focus on transform coding, which is more effective for images. One of the most popular lossy coding schemes for images is transform coding. In block-based transform coding, one divides an image into non-overlapping blocks. For each block, one first transforms the original pixel values into a set of transform coefficients using a unitary transform. The transformed coefficients are then quantized and coded. In the decoder, one reconstructs the original block from the quantized coefficients through an inverse transform. The transform is designed to compact the energy of the original signal into only a few coefficients, and to reduce the correlation among the variables to be coded. Both will contribute to the reduction of the bit rate. 5 II.2.1 The Discrete Cosine Transformation (DCT) The DCT is popular with image signals because it matches well with the statistics of common image signals. The basis vectors of the one dimensional N-point DCT are defined by: 1 = ()21nk+ π k 0 hn()= α ()cos( k ),withα ( k ) = N k 2N 2 kN=−12, ,..., 1 .
Details
-
File Typepdf
-
Upload Time-
-
Content LanguagesEnglish
-
Upload UserAnonymous/Not logged-in
-
File Pages15 Page
-
File Size-