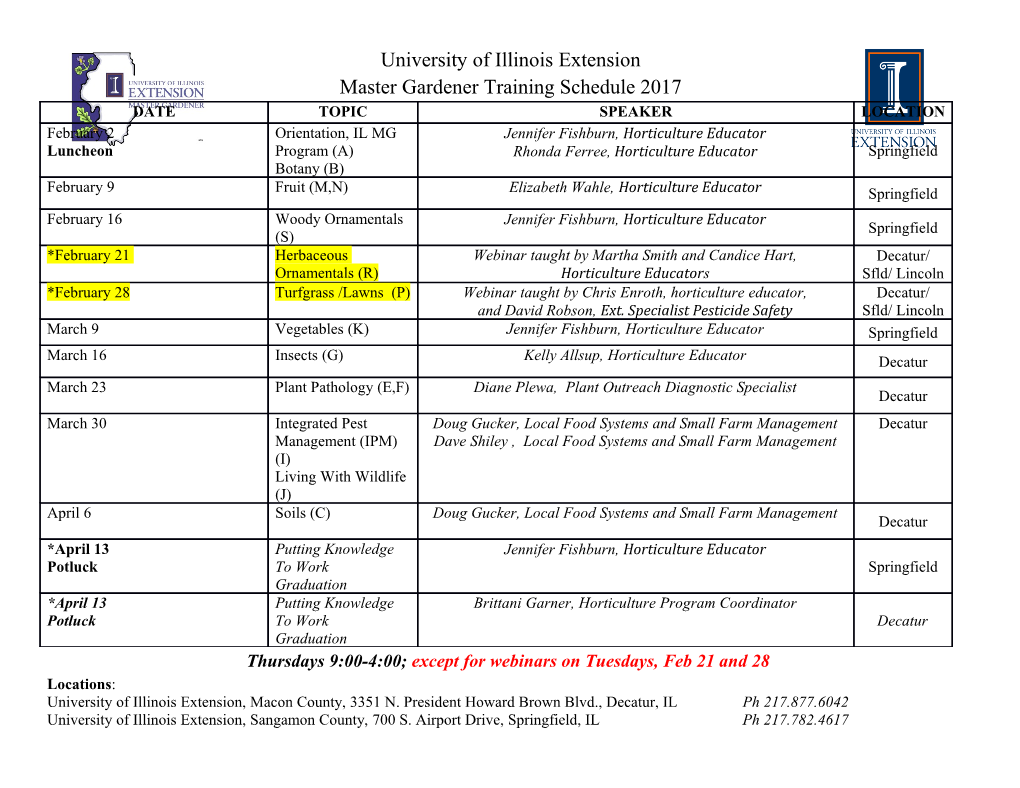
Information theory and its application to optical communication Citation for published version (APA): Willems, F. M. J. (2017). Information theory and its application to optical communication. In Signal Processing in Photonic Communications 2017, 24-27 July 2017, New Orleans, Louisiana [SpTu3E] Optical Society of America (OSA). https://doi.org/10.1364/SPPCOM.2017.SpTu3E.1 DOI: 10.1364/SPPCOM.2017.SpTu3E.1 Document status and date: Published: 01/01/2017 Document Version: Publisher’s PDF, also known as Version of Record (includes final page, issue and volume numbers) Please check the document version of this publication: • A submitted manuscript is the version of the article upon submission and before peer-review. There can be important differences between the submitted version and the official published version of record. People interested in the research are advised to contact the author for the final version of the publication, or visit the DOI to the publisher's website. • The final author version and the galley proof are versions of the publication after peer review. • The final published version features the final layout of the paper including the volume, issue and page numbers. Link to publication General rights Copyright and moral rights for the publications made accessible in the public portal are retained by the authors and/or other copyright owners and it is a condition of accessing publications that users recognise and abide by the legal requirements associated with these rights. • Users may download and print one copy of any publication from the public portal for the purpose of private study or research. • You may not further distribute the material or use it for any profit-making activity or commercial gain • You may freely distribute the URL identifying the publication in the public portal. If the publication is distributed under the terms of Article 25fa of the Dutch Copyright Act, indicated by the “Taverne” license above, please follow below link for the End User Agreement: www.tue.nl/taverne Take down policy If you believe that this document breaches copyright please contact us at: [email protected] providing details and we will investigate your claim. Download date: 03. Oct. 2021 Information Theory and its Application to Optical Communication FMJ Willems INTRODUCTION INFORMATION THEORY Information Theory and its Application to Optical WAVEFORM Communication CHANNELS AGN CAPACITY SOME CODES Frans M.J. Willems CODED MODULATION SHAPING CODES ICT Lab, SPS Group Department of Electrical Engineering REMARKS Eindhoven University of Technology SPPCom, Advanced Photonics 2017, July 25, New Orleans Introduction Information Theory and its Application to Optical Communication FMJ Willems Optical communication links carry most of the data that is transmitted INTRODUCTION around the world. Home connections are being replaced by optical links. INFORMATION THEORY We like to achieve the highest possible data rates for the smallest cost. Replacing links should be delayed as long as possible. WAVEFORM CHANNELS Therefore advance transmission protocols (equalisation, modulation, AGN CAPACITY coding) are required. SOME CODES INFORMATION THEORY tells us what the ultimate performances are CODED (e.g. capacity), and what the techniques are that achieve ultimate MODULATION performance. SHAPING CODES Wireless communication is characterized by major developments (coding, REMARKS mimo, cooperative communications, etc.), often boosted by information theoretical methods. Optical Communication is going through a similar innovation cycle now. Information theory can also be useful here. Claude Shannon (1916-2001) Information Theory and its Application to Optical Communication 1948: \A Mathematical Theory of Communication," FMJ Willems Bell Syst. Tech. J.: Shannon combined the noise power spectral density N0=2, the channel bandwidth INTRODUCTION W , and the transmit power P; into a single parameter INFORMATION C; which he called the channel capacity. More THEORY precisely Shannon Entropy, Conditional P Entropy, Mutual C = W log2(1 + ) Information N0W Capacity Discrete Memoryless Channel represents the maximum number of bits that can be Capacity Gaussian Channel sent per second reliably from transmitter to receiver. WAVEFORM Codes can be used to achieve capacity. CHANNELS 1938: Shannon also applied Boole's algebra to AGN CAPACITY switching circuits (MSc thesis, MIT). SOME CODES WW2: Shannon developed cryptographic equipment CODED for transoceanic conferences (Roosevelt-Churchill). His MODULATION ideas can be found in \Communication Theory of SHAPING CODES Secrecy Systems", a confidential report from 1945, REMARKS published in 1949. 1949: Shannon introduced the sampling theorem to the engineering community. Entropy Information Theory and its Application to Let X be a discrete random variable with alphabet X and probability mass Optical Communication function p(x) = PrfX = xg for x 2 X . FMJ Willems Definition INTRODUCTION The entropy H(X ) of discrete random variable X is defined as INFORMATION THEORY Shannon X 1 H(X ) = p(x) log [bit]: Entropy, Conditional 2 p(x) Entropy, Mutual x2X Information Capacity Discrete Memoryless Channel Capacity Gaussian Channel Example WAVEFORM CHANNELS Binary random variable X with alphabet X = f0; 1g. Let AGN CAPACITY 0 with probability 1 − p; X = SOME CODES 1 with probability p: CODED MODULATION Then the entropy of X is SHAPING CODES H(X ) = h(p); REMARKS where ∆ 1 1 h(p) = (1 − p) log + p log [bit]: 2 1 − p 2 p Entropy, Binary Entropy Function Information Theory and its Application to Optical Example Communication 1 FMJ Willems 0.9 INTRODUCTION 0.8 INFORMATION THEORY 0.7 Shannon Entropy, Conditional 0.6 Entropy, Mutual Information 0.5 Capacity Discrete Memoryless Channel h(p) (bit) 0.4 Capacity Gaussian Channel 0.3 WAVEFORM CHANNELS 0.2 AGN CAPACITY 0.1 SOME CODES 0 0 0.1 0.2 0.3 0.4 0.5 0.6 0.7 0.8 0.9 1 CODED p MODULATION SHAPING CODES Observe that h(p) = h(1 − p) and that e.g. h(0:1) = 0:4690. REMARKS Think of H(X ) as the uncertainty in X . It can be shown that 0 ≤ H(X ) ≤ log2 jX j. Conditional Entropy Information Theory Let X and y be discrete random variables with alphabets X and Y and its Application to Optical respectively and joint probability mass function p(x; y) = PrfX = x; Y = yg Communication for x 2 X and x 2 Y. P FMJ Willems Note that p(y) = x2X p(x; y) and p(xjy) = p(x; y)=p(y). INTRODUCTION Definition INFORMATION THEORY The conditional entropy H(X jY ) of discrete random variable X given Y is Shannon defined as Entropy, Conditional Entropy, Mutual Information X X 1 Capacity Discrete H(X jY ) = p(y) p(xjy) log2 [bit]: Memoryless Channel p(xjy) Capacity Gaussian y2Y x2X Channel WAVEFORM CHANNELS Think of H(X jY ) as the uncertainty in X when Y is given. It can be shown that 0 ≤ H(X jY ) ≤ H(X ). Conditioning can only reduce AGN CAPACITY entropy. SOME CODES Note also that CODED X MODULATION H(X jY ) = p(y)H(X jY = y); y2Y SHAPING CODES where REMARKS X 1 H(X jY = y) = p(xjy) log : 2 p(xjy) x2X Conditional entropy H(X jY ) is the expected value of entropies H(X jY = y) w.r.t. p(y). Example: Binary Symmetric Channel (BSC) Information Theory and its Application to Example Optical Communication Transition probabilities p(Y = 1jX = 0) = p(Y = 0jX = 1) = p. FMJ Willems X = 1 Y = 1 1 − p INTRODUCTION p INFORMATION THEORY p Shannon 1 − p Entropy, Conditional Entropy, Mutual X = 0 Y = 0 Information Capacity Discrete For uniform p(X = 0) = p(X = 1) = 1=2 we obtain that the entropy Memoryless Channel Capacity Gaussian Channel H(X ) = h(1=2) = 1: WAVEFORM CHANNELS Moreover: AGN CAPACITY SOME CODES p(Y = 1) = p(X = 0) · p + p(X = 1) · (1 − p) = 1=2; CODED p(X = 1; Y = 0) p(X = 1) · p MODULATION p(X = 1jY = 0) = = = p; p(Y = 0) p(Y = 0) SHAPING CODES p(X = 1; Y = 1) p(X = 1) · (1 − p) REMARKS p(X = 1jY = 1) = = = 1 − p; p(Y = 1) p(Y = 1) and the conditional entropy H(X jY ) = p(Y = 0)h(p) + p(Y = 1)h(1 − p) = h(p): Mutual Information Information Theory and its Application to Definition Optical Communication The mutual information I (X ; Y ) between the discrete random variable X and FMJ Willems Y is defined as I (X ; Y ) = H(X ) − H(X jY ) [bit]. INTRODUCTION INFORMATION THEORY Think of I (X ; Y ) as the decrease in uncertainty about X when Y is Shannon released. Equivalently it is the information that Y contains about X . Entropy, Conditional Entropy, Mutual It can be shown that always 0 ≤ I (X ; Y ) ≤ H(X ). Information I (X ; Y ) is also the decrease in uncertainty about Y when X is released. Capacity Discrete Memoryless Channel Capacity Gaussian Example Channel WAVEFORM Binary symmetric channel (BSC) with transition probability p: CHANNELS X = 1 Y = 1 AGN CAPACITY 1 − p SOME CODES p CODED p MODULATION 1 − p SHAPING CODES X = 0 Y = 0 REMARKS For uniform p(X = 0) = p(X = 1) = 1=2, we obtain that I (X ; Y ) = H(X ) − H(X jY ) = 1 − h(p): For p = 0:1 we obtain I (X ; Y ) = 1 − 0:4690 = 0:5310 bit. Discrete Memoryless Channel Information Theory and its Application to Optical Communication Definition FMJ Willems Channel input alphabet X , channel output alphabet Y. INTRODUCTION For each x 2 X the transition probabilities PrfY = yjX = xg for y 2 Y are P INFORMATION denoted by p(yjx), where y2Y p(yjx) = 1. THEORY Shannon Entropy, Conditional Entropy, Mutual Example Information Capacity Discrete Memoryless Channel p(yjx) Capacity Gaussian ∆ ∆ Channel jX j jYj Here X = f1; 2; ··· ; jX jg and Y = WAVEFORM f1; 2; ··· ; jYjg. CHANNELS AGN CAPACITY . SOME CODES . CODED MODULATION 2 2 SHAPING CODES REMARKS 1 1 Channel Capacity, Definition Information Theory and its Application to Observe that: Optical Communication the channel input distribution fp(x); x 2 X g determines the joint FMJ Willems distribution INTRODUCTION p(x; y) = p(x)p(yjx); for all x 2 X ; y 2 Y; INFORMATION THEORY and therefore the mutual information Shannon Entropy, Conditional Entropy, Mutual I (X ; Y ) = H(X ) − H(X jY ): Information Capacity Discrete Memoryless Channel The maximum value of I (X ; Y ) is called the channel capacity C.
Details
-
File Typepdf
-
Upload Time-
-
Content LanguagesEnglish
-
Upload UserAnonymous/Not logged-in
-
File Pages86 Page
-
File Size-