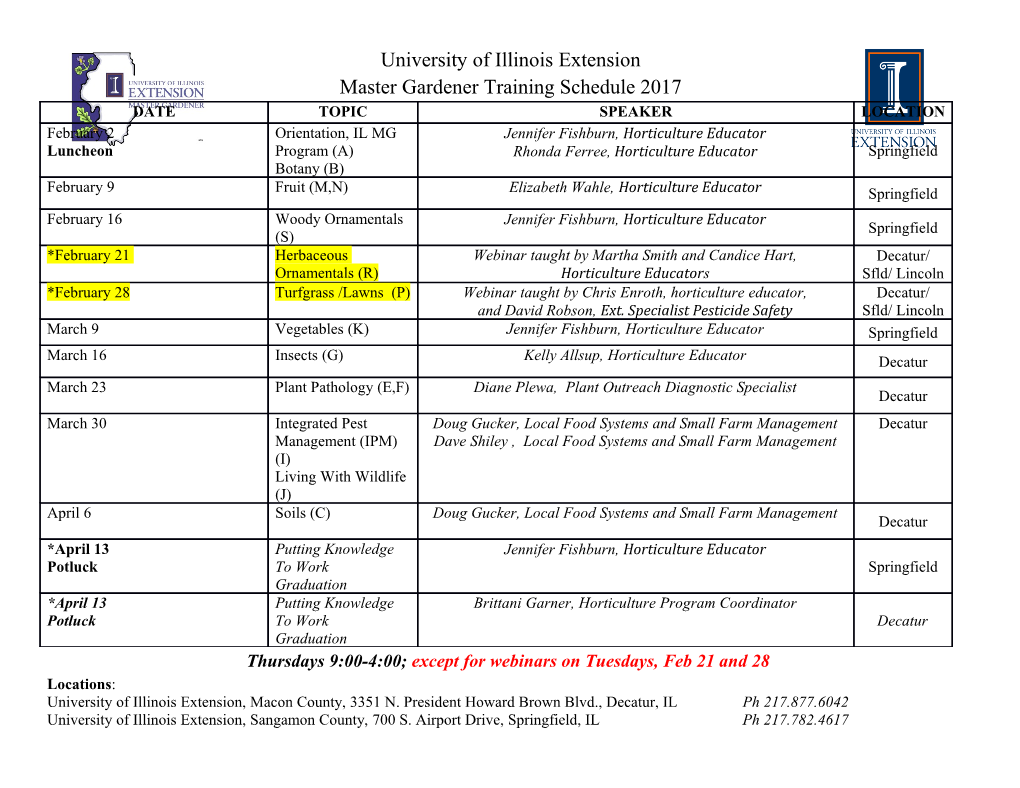
Machine Learning Empirical Risk Minimization Dmitrij Schlesinger WS2014/2015, 08.12.2014 Recap – tasks considered before n For a training dataset L = (xl, yl) ... with xl ∈ R (data) and yl ∈ {+1, −1} (classes) fing a separating hyperplane yl · [hw, xli + b] ≥ 0 ∀l → Perceptron algorithm The goal is to fing a "corridor" (stripe) of the maximal width that separates the data → Large Margin learning, linear SVM 1 kwk2 → min 2 w s.t. yl[hw, xli + b] ≥ 1 In both cases the data is assumed to be separable. What if not? ML: Empirical Risk Minimization 08.12.2014 2 Empirical Risk Minimization Let a loss function C(y, y0) be given that penalizes deviations between the true class and the estimated one (like the loss in the Bayesian Decision theory). The Empirical Risk of a decision strategy is the total loss over the training set: X R(e) = C yl, e(xl) → min e l It should bi minimized with respect to the decision strategy e. Special case (today): – the set of decisions is {+1, −1} – the loss is the delta-function C(y, y0) = δ(y 6= y0) – the decision strategy can be expressed in the form e(x) = sign f(x) with an evaluation function f : X → R Example: f(x) = hw, xi − b is a linear classifier. ML: Empirical Risk Minimization 08.12.2014 3 Hinge Loss The problem: the subject is not convex The way out: replace the real loss by its convex upper bound ← example for y = 1 (for y = −1 it should be flipped) δ y 6= sign f(x) ≤ max 0, 1 − y · f(x) It is called Hinge Loss ML: Empirical Risk Minimization 08.12.2014 4 Sub-gradient algorithm Let the evaluation function be parameterized, i.e. f(x; θ) for example θ = (w, b) for linear classifiers The optimization problem reads: X H(θ) = max 0, 1 − yl · f(xl; θ) → min θ l It is convex with respect to f but not differentiable Solution by the sub-gradient (descent) algorithm: 1. Compute the sub-gradient (later) 2. Apply it with a step size that is decreasing in time ∂H θ(t+1) = θ(t) − γ(t) ∂θ ∞ with lim γ(t) = 0 and P γ(t) = ∞ (e.g. γ(t) = 1/t) t→∞ t=1 ML: Empirical Risk Minimization 08.12.2014 5 The sub-gradient for the Hinge loss X H(θ) = max 0, 1 − yl · f(xl; θ) → min θ l 1. Estimate data points with the Hinge grater zero 0 L = {l : yl · f(xl) < 1} 2. The sub-gradient is ∂H X ∂f(xl; θ) = − yl · ∂θ l∈L0 ∂θ In particular, for linear classifiers ∂H X = − yl · xl ∂θ l∈L0 i.e. some data points are added to the parameter vector → it reminds on the Perceptron algorithm ML: Empirical Risk Minimization 08.12.2014 6 Kernelization Let us optimize the Hinge loss in a feature space Φ: X → H, → the (linear) evaluation function is not f(x; w) = hx, wi but f(x; w) = hΦ(x), wi Remember (see the previous lecture) that the evaluation function can be expressed as a linear combination X f(x; α) = hΦ(x), wi = hΦ(x), αiyiΦ(xi)i = i X X = αiyihΦ(x), Φ(xi)i = αiyiκ(x, xi) i i The subject to be minimized reads now X X H(α) = max 0, 1 − αiyiκ(xl, xi) → min α l i ML: Empirical Risk Minimization 08.12.2014 7 Kernelization The task: X X H(α) = max 0, 1 − αiyiκ(xl, xi) → min α l i The sub-gradient: ∂H X = −yi ylκ(xl, xi) ∂αi l∈L0 As usual for kernels neither the feature space H nor the mapping Φ(x) are necessary in order to estimate α-s, if the kernel κ(x, x0) is given !!! ML: Empirical Risk Minimization 08.12.2014 8 Maximum margin vs. minimum loss – Linear SVM: maximum margin, separable data – Non-separable data: min. Empirical Risk, Hinge loss – "Kernelization" can be done for both variants Does is have sense to to minimize loss (especially the Hinge loss, which has a "geometrical nature") defined in the feature space ? It is indeed always possible to make the training set separable by choosing a suitable kernel. Interesting – both formulations are equivalent in certain circumstances. ML: Empirical Risk Minimization 08.12.2014 9 A "unified" formulation In Machine Learning it is a common technique to enhance an objective function (e.g. the average loss) by a regularizer C X R(w) + `(xl, yl, w) |L| l with – parameter vector w – loss `(xl, yl, w), e.g. delta, Hinge, metric, additive etc. 2 – regularizer R(w), e.g. ||w|| , |w|1 etc. – balancing factor C Now: we start from the unified formulation, make some assumption and end up with the maximum margin learning ML: Empirical Risk Minimization 08.12.2014 10 Hinge loss → maximum margin C X R(w) + `(xl, yl, w) → min w |L| l Assumption: the training set is separable, i.e. there exists such w that the loss is zero. Set C to a very high value and obtain R(w) → min w s.t. `(xl, yl, w) = 0 ∀l Set R(w) = 1/2||w||2 and ` to the Hinge loss (for linear cl.) `(xl, yl, w) = max(0, 1 − ylhw, xli) = 0 It results in the maximum margin learning 1 ||w||2 → min 2 w s.t. ylhw, xli ≥ 1 ∀l ML: Empirical Risk Minimization 08.12.2014 11 Maximum margin → Hinge loss Let us "weaken" the maximum margin learning – we introduce so-called slack-variables ξl ≥ 0 that represent "errors": 1 2 C X ||w|| + ξl → min w 2 |L| l s.t. ylhw, xli ≥ 1 − ξl ∀l Note that we want to minimize the total error. On the other hand ξl ≥ 1 − ylhw, xli and ξl ≥ 0 ⇒ ξl = max(0, 1 − ylhw, xli) Substituting it gives 1 2 C X ||w|| + max(0, 1 − ylhw, xli) → min w 2 |L| l i.e. the Hinge loss minimization ML: Empirical Risk Minimization 08.12.2014 12 Summary C X R(w) + `(xl, yl, w) → min w |L| l Two extremes: – Big C → the loss is more important → better recognition rate but smaller margin (worse generalization) – Small C → the generalization is more important → larger margin (more robust classifier) but worse recognition rate Recommended reading: Sebastian Nowozin and Christopher H. Lampert, "Structured Prediction and Learning in Computer Vision", Foundations and Trends in Computer Graphics and Vision, Vol. 6, Nr. 3-4 http://www.nowozin.net/sebastian/cvpr2012tutorial ML: Empirical Risk Minimization 08.12.2014 13.
Details
-
File Typepdf
-
Upload Time-
-
Content LanguagesEnglish
-
Upload UserAnonymous/Not logged-in
-
File Pages13 Page
-
File Size-