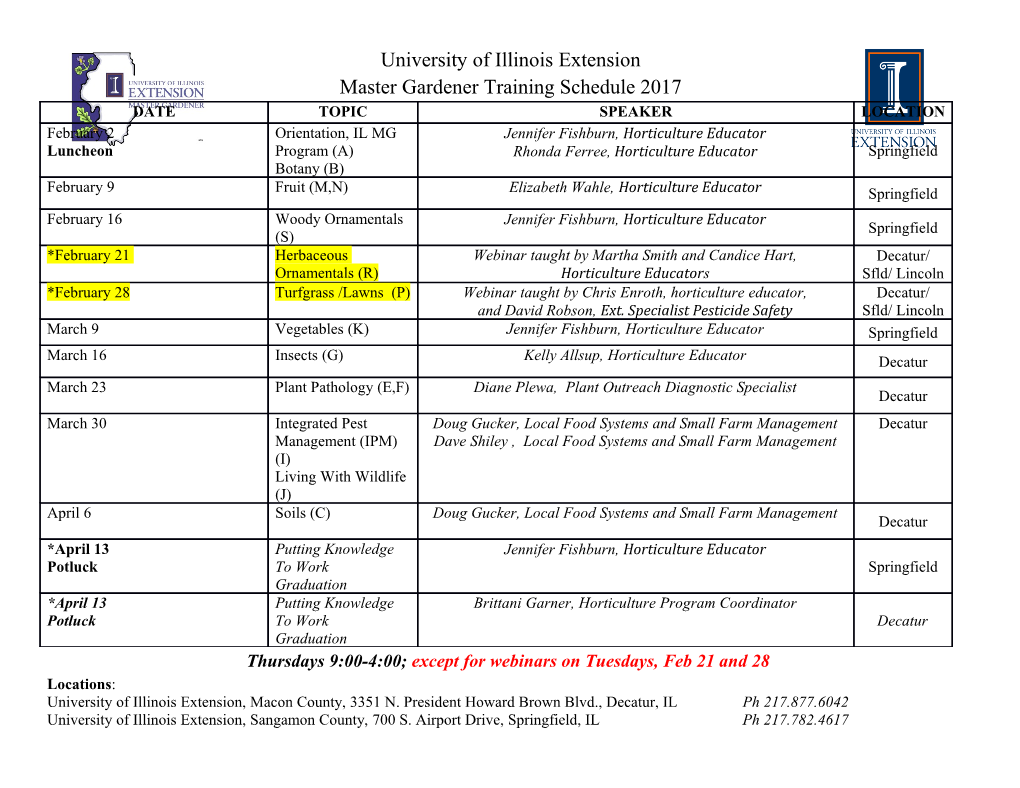
Center for Statistics and Applications in CSAFE Presentations and Proceedings Forensic Evidence 10-17-2017 What is Forensic Statistics, and What Does it Mean for Forensic Pattern Recognition Simon A. Cole University of California, Irvine Follow this and additional works at: https://lib.dr.iastate.edu/csafe_conf Part of the Forensic Science and Technology Commons Recommended Citation Cole, Simon A., "What is Forensic Statistics, and What Does it Mean for Forensic Pattern Recognition" (2017). CSAFE Presentations and Proceedings. 54. https://lib.dr.iastate.edu/csafe_conf/54 This Presentation is brought to you for free and open access by the Center for Statistics and Applications in Forensic Evidence at Iowa State University Digital Repository. It has been accepted for inclusion in CSAFE Presentations and Proceedings by an authorized administrator of Iowa State University Digital Repository. For more information, please contact [email protected]. What is Forensic Statistics, and What Does it Mean for Forensic Pattern Recognition Disciplines Forensic Science and Technology Comments Posted with permission of CSAFE. This presentation is available at Iowa State University Digital Repository: https://lib.dr.iastate.edu/csafe_conf/54 What Is Forensic Statistics, and What Does it Mean for Forensic Pattern Recognition? Arizona Identification Council Tri-Division Educational Conference October 17, 2017 Simon A. Cole Department of Criminology, Law & Society Newkirk Center for Science & Society Center for Statistical Applications of Forensic Evidence University of California, Irvine NIST OSAC NIST OSAC NIST OSAC US Department of Justice, Forensic Science Discipline Review (2016) CSAFE Which discipline? • Biology? • Chemistry? • Engineering? • STS? – See “STS on Trial” and remarks by Downey tomorrow; interventions by Edmond, Mnookin, etc. • Psychology? – Human factors, bias, training, innate abilities • Statistics – How and why has statistics emerged as the panacea for forensic science? Questions 1. Why is this happening? 2. Why is this important? History of probability theory • Pascal (17th cent.) • Laplace (19th cent.) When did expert evidence become statistical? • Dreyfus Affair (France 1894) • Questioned document • Poincare uses Bayesian approach to refute Bertillon’s mathematical report – Taroni, et al., “Forerunners of Bayesianism in Early Forensic Science, Jurimetrics, Vol. 38 (1998), 183- 200. Howland will • Famous philosopher C.S. Peirce and father Benjamin Peirce calculated probability of similarity of two signatures – Meier & Zabell, “Benjamin Peirce and the Howland Will,” Journal of the Statistical Association, Vol. 75 (1980), 497. Locard tripartite rule – fingerprinting (1912) Corresponding friction ridge details Justifiable conclusion < 8 Cannot identify 8-12 Probable identification > 12 Identification • Arguably a probabilistic understanding of fingerprint evidence that was subsequently lost in the discipline. Non-statistical approach • Compare refractive indices of glass from crime scene and suspect • If indices are “consistent” (using some measure of “consistency”), then report . – Glass on suspect might/must have come from crime scene Lindley’s approach • Need to know: – likelihood of consistent glass fragments if suspect was present at crime scene – likelihood of consistent glass fragments if suspect was NOT present at crime scene – likelihood of NOT consistent glass fragments if suspect was present at crime scene – likelihood of NOT consistent glass fragments if suspect was NOT present at crime scene Lindley’s approach 1. Likelihood ratio 2. Bayes theorem 3. Decision theory Likelihood ratios NIST/NIJ Report at 134 Tenets of forensic statistics • All evidence is probabilistic • All evidence can be characterized by a LR • All evidence can be integrated into a case decision using Bayes theorem Implications for forensic science • All evidence must be characterized probabilistically • Probably through a LR • Forensic analysts should not testify to posterior odds Forensic statistics • Over the next ~30 years, “forensic statistics” become recognizable subdiscipline – Not quite “statistics” and not quite “forensic science” • Conferences, journals, and other markers of academic legitimacy – 1990 – 1st International Conference on Forensic Statistics (Edinburgh) • 83 attendees – 2017 – 10th International Conference on Forensic Inference and Statistics (Minneapolis) • 148 attendees Tenets of forensic statistics • All evidence is probabilistic • All evidence can be characterized by a LR • All evidence can be integrated into a case decision using Bayes theorem Events Events Events • Colin Aitken et al., “Fundamentals of Probability and Statistical Evidence in Criminal Proceedings: Guidance for Judges, Lawyers, Forensic Scientists and Expert Witnesses” (Royal Statistical Society, 2010). Probabilistic nature of evidence (Cromwell’s rule) • Dennis Lindley, Understanding Uncertainty (2006), 91. Denial of probability: fingerprints • Fingerprint evidence is non-probabilistic – Vanderkolk (1993) • “Dactyloscopy is a domain separate from criminalistics. The information is certain; a fingerprint can only be from one person.” – Grieve quoted in Champod (1995) “any member, officer or certified latent print examiner who provides oral or written reports, or gives testimony of possible, probable, or likely friction ridge identification shall be deemed to be engaged in conduct unbecoming such member, officer, or certified latent print examiner.” – IAI Resolution VII (1979) [amended by Resolution V (1980)] Champod & Evett (2001) Likelihood ratios NIST/NIJ Report at 134 • Danger of transposed conditional • Later known as “prosecutor’s fallacy” • Thompson & Schumann, “Interpretation of Statistical Evidence in Criminal Trials,” Law & Human Behavior Vol. 11 (1987), 167-187. “Bayes’s theorem” Prior Odds Evidence Posterior odds Likelihood Likelihood Likelihood of guilt of of guilt with no evidence if evidence D. guilty _________ * _________ = _________ Likelihood Likelihood Likelihood of of of innocence evidence if innocence with no D. evidence innocent Bayesian approach • LR should be distinguished from “Bayesian” approach • LR is used in “Bayes theorem” • But you can use LR without using a “Bayesian approach” • Well suited for single events in which you cannot run repeated trials • Good at combining heterogeneous evidence • Subjective probabilities ok • Arguably very well suited for legal problems • Reputation for punctiliousness • Dogmatic quality – Religion jokes Jury comprehension Likelihood ratio approach Current approach • Can juries understand • Ambiguity around “match” likelihood ratios – Defendant only possible source? – Defendant a member of a group of • Innumeracy possible sources? • How many? • Susceptibility to logical • Ambiguity around “identification” fallacies – Defendant only possible source? – E.g., prosecutor’s fallacy – Defendant a member of a group of possible sources? – Defense attorney’s fallacy • How many? • Historical and vernacular meaning • Jury comprehension of word “identification” demanded • Jury comprehension not demanded Decision Theory approach 1. Form a LR based on the print evidence 2. Calculate a “utility function” – Consequences, preferences, and utilities of each decision being right and wrong • E.g., wrongful conviction vs. wrongful acquittal; death penalty 3. Combining the LR and the utility function, decide whether you want to behave as if the prints come from the same source or from different sources – Biedermann, et al., “Decision Theoretic Properties of Forensic Identification, Forensic Science International, Vol. 177 (2008). Decision Theory graphical Possible Ground truth Consequence Potential legal decisions consequence Correct Rightful implication conviction True False Wrongful Same source implication conviction False Identification LR Utility function evidence Decision True Correct Different Rightful exculpation source acquittal False False Wrongful exculpation acquittal Consequences of decision theory • Output is never “true,” but a decision to behave as if something were true, based on both the relatively likelihood of it being true and one’s preferences regarding the consequences of being right and wrong • But whose preferences? – The expert’s? • It can’t be • But that is the system we are using now! – It must be the fact-finder • If so, then expert must confine herself to reporting the LR only, not a decision • Of course, then someone has to explain all this to the fact-finder Forensic statistics in practice c. 2010 • Netherlands Forensic Institute • Swedish National Laboratory • UK Forensic Science Service – Closure announced 2010 R. v. T. (UK 2010) • Shoe print comparison • Witness gives likelihood ratio based on subjective probabilities, rather than formal data, and back- of-the-envelope calculations • Evidence excluded • Looks like repudiation of forensic statistics • Or, could be an insistence on formal data • Either way, undermined practitioners confidence in forensic statistics Post-2010 • Increasing institutional acceptance • Could forensic science be turning statistical? Appeal of forensic statistics • Packaged approach • More or less same approach to all evidence • Broad consensus around approach (paradigm?) Likelihood ratio approach (Neumann) Kelly Servick, “Sizing up the Evidence,” Science, Vol. 351 (Mar. 11, 2016), 1131. breakdown (external)? Accepting a paradigm • Just because a paradigm is dominant does not compel acceptance • Court could reason that: – This is a practice for which
Details
-
File Typepdf
-
Upload Time-
-
Content LanguagesEnglish
-
Upload UserAnonymous/Not logged-in
-
File Pages52 Page
-
File Size-