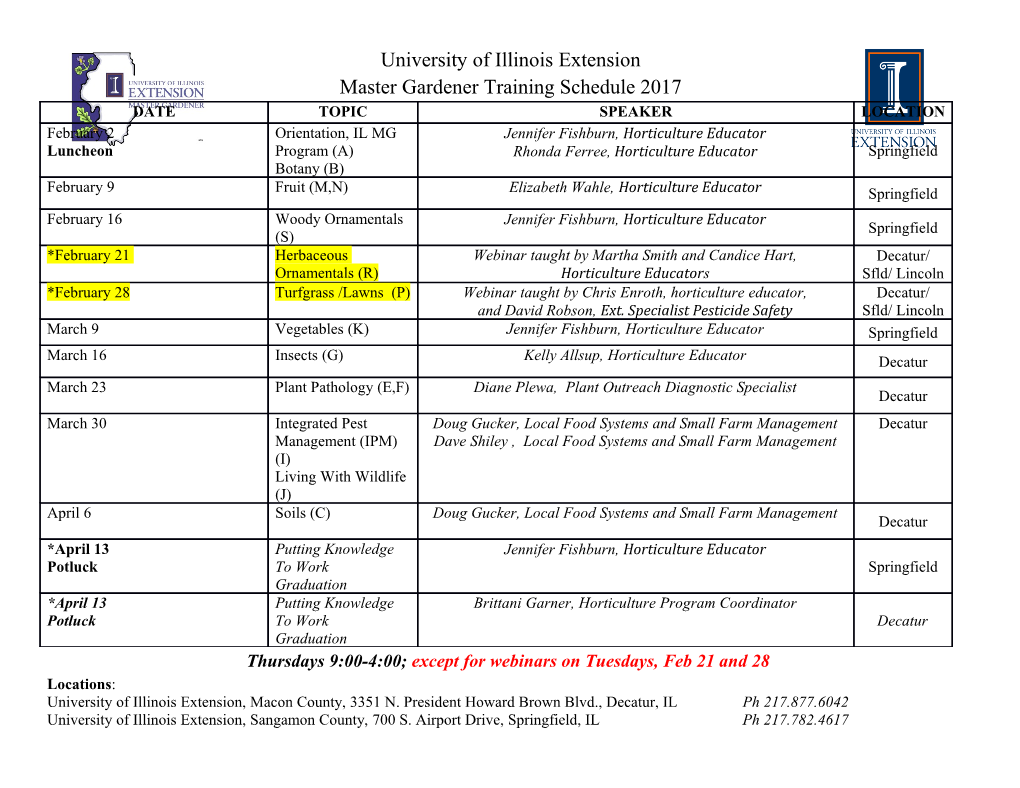
Exploring the Limits of Language Modeling Rafal Jozefowicz [email protected] Oriol Vinyals [email protected] Mike Schuster [email protected] Noam Shazeer [email protected] Yonghui Wu [email protected] Google Brain Abstract language models improves the underlying metrics of the In this work we explore recent advances in Re- downstream task (such as word error rate for speech recog- current Neural Networks for large scale Lan- nition, or BLEU score for translation), which makes the guage Modeling, a task central to language un- task of training better LMs valuable by itself. derstanding. We extend current models to deal Further, when trained on vast amounts of data, language with two key challenges present in this task: cor- models compactly extract knowledge encoded in the train- pora and vocabulary sizes, and complex, long ing data. For example, when trained on movie subti- term structure of language. We perform an ex- tles (Serban et al., 2015; Vinyals & Le, 2015), these lan- haustive study on techniques such as character guage models are able to generate basic answers to ques- Convolutional Neural Networks or Long-Short tions about object colors, facts about people, etc. Lastly, Term Memory, on the One Billion Word Bench- recently proposed sequence-to-sequence models employ mark. Our best single model significantly im- conditional language models (Mikolov & Zweig, 2012) as proves state-of-the-art perplexity from 51.3 down their key component to solve diverse tasks like machine to 30.0 (whilst reducing the number of param- translation (Sutskever et al., 2014; Cho et al., 2014; Kalch- eters by a factor of 20), while an ensemble of brenner et al., 2014) or video generation (Srivastava et al., models sets a new record by improving perplex- 2015a). ity from 41.0 down to 23.7. We also release these models for the NLP and ML community to study Deep Learning and Recurrent Neural Networks (RNNs) and improve upon. have fueled language modeling research in the past years as it allowed researchers to explore many tasks for which the strong conditional independence assumptions are unre- 1. Introduction alistic. Despite the fact that simpler models, such as N- grams, only use a short history of previous words to predict Language Modeling (LM) is a task central to Natural the next word, they are still a key component to high qual- Language Processing (NLP) and Language Understanding. ity, low perplexity LMs. Indeed, most recent work on large Models which can accurately place distributions over sen- scale LM has shown that RNNs are great in combination arXiv:1602.02410v2 [cs.CL] 11 Feb 2016 tences not only encode complexities of language such as with N-grams, as they may have different strengths that grammatical structure, but also distill a fair amount of in- complement N-gram models, but worse when considered formation about the knowledge that a corpora may con- in isolation (Mikolov et al., 2011; Mikolov, 2012; Chelba tain. Indeed, models that are able to assign a low probabil- et al., 2013; Williams et al., 2015; Ji et al., 2015a; Shazeer ity to sentences that are grammatically correct but unlikely et al., 2015). may help other tasks in fundamental language understand- ing like question answering, machine translation, or text We believe that, despite much work being devoted to small summarization. data sets like the Penn Tree Bank (PTB) (Marcus et al., 1993), research on larger tasks is very relevant as overfit- LMs have played a key role in traditional NLP tasks such ting is not the main limitation in current language model- as speech recognition (Mikolov et al., 2010; Arisoy et al., ing, but is the main characteristic of the PTB task. Results 2012), machine translation (Schwenk et al., 2012; Vaswani on larger corpora usually show better what matters as many et al.), or text summarization (Rush et al., 2015; Filippova ideas work well on small data sets but fail to improve on et al., 2015). Often (although not always), training better Exploring the Limits of Language Modeling • We show that an ensemble of a number of different models can bring down perplexity on this task to 23.7, a large improvement compared to current state-of-art. • We share the model and recipes in order to help and motivate further research in this area. In Section2 we review important concepts and previous work on language modeling. Section3 presents our contri- butions to the field of neural language modeling, emphasiz- ing large scale recurrent neural network training. Sections 4 and5 aim at exhaustively describing our experience and understanding throughout the project, as well as emplacing our work relative to other known approaches. 2. Related Work Figure 1. A high-level diagram of the models presented in this pa- In this section we describe previous work relevant to the per. (a) is a standard LSTM LM. (b) represents an LM where both approaches discussed in this paper. A more detailed dis- input and Softmax embeddings have been replaced by a character cussion on language modeling research is provided in CNN. In (c) we replace the Softmax by a next character prediction LSTM network. (Mikolov, 2012). 2.1. Language Models larger data sets. Further, given current hardware trends and Language Modeling (LM) has been a central task in NLP. vast amounts of text available on the Web, it is much more The goal of LM is to learn a probability distribution over straightforward to tackle large scale modeling than it used sequences of symbols pertaining to a language. Much work to be. Thus, we hope that our work will help and motivate has been done on both parametric (e.g., log-linear models) researchers to work on traditional LM beyond PTB – for and non-parametric approaches (e.g., count-based LMs). this purpose, we will open-source our models and training Count-based approaches (based on statistics of N-grams) recipes. typically add smoothing which account for unseen (yet pos- We focused on a well known, large scale LM benchmark: sible) sequences, and have been quite successful. To this the One Billion Word Benchmark data set (Chelba et al., extent, Kneser-Ney smoothed 5-gram models (Kneser & 2013). This data set is much larger than PTB (one thou- Ney, 1995) are a fairly strong baseline which, for large sand fold, 800k word vocabulary and 1B words training amounts of training data, have challenged other paramet- data) and far more challenging. Similar to Imagenet (Deng ric approaches based on Neural Networks (Bengio et al., et al., 2009), which helped advance computer vision, we 2006). believe that releasing and working on large data sets and Most of our work is based on Recurrent Neural Networks models with clear benchmarks will help advance Language (RNN) models which retain long term dependencies. To Modeling. this extent, we used the Long-Short Term Memory model The contributions of our work are as follows: (Hochreiter & Schmidhuber, 1997) which uses a gating mechanism (Gers et al., 2000) to ensure proper propaga- • We explored, extended and tried to unify some of the tion of information through many time steps. Much work current research on large scale LM. has been done on small and large scale RNN-based LMs (Mikolov et al., 2010; Mikolov, 2012; Chelba et al., 2013; • Specifically, we designed a Softmax loss which is Zaremba et al., 2014; Williams et al., 2015; Ji et al., 2015a; based on character level CNNs, is efficient to train, Wang & Cho, 2015; Ji et al., 2015b). The architectures that and is as precise as a full Softmax which has orders of we considered in this paper are represented in Figure1. magnitude more parameters. In our work, we train models on the popular One Bil- • Our study yielded significant improvements to the lion Word Benchmark, which can be considered to be a state-of-the-art on a well known, large scale LM task: medium-sized data set for count-based LMs but a very large from 51.3 down to 30.0 perplexity for single models data set for NN-based LMs. This regime is most interesting whilst reducing the number of parameters by a factor to us as we believe learning a very good model of human of 20. language is a complex task which will require large models, Exploring the Limits of Language Modeling T and thus large amounts of data. Further advances in data inner product zw = h ew where h is a context vector and availability and computational resources helped our study. ew is a “word embedding” for w. We argue this leap in scale enabled tremendous advances in The main challenge when jV j is very large (in the order deep learning. A clear example found in computer vision is of one million in this paper) is the fact that computing Imagenet (Deng et al., 2009), which enabled learning com- all inner products between h and all embeddings becomes plex vision models from large amounts of data (Krizhevsky prohibitively slow during training (even when exploiting et al., 2012). matrix-matrix multiplications and modern GPUs). Several A crucial aspect which we discuss in detail in later sections approaches have been proposed to cope with the scaling is- is the size of our models. Despite the large number of pa- sue: importance sampling (Bengio et al., 2003; Bengio & rameters, we try to minimize computation as much as pos- Senecal´ , 2008), Noise Contrastive Estimation (NCE) (Gut- sible by adopting a strategy proposed in (Sak et al., 2014) mann & Hyvarinen¨ , 2010; Mnih & Kavukcuoglu, 2013), of projecting a relatively big recurrent state space down so self normalizing partition functions (Vincent et al., 2015) that the matrices involved remain relatively small, yet the or Hierarchical Softmax (Morin & Bengio, 2005; Mnih & model has large memory capacity.
Details
-
File Typepdf
-
Upload Time-
-
Content LanguagesEnglish
-
Upload UserAnonymous/Not logged-in
-
File Pages11 Page
-
File Size-