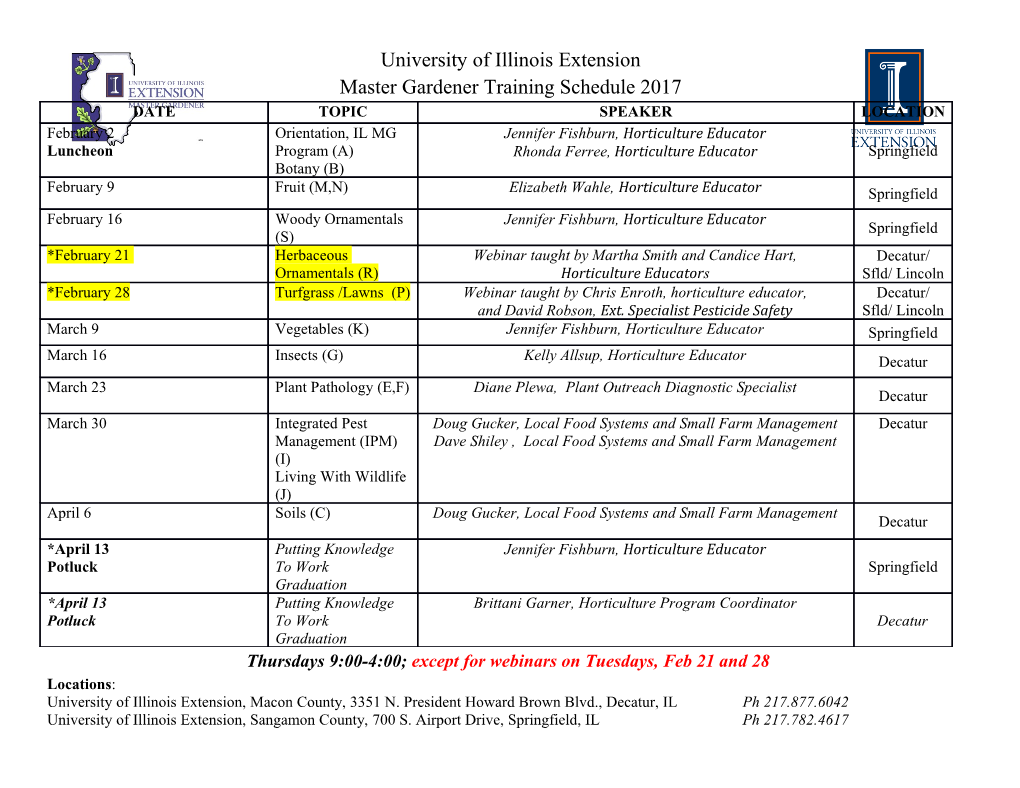
UC San Diego UC San Diego Electronic Theses and Dissertations Title Scaling up psycholinguistics Permalink https://escholarship.org/uc/item/9hh0x7tq Author Smith, Nathaniel J. Publication Date 2011 Peer reviewed|Thesis/dissertation eScholarship.org Powered by the California Digital Library University of California UNIVERSITY OF CALIFORNIA, SAN DIEGO Scaling up psycholinguistics A dissertation submitted in partial satisfaction of the requirements for the degree Doctor of Philosophy in Cognitive Science by Nathaniel J. Smith Committee in charge: Roger Levy, Chair Marta Kutas, Co-Chair Seana Coulson Jeffrey L. Elman Eric Halgren Scott Makeig 2011 Copyright Nathaniel J. Smith, 2011 All rights reserved. The dissertation of Nathaniel J. Smith is approved, and it is acceptable in quality and form for publication on microfilm and electronically: Co-Chair Chair University of California, San Diego 2011 iii TABLE OF CONTENTS Signature Page................................... iii Table of Contents.................................. iv List of Figures.................................... vii List of Tables.................................... ix Acknowledgements.................................x Vita and Publications................................ xi Abstract of the Dissertation............................. xii Chapter 1 Introduction.............................1 References..............................5 Chapter 2 Predictability effects on processing times.............6 2.1 A rational model of cognitive processing times.......8 2.1.1 Deriving the generic predictability effect...... 10 2.1.2 Deriving the form of the predictability effect in lan- guage comprehension................. 15 2.2 Empirical validation...................... 18 2.2.1 Materials and Methods................ 19 2.2.2 Results........................ 20 2.2.3 Discussion...................... 22 2.3 Conclusion.......................... 27 2.4 Acknowledgements...................... 28 2.A Derivation: Monotonicity of processing time......... 28 2.B Derivation: T (r) for logarithmic processing times...... 29 2.C Estimates for low probability words............. 31 2.D Validity of penalized spline regression............ 33 References.............................. 35 Chapter 3 Cloze, corpus, and subjective probabilities............. 40 3.1 Experiment 1......................... 44 3.1.1 Methods........................ 44 3.1.2 Results and Discussion................ 45 3.2 Experiment 2......................... 51 3.2.1 Methods........................ 51 3.2.2 Results and Discussion................ 52 3.2.3 Experiment 3..................... 55 iv 3.2.4 Methods........................ 55 3.2.5 Results and discussion................ 56 3.2.6 Experiment 4..................... 57 3.2.7 Methods........................ 58 3.2.8 Results and Discussion................ 58 3.3 Conclusion and Future Directions.............. 59 3.4 Acknowledgments...................... 62 References.............................. 62 Chapter 4 Pointwise regression for ERP estimation.............. 65 4.1 Least-squares estimation for ERPs.............. 67 4.2 From averaging to regression................. 70 4.2.1 The basic ERP as an intercept term......... 71 4.2.2 Multiple ERPs via dummy coding.......... 74 4.2.3 Difference ERPs via treatment coding........ 75 4.2.4 ‘Slope’ ERPs from numeric predictors........ 78 4.2.5 Putting it all together................. 80 4.3 Non-linear regression..................... 83 4.4 Further considerations.................... 94 4.4.1 Baselining...................... 94 4.4.2 Filtering........................ 94 4.4.3 Significance testing and averaging over windows.. 95 4.4.4 Collinearity: Curse or blessing?........... 97 4.4.5 Which statistic to use? β, R2, r, t, p,...?...... 103 4.5 Discussion........................... 108 4.6 Acknowledgements...................... 110 4.A Derivations for baselining, filtering, windowing....... 110 References.............................. 113 Chapter 5 Continuous-time regression for ERP estimation.......... 118 5.1 One regression for all latencies................ 119 5.1.1 A note on baselining and filtering.......... 121 5.2 Overlap correction...................... 121 5.2.1 Simulation...................... 125 5.2.2 Analyzing a response-related potential........ 128 5.2.3 Comparison to Adjar................. 132 5.3 Generalized Least Squares.................. 133 5.3.1 Motivation...................... 133 5.3.2 Mathematics..................... 136 5.3.3 Finding S ....................... 141 5.3.4 rERPs with less noise................. 149 5.3.5 The linear hypothesis test............... 151 5.3.6 Simulating the linear hypothesis test......... 155 v 5.4 Acknowledgements...................... 157 5.A GLS for orthogonal designs.................. 157 5.B Implementation considerations................ 160 References.............................. 163 Chapter 6 Conclusion............................. 165 vi LIST OF FIGURES Figure 2.1: For any particular computation, there are many possible implemen- tations. ................................9 Figure 2.2: Finding the value of ri which minimizes event i’s unique contribu- tion to the total expected processing cost............... 13 Figure 2.3: As predicted, the relationship between reading time and probability is approximately logarithmic..................... 21 Figure 2.4: To visualize inter-individual variation, we break down the Dundee corpus data by participants and analyze each separately....... 23 Figure 2.5: The same model fits shown in Figure 2.3, but showing the individual effects of the probability of word n on reading time measured at word n and on successive words (the spill-over region)........ 24 Figure 2.6: Here we reproduce Figure 2.3, but extending the x-axis to show the full range of probabilities....................... 31 Figure 2.7: For completeness, we also show the individual word breakdowns across the full range of the data.................... 32 Figure 2.8: The effect of penalization in controlling over-fitting......... 34 Figure 3.1: An informal illustration of the situation faced by those who wish to study linguistic prediction....................... 42 Figure 3.2: Cloze versus corpus probability.................... 47 Figure 3.3: Sample stimulus for Experiment 2................... 53 Figure 4.1: A hypothetical oddball experiment used to illustrate the rERP tech- nique.................................. 72 Figure 4.2: The relationship between xs (predictors), β coefficients (rERPs), and predicted ERPs.......................... 73 Figure 4.3: Likewise, in a model containing a single continuous predictor.... 79 Figure 4.4: Likewise, in a model containing both a categorical and a continuous predictor................................ 82 Figure 4.5: Two sets of possible basis functions for use in fitting non-linear curves to data............................. 87 Figure 4.6: Demonstration of non-linear rERPs applied to analyzing an effect on the latency of an ERP positivity.................. 89 Figure 4.7: For techniques which can detect non-linear effects, we have a trade- off to make. Higher degrees of smoothing produce more stable es- timates; but they may also hide structure present in the data..... 93 Figure 4.8: Average power (µV2) at each electrode from a 500 ms baseline in- terval in the previously mentioned go/no-go task, for each of 7 par- ticipants................................ 104 vii Figure 5.1: Going from one regression model for each latency (previous chap- ter) to a combined regression model for all latencies (this chapter).. 120 Figure 5.2: Simulation 1: Conventional averaged ERPs, and overlap-corrected rERPs, estimated from various amounts of simulated data with SOAs randomly varying in the 200–400 ms range.......... 126 Figure 5.3: Simulation 2: Conventional averaged ERPs, and overlap-corrected rERPs, estimated from various amounts of simulated data, with a fixed SOA of 300 ms and a continuous factor which acts linearly on the ERP................................ 127 Figure 5.4: Simulation 3: Conventional averaged ERPs, and overlap-corrected rERPs, estimated from various amounts of simulated data, with SOAs varying randomly between 200–400 ms and a continuous fac- tor which acts linearly on the ERP................... 129 Figure 5.5: rERP estimates time-locked to stimulus presentation and response, as estimated by our overlap correction technique........... 130 Figure 5.6: Comparison between overlap model and non-linear model at de- scribing go/no-go data......................... 131 Figure 5.7: The autocorrelation and power spectral density function for white versus EEG noise........................... 135 Figure 5.8: The correlation matrix for EEG noise, corrected using Option 1... 144 Figure 5.9: The correlation matrix for EEG noise, corrected using Options 2 and 3.................................. 145 Figure 5.10: Simulation 4: Overlap correction under extreme conditions, using three different estimation techniques................. 150 Figure 5.11: Simulation 5: Distribution of p values in a linear hypothesis test on simulated data, where the null was true................ 156 Figure 5.12: Simulation 6: Distribution of p values in a linear hypothesis test on simulated data, where an effect existed................ 156 viii LIST OF TABLES Table 3.1: Sample continuation distributions from Experiment 1......... 46 Table 3.2: Estimated coefficients from a (modified) log-linear model regressing cloze responses against corpus probability and other measures.... 49 Table 3.3: Mixed-effects
Details
-
File Typepdf
-
Upload Time-
-
Content LanguagesEnglish
-
Upload UserAnonymous/Not logged-in
-
File Pages179 Page
-
File Size-